

Explanation and Formula of Conditional Probability
Conditional probability is described as the possibility of occurrence of an event or outcome, based on the eventuality of a preceding event or outcome. The concept of conditional probability function is one of the ideal theories of probability mathematics. It is chiefly associated with Bayes’ theorem, which is again one of the most dominant concepts in statistics. You have understood the concept of conditional probability. Now quickly let’s take you how Conditional probability is calculated. To calculate Conditional probability— multiply the probability of the previous event by the new or updated probability of the subsequent, or conditional, event.
(Images will be Uploaded soon)
Conditional Probability Formula
You might not know but the formula for conditional probability is extracted from the probability multiplication rule. P {A and B} = P{A}*P {B|A}. You may also observe this law in the form P (A∪B). Where, the Union symbol (∪) denotes “and”, in the sense that event A happening and event B is happening.
P {B|A} = P {A and B} / P {A}
Thus, you can write your conditional probability formula as image shown below:
(Images will be Uploaded soon)
Conditional Probability Example and Solution
To take an event as an example, let’s solve the conditional probability like already a champion. Say that,
ü Event A is that it is shining bright outside after two days of consecutive rain, and it has a 0.4(40%) likeliness of sunlight today.
ü Event B is that you will require stepping outside and that has a chance of 0.6 (60%)
Thus, use the formula and the conditional probability is
P {A|B} = {0.4*0.6}/0.4 = 60%
A conditional probability would take into consideration these two events in relationship with one another, such as the probability that it is both sun shining and you will have to step outside home.
Conditional Probability vs. Joint Probability
Conditional Probability: p (A|B) is the probability of event or outcome ‘A’ happening, provided that event ‘B’ has already happened. Example: provided that from a deck of 52 playing cards, you drew a black card, what’s the probability that it’s a six (p {six|red} )=2/26=1/13. Therefore, out of the 26 black cards (given a black card), there are two six’s thus, 2/26=1/13.
Joint Probability: p (A and B). The probability of event or outcome ‘A’ and event ‘B’ happening. It is actually the probability or likeliness of the bisection of two or more events. The probability of the bisection of A and B may be mathematically expressed as p (A ∩ B). Example: the probability that a card is a six and black =p (six and black) = 2/52=1/26. (There are two black six cards in a deck of 52 playing cards, the 4 of spades and the 4 of suits).
(Images will be Uploaded soon)
Solved Examples
Problem1:
A piggy bank contains 3 coins. Of which 2 are regular coins and 1 is a fake-headed coin. {P (H) = 1 P (H)=1}.
A. You randomly picked a coin and tossed it. What is the probability that it will fetch up heads?
Solution:
Given the assumption,
Let’s assume A1A1 be the condition that you select a regular coin, and A2A2 be the condition that you select the 2-headed coin. Keep in mind that A1A1 and A2A2 develop a sample space partition.
Now,
P {H|A1) = 0.5, P {H|A1} = 0.5,
P {H|A2} =1.P {H|A2} =1
Here, applying the principle of probability, we write
P {H} P {H} = P {H|A1} P {A1} + P {H|A2} P {A2} - P {H|A1} P {A1} + P {H|A2} {A2}
1/2.2/3 + 1.1 /3 = 2/3
Hence, you get the probability = 2/3.
Problem2:
A piggy bank contains 3 coins. Of which 2 are regular coins and 1 is a fake-headed coin. {P (H) = 1 P (H) =1}.
B. You randomly picked a coin and tossed it, and you got heads. What is the probability that the coin will be a two-headed coin?
Solution:
In order to find out P {A2|H} P {A2|H}
Applying the Bayes’ rule
P {A2|H} P {A2|H} =P {H|A2} P {A2} + P {H} = P {H|A2} P{A2} / P {H}
1/1.3/ 2/3 = ½
Thus, the probability is ½.
Did You Know
Conditional probability does not indicate that there is a causal relationship between the two events all the time
Conditional probability does not state that both take place at the same time
Conditional probability can set opposition against unconditional probability.
FAQs on Conditional Probability
1. What is Bayes' Theorem?
Named after the renowned mathematician of the 18th-century—Thomas Bayes is the Bayes' theorem aka Bayes' Law or Bayes' Rule. It is actually a mathematical formula that is used to identify conditional probability. This set of rules of probability aids to update the predictions of events while making for better and more dynamic estimates.
In typical terms, Bayes’ theorem provides an easy and influential strategy to revise existing anticipations or theories (update probabilities) based on new or additional proofs that have been received. Bayes’ theorem is not only restricted to mathematics but is also used in different fields like statistics, machine learning, finance, etc. For example, in finance, it can be utilized to assess and rate the risk of lending money to borrowers.
2. Can Conditional Probability be Used in Real Life?
It’s been many years since the Conditional probability is in use. Conditional probability is being actively used in diverse fields such as insurance, calculus, and politics. A typical conditional probability real-life example would be, the re-election of a ruling political party depends upon the voting preference of voters and perhaps a successful marketing campaign—even the probability of the opponent party making blunders during press!
The weather forecast team might announce that your area has a probability of getting a cyclone of 60%. Still so, this fact is conditional on various factors, such as the probability of…
A large system of sufficient warm, moist winds over the ocean rises from near the surface.
Rise of the warm wind in your area
High humidity
An area of lower air pressure below
We can state that the conditional probability for a cyclone to form depends on all the above events.
