What is Correlation and Covariance: Introduction
FAQs on Difference Between Correlation and Covariance Characteristics for JEE Main 2024
1. How are correlation and covariance related?
Correlation and covariance are related statistical measures that describe the relationship between two variables. Covariance measures the joint variability between the variables, indicating whether they tend to vary together or in opposite directions. Correlation, on the other hand, standardizes the covariance by dividing it by the product of the standard deviations of the variables. This normalization produces a correlation coefficient that ranges from -1 to +1, representing the strength and direction of the linear relationship.
2. What is the range of correlation?
The range of correlation is from -1 to +1. The correlation coefficient represents the strength and direction of the linear relationship between two variables. A correlation coefficient of +1 indicates a perfect positive correlation, meaning that as one variable increases, the other variable increases proportionally. Conversely, a correlation coefficient of -1 indicates a perfect negative correlation, where one variable increases as the other decreases. A correlation coefficient of 0 signifies no linear relationship between the variables.
3. Is correlation affected by the scale of measurement?
No, correlation is not affected by the scale of measurement of the variables. Correlation is a unitless measure that assesses the linear relationship between variables. It is based on the calculation of covariances and the standard deviations of the variables. Since the calculations involve dividing covariances by the product of standard deviations, the scale or units of measurement cancel out. This allows for comparisons of correlation coefficients across different studies or datasets, regardless of the specific scale used for the variables.
4. What are some applications of correlation and covariance?
Correlation and covariance find applications in various fields. In finance, they help analyze the relationships between different assets and portfolios. In economics, they aid in understanding the interactions between economic variables. In social sciences, they assist in studying relationships between variables like income and education. In scientific research, they help determine associations between variables in experiments. In data analysis, they provide insights into data patterns and dependencies. Additionally, they are used in predictive modeling, risk assessment, portfolio optimization, and quality control.
