

Central Limit Theorem for Proportions
If we talk about the central limit theorem meaning, it means that the mean value of all the samples of a given population is the same as the mean of the population in approximate measures, if the sample size of the population is fairly large and has a finite variation. The central limit theorem is one of the important topics when it comes to statistics. In this article, we will be learning about the central limit theorem standard deviation, the central limit theorem probability, its definition, formula, and examples.
Central Limit Theorem Definition
Let us first define the central limit theorem.
The Central Limit Theorem states that the overall distribution of a given sample mean is approximately the same as the normal distribution when the sample size gets bigger and we assume that all the samples are similar to each other, irrespective of the shape of the total population distribution.
Central Limit Theorem Statistics Example
To understand the Central Limit Theorem better, let us consider the following example.
(Image to be added soon)
Assume that you have 10 different sports teams in your school and each team consists of 100 students. Now, we need to find out the average height of all these students across all the teams. How will we do it when there are so many teams and so many students?
Well, the easiest way in which we can find the average height of all students is by determining the average of all their heights. To do so, we will first need to determine the height of each student and then add them all. Then, we will need to divide the total sum of the heights by the total number of the students and we will get the average height of the students. Well, this method to determine the average is too tedious and involves tiresome calculations. So, how do we calculate the average height of the students? We can do so by using the Central Limit Theorem for making the calculations easy.
(Image to be added soon)
In this method of calculating the average, we will first pick the students randomly from different teams and determine a sample. Every sample would consist of 20 students. Then, we would follow the steps mentioned below:
First, we will take all the samples and determine the mean of each sample individually.
Then, we will determine the mean of these sample means.
This way, we can get the approximate mean height of all the students who are a part of the sports teams.
If we find the histogram of all these sample mean heights, we will obtain a bell-shaped curve.
(Image to be added soon)
Note: It is important to remember that the samples that are taken should be enough by size. When we take a larger sample size, the sample mean distribution becomes normal when we calculate it by repeated sampling.
Central Limit Theorem Formula
Now that we learned how to explain the central limit theorem and saw the example, let us take a look at what is the formula of the Central Limit Theorem.
We can apply the Central Limit Theorem for larger sample size, i.e., when n ≥ 30.
The formula of the Central Limit Theorem is given below.
μx = μ
𝜎x= 𝜎/√n
Here,
μ is the population mean
𝜎 is the standard deviation of the population
μx is the sample mean
𝜎x is the sample standard deviation
n is the sample size
FAQs on Central Limit Theorem
1. State the central limit theorem.
The Central Limit Theorem or CLT, according to the probability theory, states that the distribution of all the samples is approximately equal to the normal distribution when the sample size gets larger, it is assumed that the samples taken are all similar in size, irrespective of the shape of the population distribution.
To explain it in simpler words, the Central Limit Theorem is a statistical theory which states that when a sufficiently larger sample size of a population is given that has a finite level of variance, the mean value of all the given samples from the same given population is approximately equal to the population mean. Also, all the samples would tend to follow an approximately normal distribution pattern, when all the variances will be approximately equal to the variance of the entire population when it is divided by the size of the sample.
2. What are the applications of the central theorem in statistics?
The different applications of the Central Theorem in the field of statistics are as follows.
If the distribution is not normal or is unknown, we take into consideration that the sample distribution is normal according to the Central Limit Theorem. This method tends to assume that the given population is distributed normally. This, in turn, helps us to analyze the data in methods such as building the confidence intervals.
For estimating the mean of the population more accurately, we tend to increase the samples that are taken from the population that would ultimately decrease the mean deviation of the samples.
For creating the range of different values that are likely to have the population mean, we can make use of the sample mean.
