

What is a Standard Error in Statistics?
In statistics, the sample refers to the data which is gathered for the particular group. For example, the sample may be the data collected about the height of the student in the class. A population is a whole group from which the data has been gathered. There are multiple ways to define a population and we should be through about the definition of population.
In order to know the way a sample is denoting the population, we are required to measure the standard error for the particular measurement. The standard error is an important statistical measure and it is related to the standard deviation. It tells the way sample means determine the true population means. A large standard error indicates that there are various changes in the population. A small standard error implies that the population is in a uniform shape.
(image will be uploaded soon)
As we know standard error is quite similar to the standard deviation as both measures the amount of data is spread. The larger the number, the more the data is spread. Although both the standard deviation and standard error are similar, there is one important difference between them. The standard error makes use of sample data whereas standard deviation makes use of population data.
In this article, we will discuss what is the standard error in statistics, standard error equation, standard error of estimate formula, what is the standard error of mean, standard error of mean formula etc.
Standard Error Equation
The precision of a sample that represents a population is identified through standard error equations. The sample mean which is evacuated from the given population is given as:
\[SE_{s} = \frac{s}{\sqrt{n}}\]
In the above standard error equation, S denotes the standard deviation and N denotes the number of observations.
Standard Error of Estimate
The standard error of estimate is the evaluation of the accuracy of any estimation made with the regression line. It is represented as SEE.
The standard error of estimate formula is given by,
In the above standard error of the estimate formula ,Y denotes the individual data set, Y’ denotes the mean of the data and N is the sample size.
\[S_{est} = \sqrt{\frac{\sum (Y - Y')^{2}}{N - 2}}\]
The standard error of the estimate formula is similar to the standard deviation of the mean, the only difference in this formula is that there is N-2 in the denominator rather than N-1 which is in the case of sample standard deviation.
What is the Standard Error of Mean?
Standard error mean also known as the standard deviation of mean is a method used to estimate the standard deviation of the sampling distribution. It is also written as SEM. For example, let us perform an experiment that measures the speed of sound in the material that passes through three different directions (i.e. passing through x, y, and z coordinates). If we calculate the mean of these values, we will get an average speed of sound in this medium.
However, there are many external factors that can influence the speed of the sound that passes through different directions such as temperature changes, velocity changes and other random variations. Hence, instead of measuring the mean with one measurement. We should consider several measurements and calculate the value of mean each time. This is known as the sampling distribution. The standard error of the mean will be different with different experiments performed each time.
The standard error of the mean describes the way mean changes with different experiments measuring a similar quantity. Hence, the standard error of the mean will be larger, if the effects of the random changes are higher and the standard error of the mean will be zero if there are no random charges as the experiments are repeating.
The standard error of the mean formula is given as:
\[\sigma_{M} = \frac{\sigma}{\sqrt{N}}\]
In the above standard error of mean formula, σM is the standard error of the mean, σ is the standard deviation of the original distribution and N is the sample size .The standard error of the mean formula does not assume a normal distribution. However, in many cases the formula does assume a normal distribution. The formula states that larger the sample size, the smaller the standard error of the mean.
It is because if the mean is calculated at each step using many data points, then a small change in one value will cause less effect on the final mean. Hence, we can say that the size of the standard error of the mean is inversely proportional to the square root of the sample size.
Solved Examples
1. Find the standard error of the given data set
10,12,16,21,and 25
Solution:
Mean = Total number of observation/Number of observation
Mean = 10 + 12 + 16 + 21+ 25/5
Mean = 16.8
Standard deviation of the above data is calculated using the below formula
\[\sqrt{\frac{\sum |x - \mu|^{2}}{N}}\]
\[\sqrt{(\frac{1}{5} - 1) \times (10 - 16.8)^{2} + ( 12 - 16.8)^{2} + (16 - 16.8)^{2} + (21 - 16.8)^{2} + ( 25 - 16.8)^{2}}\]
= \[\sqrt{\frac{(154.8)}{4}}\]
= \[\sqrt{38.7}\]
= 6.22
Standard Error
\[SE_{s} = \frac{s}{\sqrt{n}}\]
= \[\frac{6.22}{\sqrt{5}}\]
= \[\frac{6.22}{2.236}\]
= 2.782
2. Calculate the standard error of mean of the following observation
10,20,30,40,50.