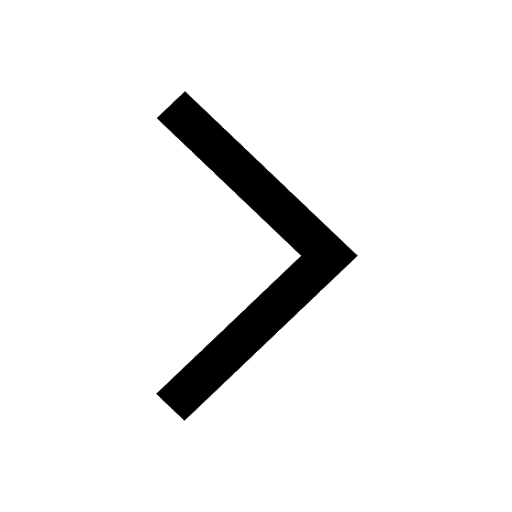
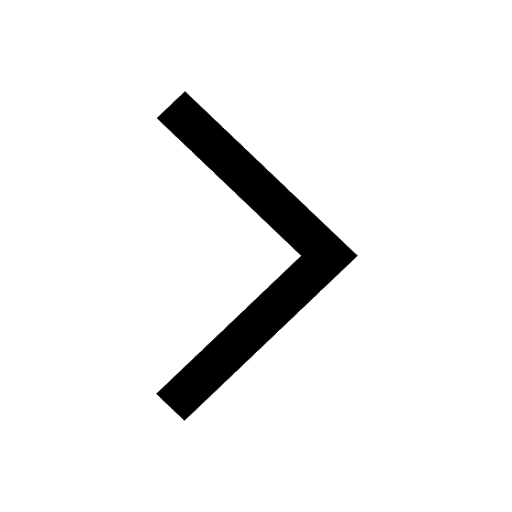
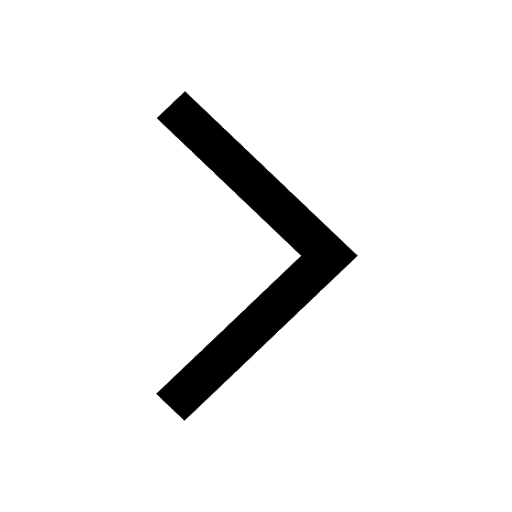
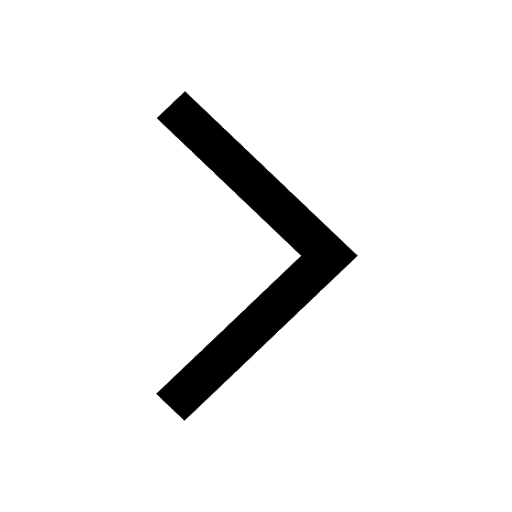
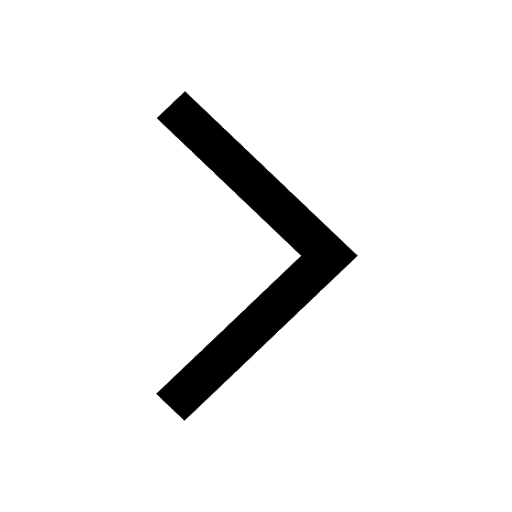
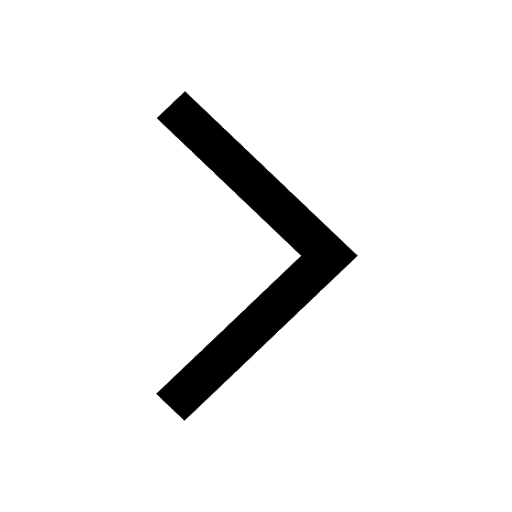