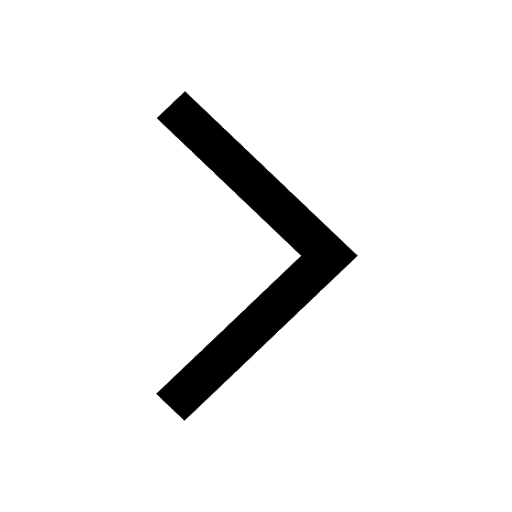
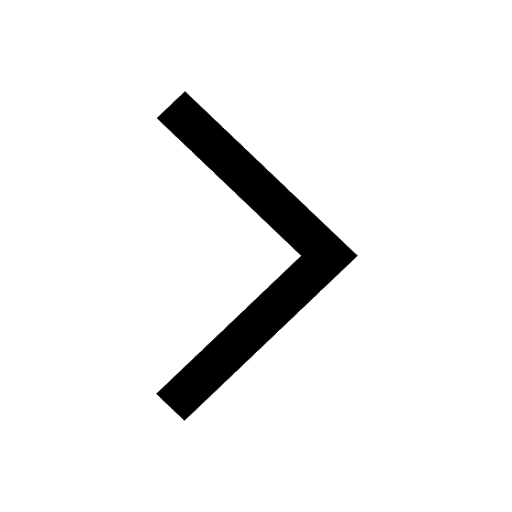
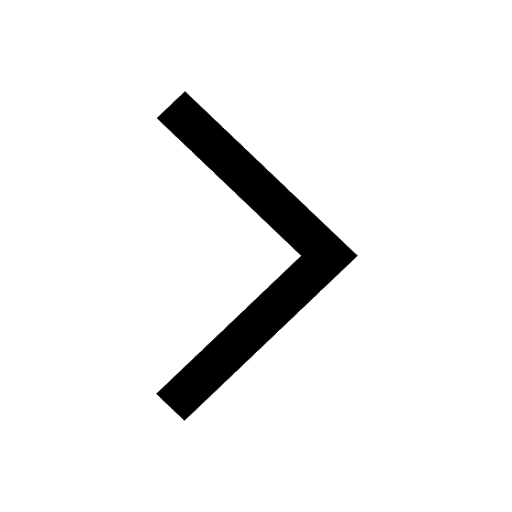
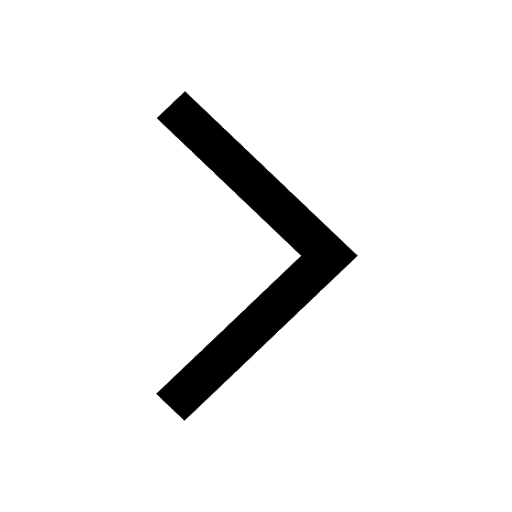
