

Covariance Meaning
Statistics is an essential branch of Mathematics which is used in various segments. Therefore, it is imperative that students develop an understanding of the concept of statistics and covariance as it will help them to not only fetch more marks in the final exam but will also assist them in the future. What is covariance in mathematical terms? Let’s find out by analysing covariance definition. Covariance meaning is the computation of the relationship between any two random variables from their mean. It measures the total variation of any two random variables obtained from their expected values.
Types of covariance:
There are two types of covariance depending upon the movement of the two variables. The two types of covariance are:
Positive Covariance:
The covariance is said to be positive when both the variables move in the same direction. When both the variables increase or when both the variables decrease, it is called positive covariance.
Negative Covariance:
The covariance is said to be negative when both the variables move in opposite directions i.e. when both the variables are inversely related. In negative covariance, if X variable increases, Y variable decreases, and vice versa.
Covariance formula:
The covariance formula used to calculate the relationship between two variables is given below:
To understand the formula, consider X and Y as two random variables to find out the covariance properties of these two variables.
Cov (X, Y) = \[\frac{\sum (X_{i} - \overline{X})(Y_{j} - \overline{Y})}{n}\]
Where,
X, Y are random variables.
Xi = data value of x
Yi = data value of y
x̄ = mean of x
ȳ = mean of y
N = number of data values.
The sample covariance formula is:
Cov (x, y) = \[\frac{\sum_{i=1}^{n} (x_{i} - \overline{x})(y_{i} - \overline{y})}{n-1}\]
What is Covariance? Explained with Covariance Example!
Consider the following covariance example to understand the covariance meaning.
Calculate the average value of X and Y variables.
X = \[\frac{2+3+2.7+3.2+4.1}{5}\] = 3.
Y = 10+14+12+15+20 = 14.2.
Substitute the values in the Covariance formula to get the results.
Cov(X,Y) = \[\frac{(2 - 3)(10 - 14.2) + (3 - 3)(14 - 14.2) + (2.7 - 3)(12 - 14.2) + (3.2 - 3)(15 - 14.2) + (4.1 - 3)(20 - 14.2)}{(5-1)}\] = 2.85.
The covariance we obtained from the above example is positive. This is the mathematical expression that answers the question of “What is covariance?”
Covariance Correlation Equation:
Correlation:
Correlation gives the measure of the degree of the linear relationship between two variables. Also, Covariance that tells whether the covariance is positive or negative, the correlation value tells the extent to which the variables move together. Correlation identifies the degree of increase or decrease in the variable.
Correlation Coefficient:
It ranges between -1 and +1. The +1 correlation coefficient value indicates a perfect positive relationship between the two variables. It means that higher scores of X are associated with higher scores of Y. The -1 correlation coefficient value indicates a perfect negative relationship between the two variables. It means that higher scores of X are associated with lower scores of Y.
The Covariance Correlation Formula is:
\[\rho_{X,Y} = \frac{cov(X,Y)}{\sigma_{X} \sigma_{Y}}\]
This is where,
“Cov” represents the covariance value
Sigma X refers to the value obtained for the standard deviation of X.
Sigma Y represents the value obtained for the standard deviation of Y.
What are the Applications of Covariance?
Covariance has wide applications in statistics. Covariance applies to many biological, genomic, financial, and meteorological concepts. It finds its use in micrometeorology and feature extraction.
In genetic and microbiological studies, covariance and correlation equations are the foundation for the measurement of the Genetic relationship matrix. The covariance equation also provides the basis to understand the key concepts of evolution, gene transmission, and natural selection.
Covariance in finance is considered significant as it helps in determining risks and opportunities. It allows the investors to identify whether the instruments are moving in positive covariance or negative covariance. It helps them in preventing losses.
The equation is used for data embedding or dimensionality reduction studies. Its properties are utilized to perform various operations and functions.
The other common application of the formula is canonical correlation analysis (CCA). With this, one understands how and where the sets of variables are moving.