Solution :
To calculate the standard error of the mean of the above data, we should know both the mean value and standard deviation value.
Let's calculate the mean value of above data
Mean = Total number of observation/Number of observation
X = 10,20,30,40,50
Total number of observation (n) = 5
Mean = ( 10 + 20 + 30 + 40 + 50)/5 = 150/5 = 3
Hence, mean value = 30
The standard deviation of the above data is calculated using the below formula
\[\sqrt{\frac{\sum |x - \mu|^{2}}{N}}\]
= \[\sqrt{(\frac{1}{5 - 1}) \times (10 - 30)^{2} + (20 - 30)^{2} + (30 - 30)^{2} + (40 - 30)^{2} + (50 - 30)^{2}}\]
= \[\sqrt{\frac{1}{4} (400 + 100 + 0 + 100 + 400}\]
= \[\sqrt{250}\]
= 15.811
Standard Error
\[SEM = \frac{SD}{\sqrt{N}}\]
= \[\frac{15.811}{\sqrt{5}}\]
= \[\frac{15.8114}{2.2361}\]
= 7.0711
Quiz Time
1. The standard deviation of the sampling distribution is also known as
Standard error
Statistical error
Sampling error
Probability error
2. The formula for the standard error of the mean
Standard deviation/square root of n
Standard deviation/ n
Standard deviation/Mean
Mean/Standard deviation
FAQs on Standard Error Formula
1. What is the Meaning of Standard Error of Measurement?
The standard error of measurement is used to determine the effect of specific results in a test. Standard deviation is a function of both the reliability of the test and standard deviation of observed scores. When the test is accurately reliable, the value of the standard error of measurement will be equal to 0. When the test is comprehensively unreliable, the standard error of measurement reaches its maximum, equivalent to the standard deviation of the observed scores.
The main benefit of the standard error of measurement is that it is the original unit of measurement. With the deviation of the extreme distribution, standard error of measurement is examined as a fixed characteristic of a specified test or measure.
2. What is Meant by a Standard Deviation?
Standard deviation is a measurement of dispersion in statistics. It gives an idea about the amount of data in a given data set that is dispersed from the mean. The definition of the standard deviation states that it is the square root of the mean of the square of the deviation of all the values of a series derived from the arithmetic mean. Standard deviation is also known as root mean square deviation. The symbol used to represent standard deviation is sigma (σ).
The formula to calculate standard deviation is
√(Σ|x - μ|²/N)
In the above formula ∑ represents “sum off” , x indicates the value in a data set, μ represents the mean of data and N represents the total number of data points in the population.