5. How can correlation and covariance be interpreted?
The interpretation of correlation and covariance requires careful consideration. Correlation indicates the strength and direction of the linear relationship between variables, while covariance measures the joint variability. A correlation coefficient close to +1 or -1 suggests a strong relationship, while a coefficient close to 0 indicates a weak or no linear relationship. Positive covariance implies that variables tend to change together, while negative covariance suggests they change in opposite directions.
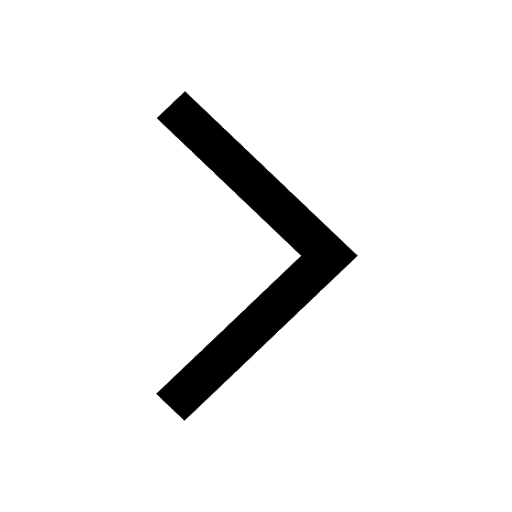
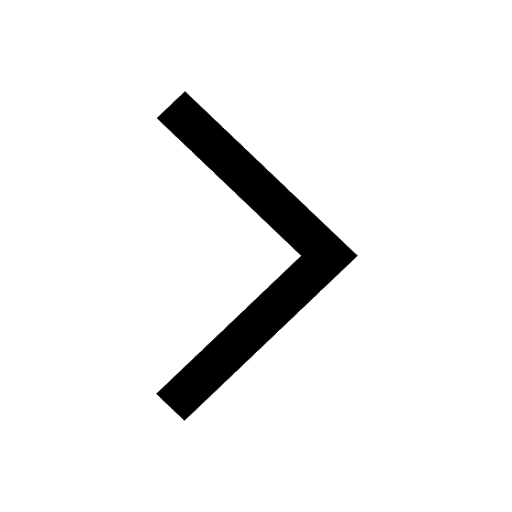
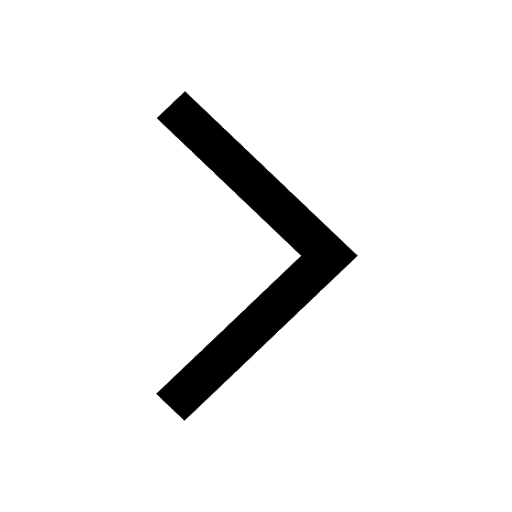
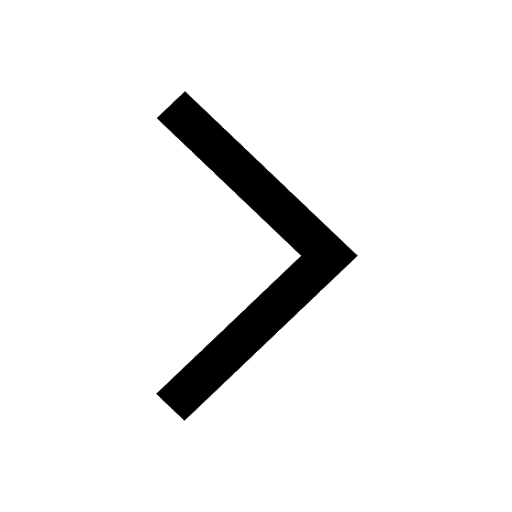
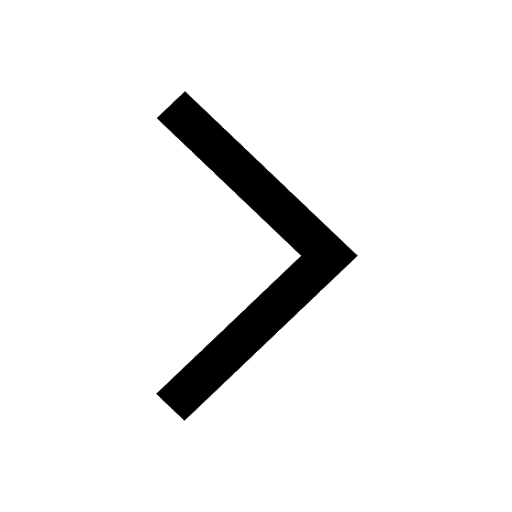
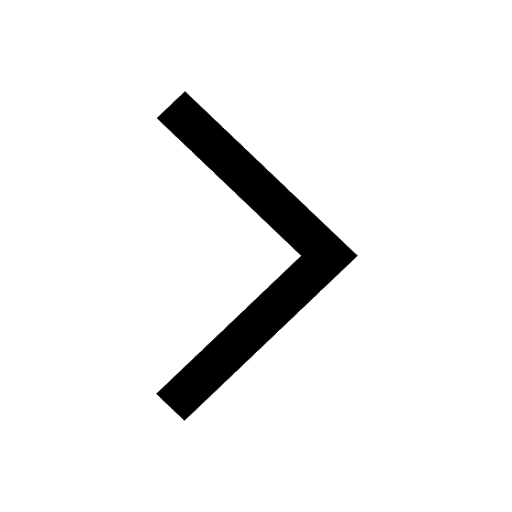
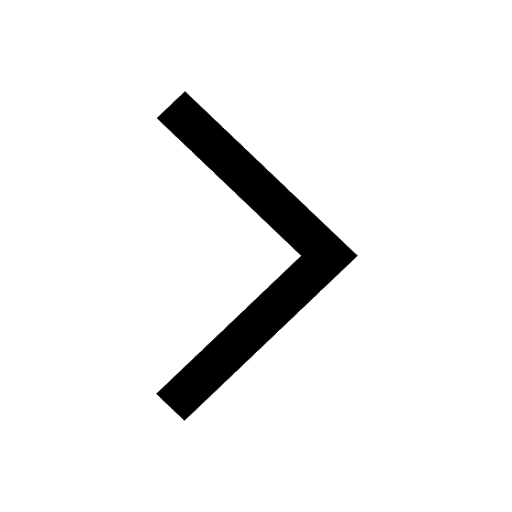
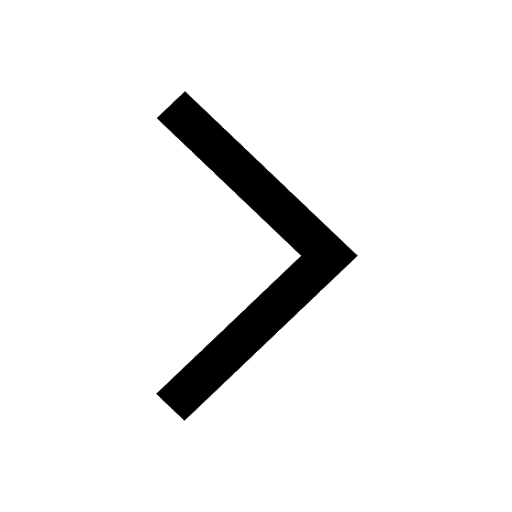
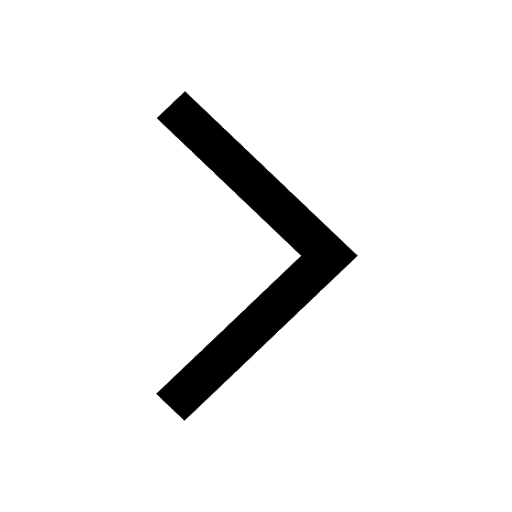
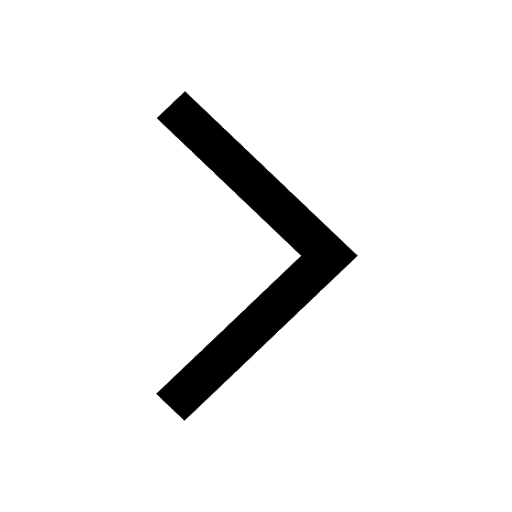
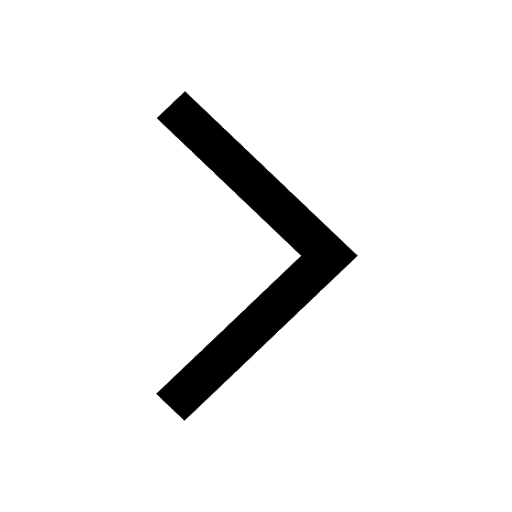
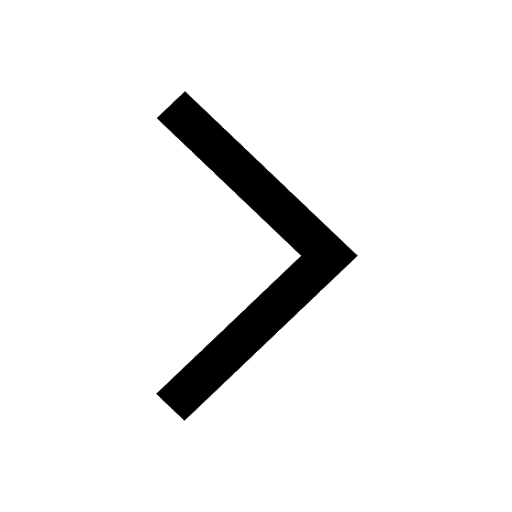