The properties are used in enabling multi-viewing learning.
There are many more applications of covariance and are considered as the basis for many statistical studies.
What is the Inverse Covariance Matrix? What is its Statistical Meaning?
The inverse covariance matrix is an inverse of the covariance matrix. An inverse covariance matrix is referred to as a precision matrix and provides information about the partial correlation of the two different variables. It estimates the tightness of the clustered variables. It estimates the tightness of the variables around the mean of the variables and the degree to which they do not co-vary with one another.
Consider this covariance example.
Covariance equation: X = A.Y (where X and Y are two variables and A is the covariance matrix).
One has to take the inverse of A to calculate Y. This inverse of A is called an inverse covariance matrix.
FAQs on Covariance
1. What does Covariance Mean?
Covariance is a metric that determines the direction of the relationship of any two random variables. Covariance reveals whether the relationship between both the variables is positive or negative. It helps to understand the total variation of two variables from their expected values. Whether the variables are moving in tandem or tend to show an inverse relationship is that one can gauge the relationship. In any case, one can neither conclude the strength of the relationship nor the dependency of the variables amongst them.
The range of covariance values is positive (+) infinity to negative (+) infinity. Covariance has units or dimensions.
2. What is the Correlation?
Correlation determines the extent or degree of the relationship between both the variables. The correlation coefficient values range from -1 to +1, unlike the covariance values. Correlation is dimensionless. +1 value indicates a strong positive