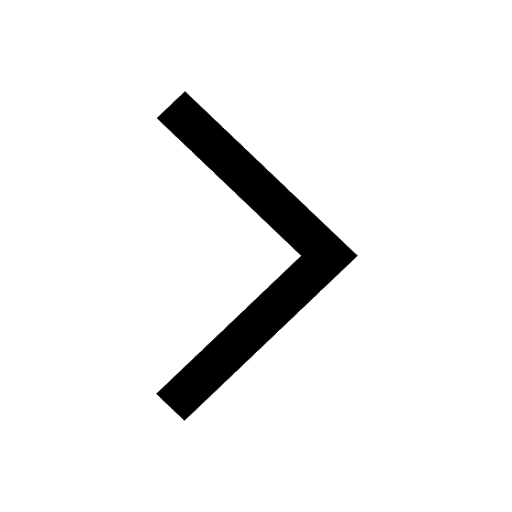
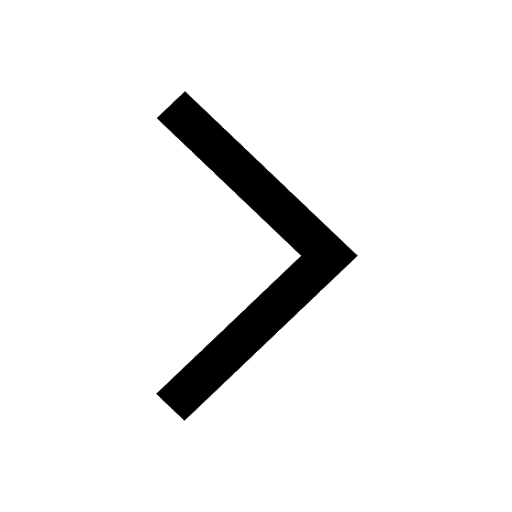
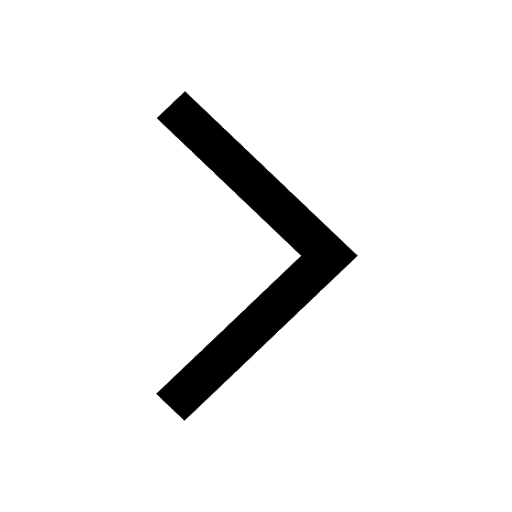
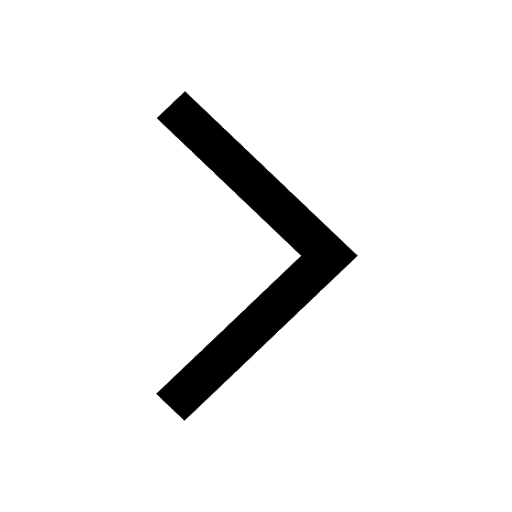
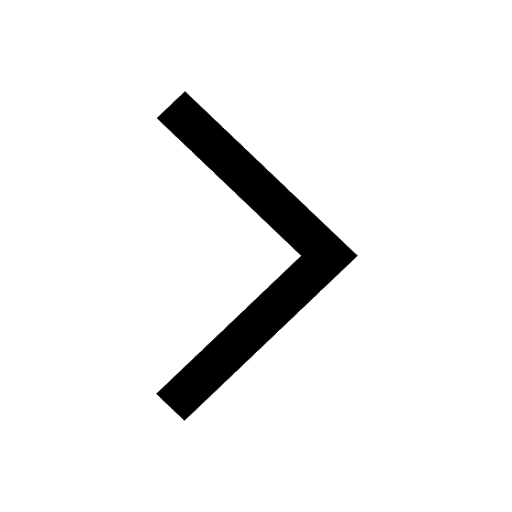