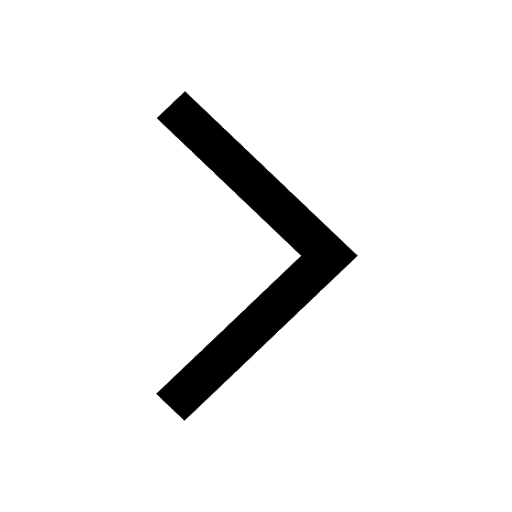
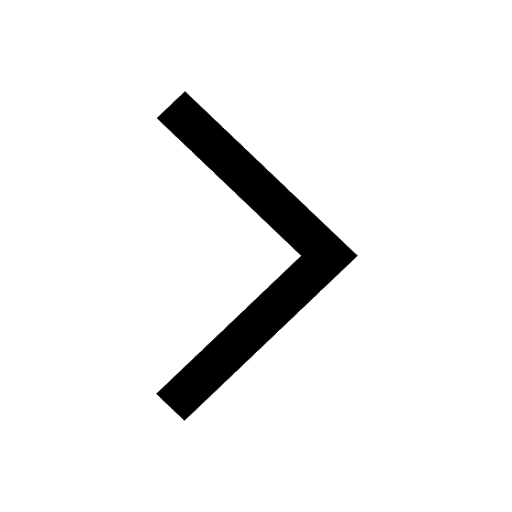
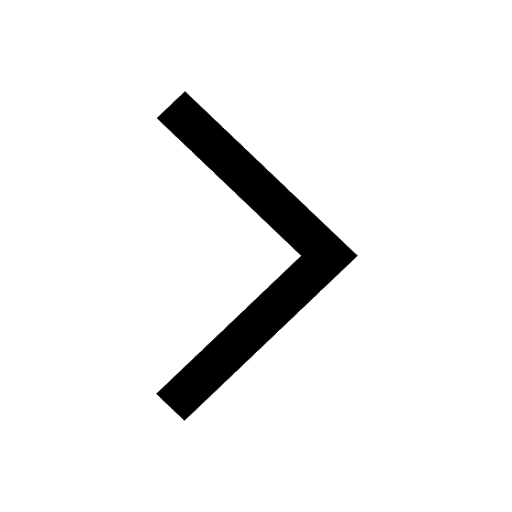
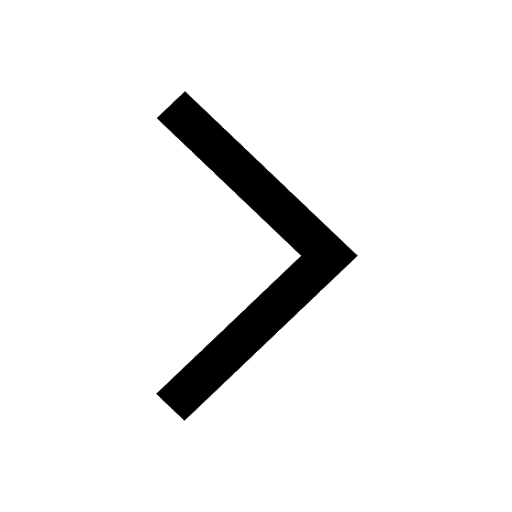