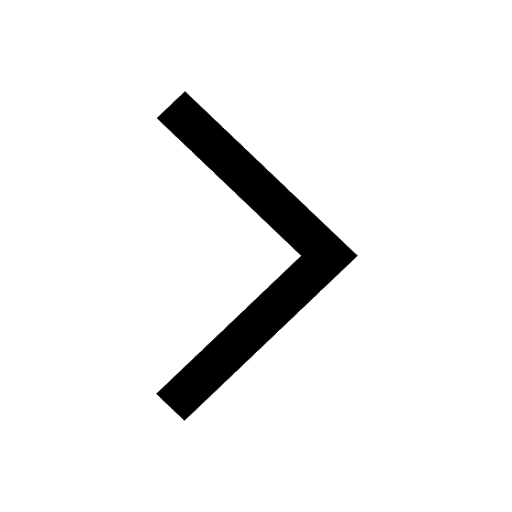
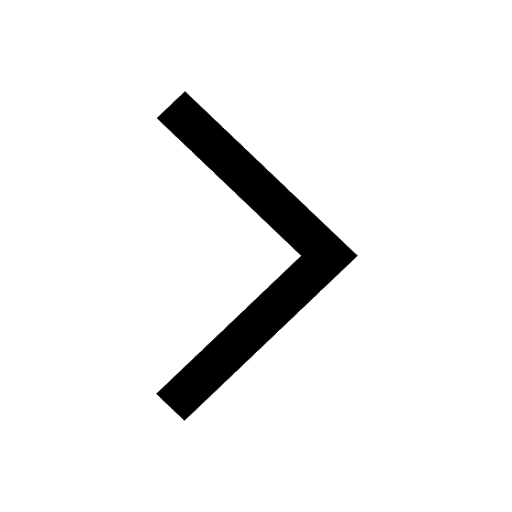
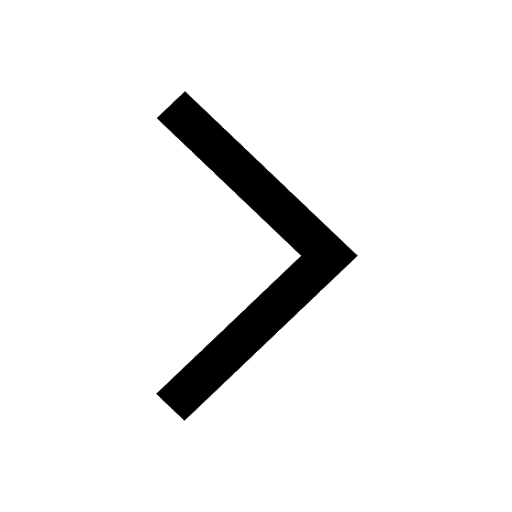
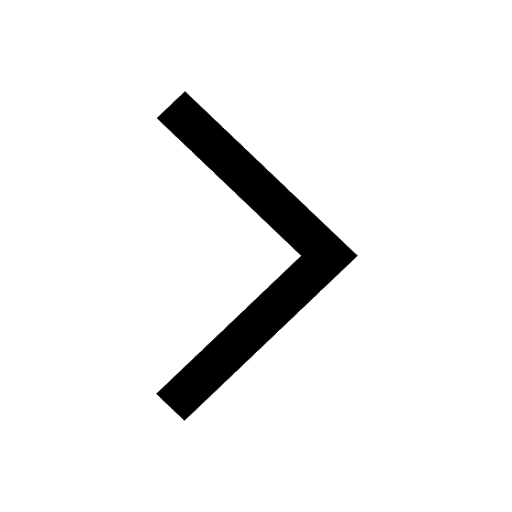