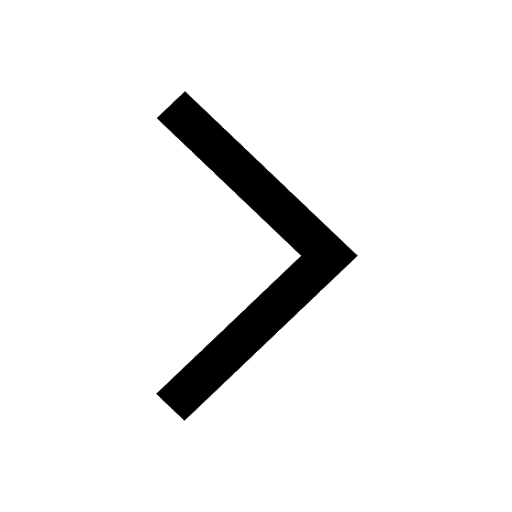
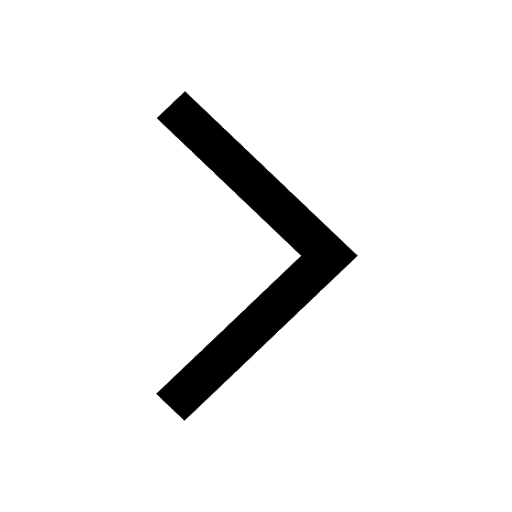
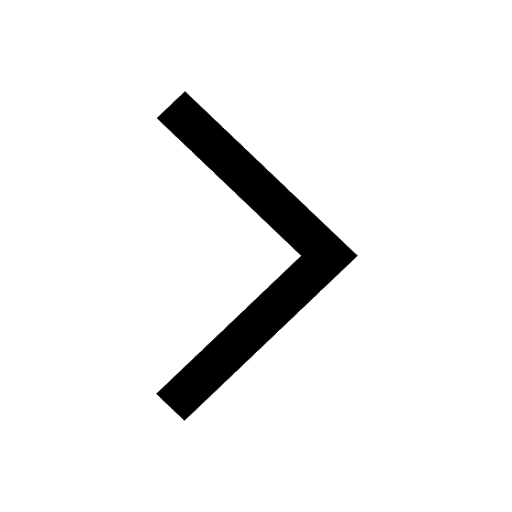
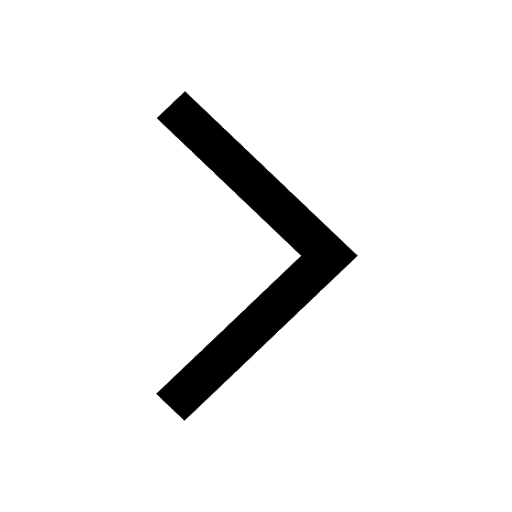
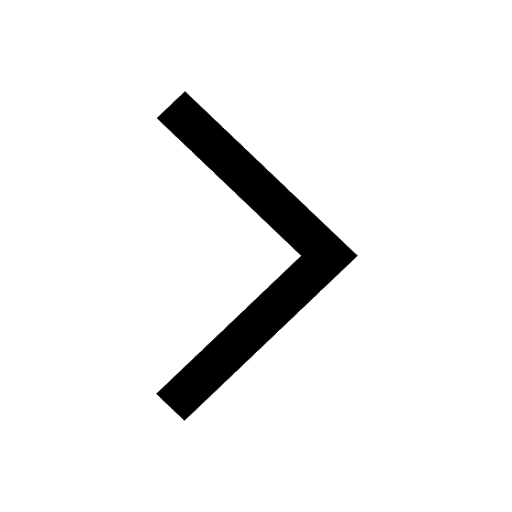
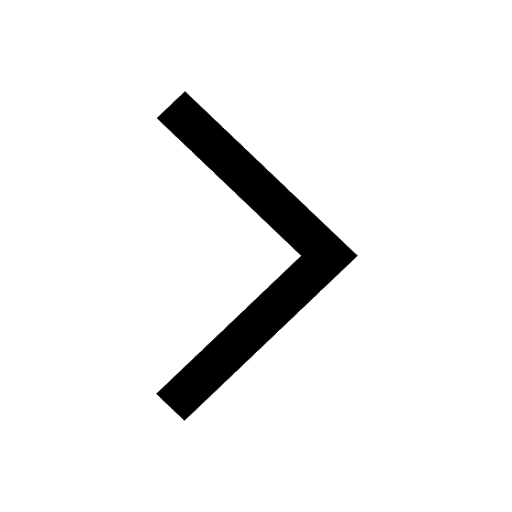
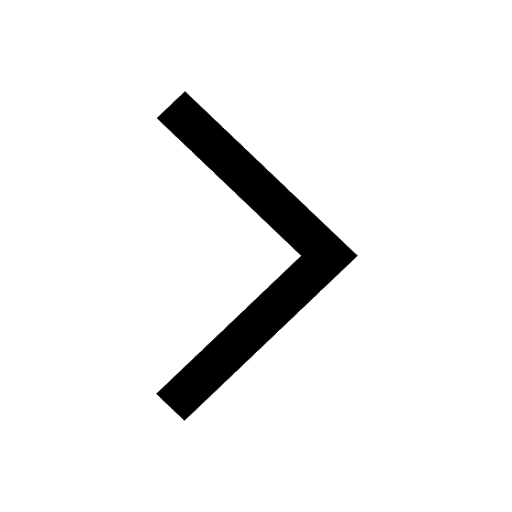
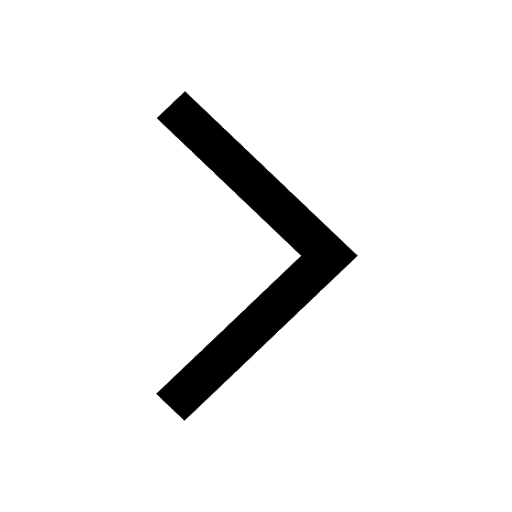
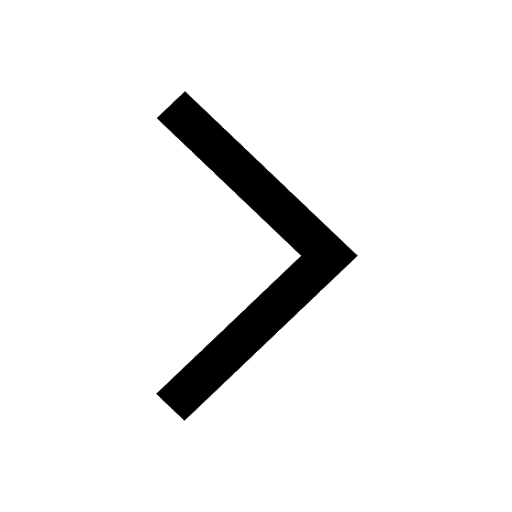