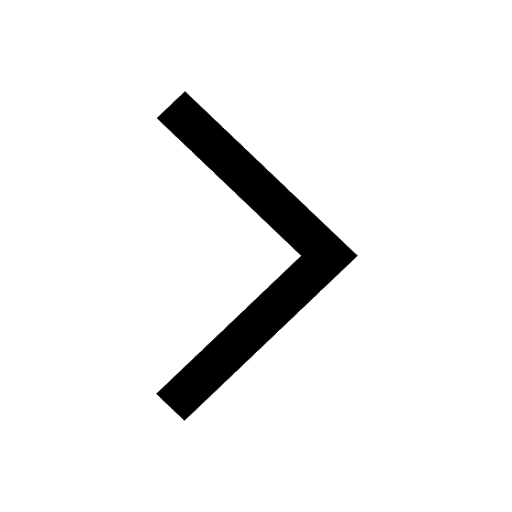
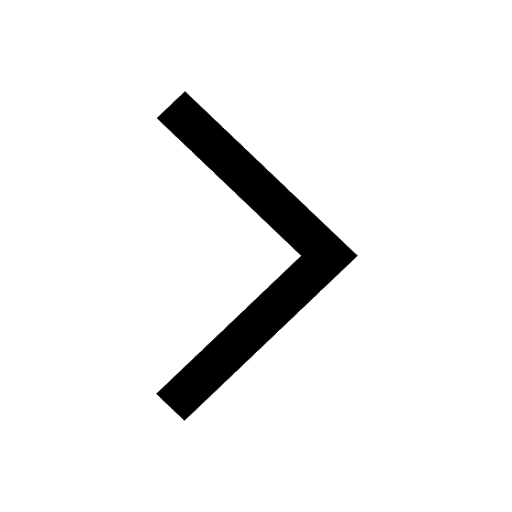
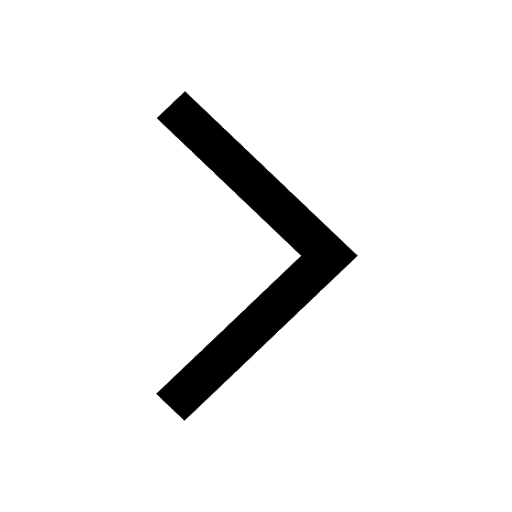
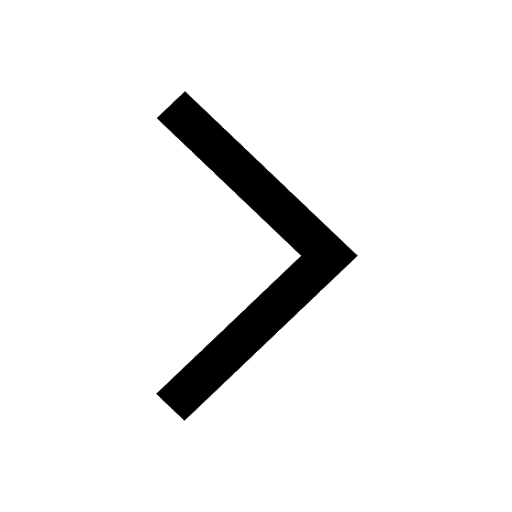
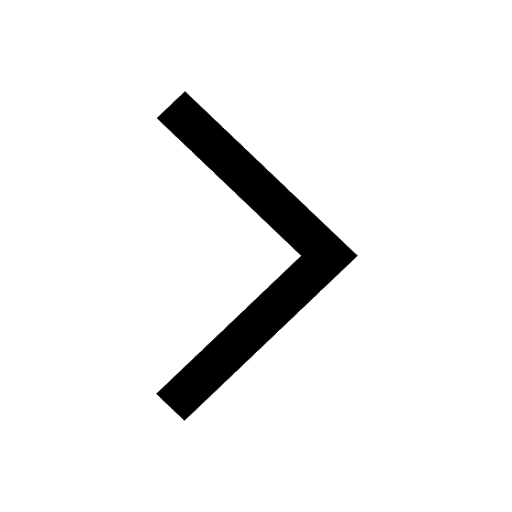