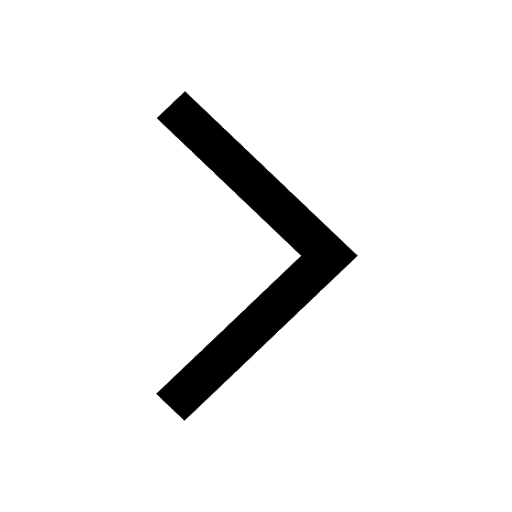
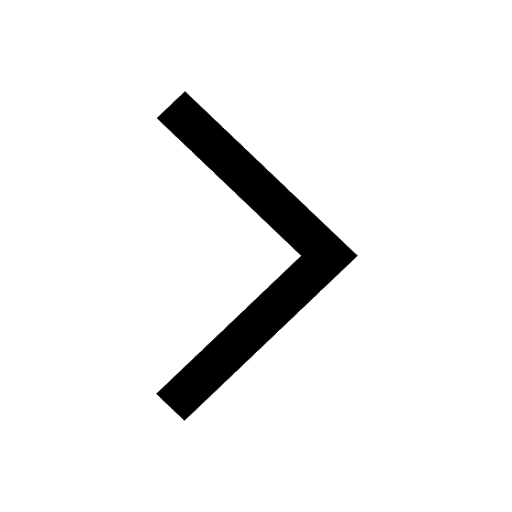
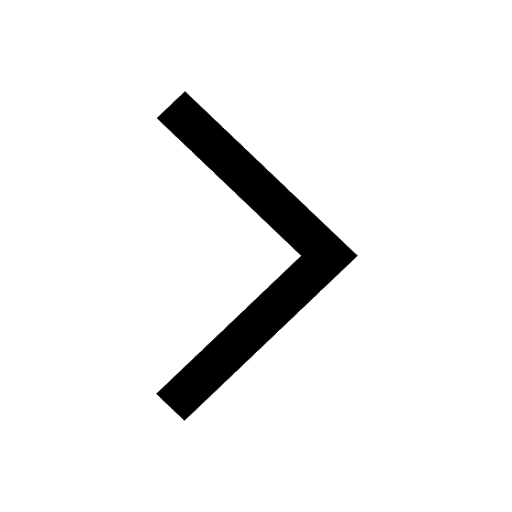
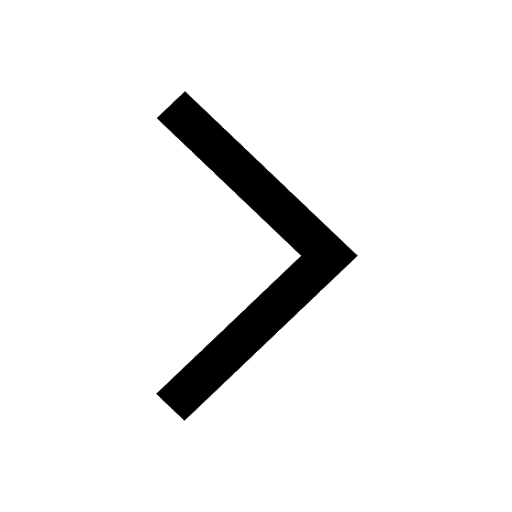
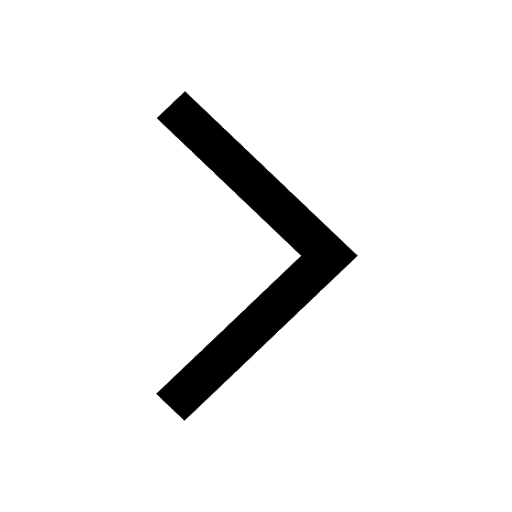