relationship and -1 indicates a strong negative relationship. The relationship between covariance and correlation is well explained with the covariance and correlation formula.
Correlations and Covariance both are subjected to assess the relationship between variables. Correlation helps to measure the bond strength of the relationship between the variables. It is the scaled measure of covariance. It has no dimension. In other words, the correlation coefficient is always a true value.
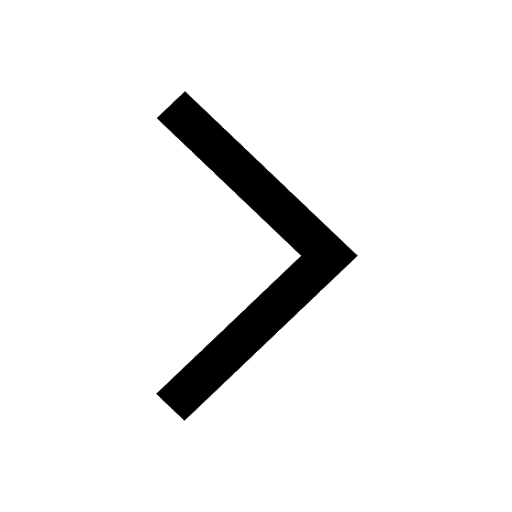
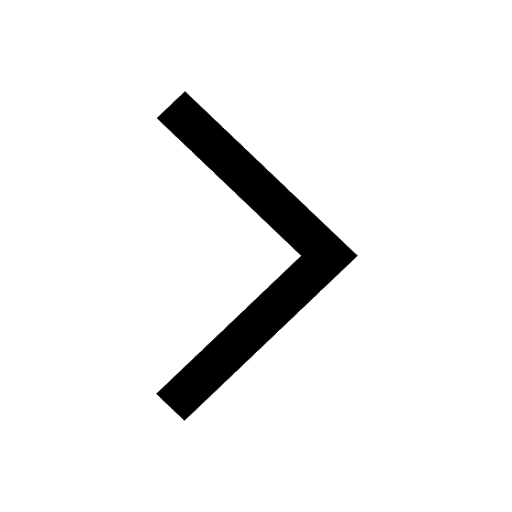
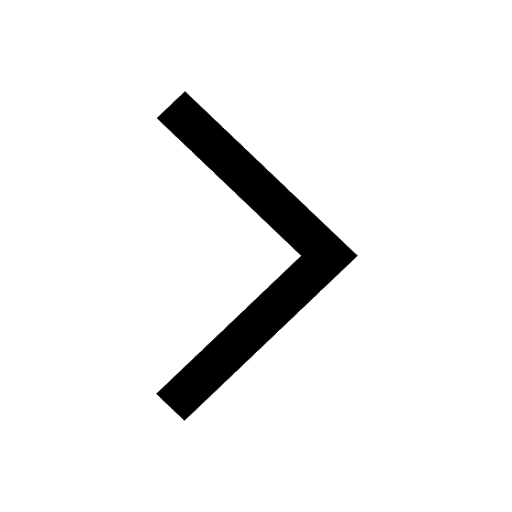
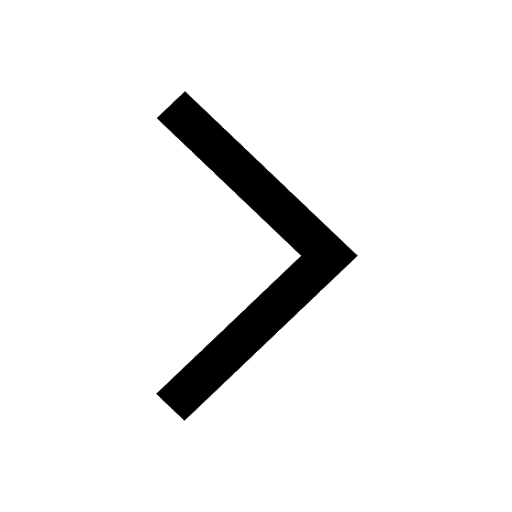
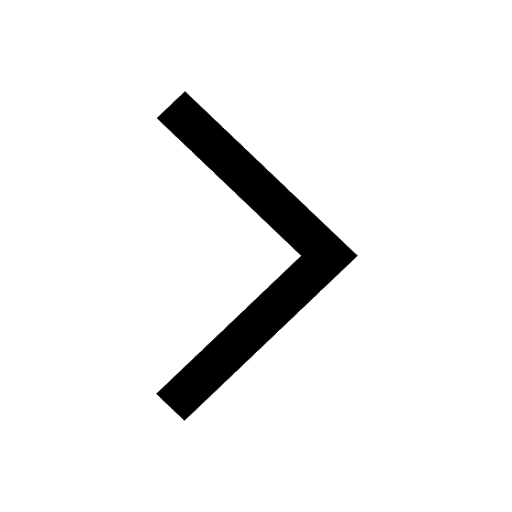
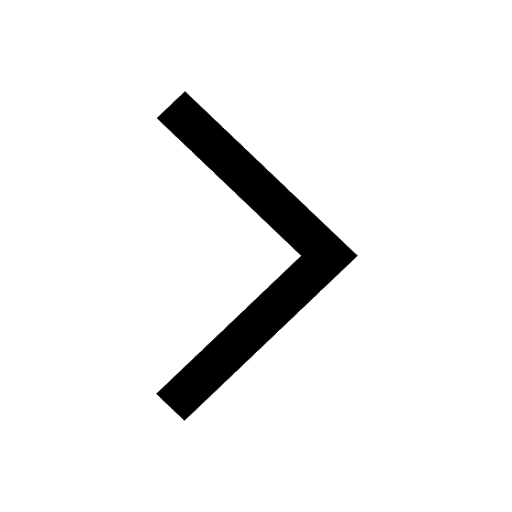
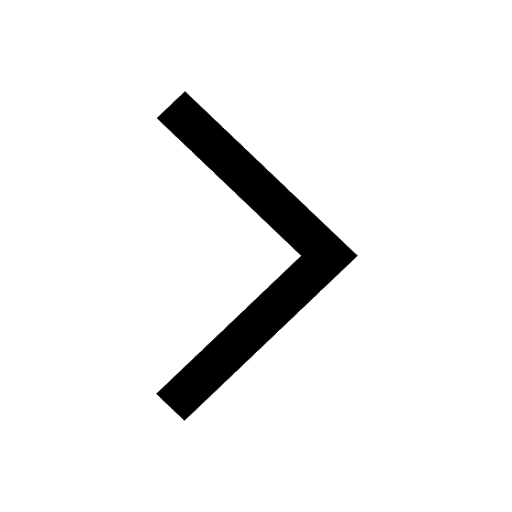
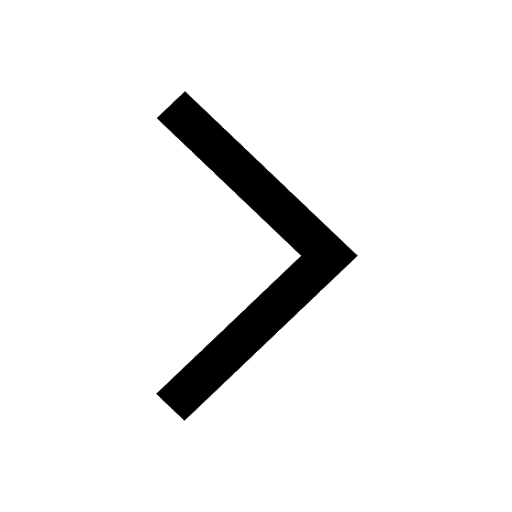
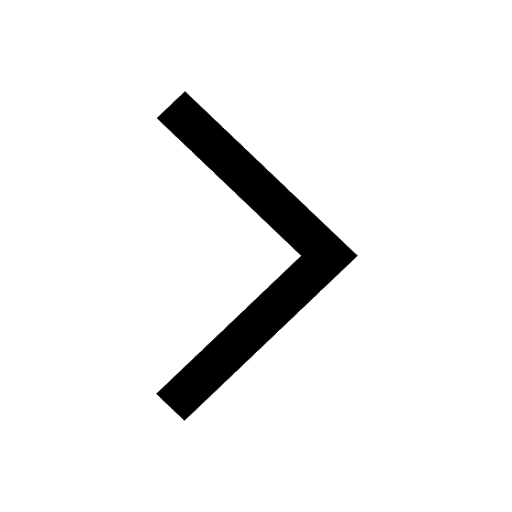
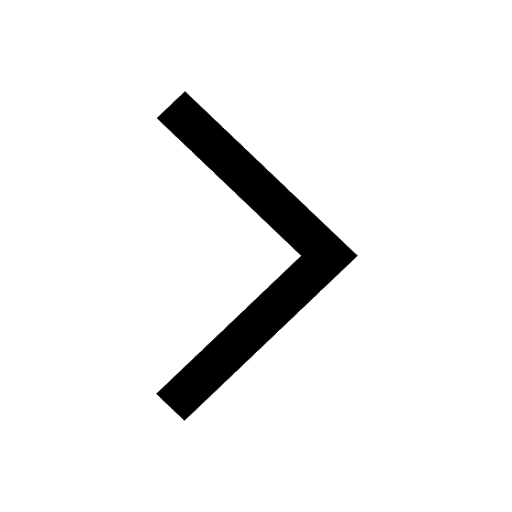
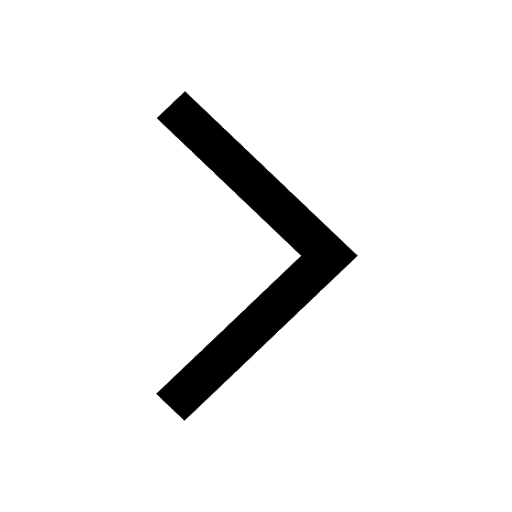
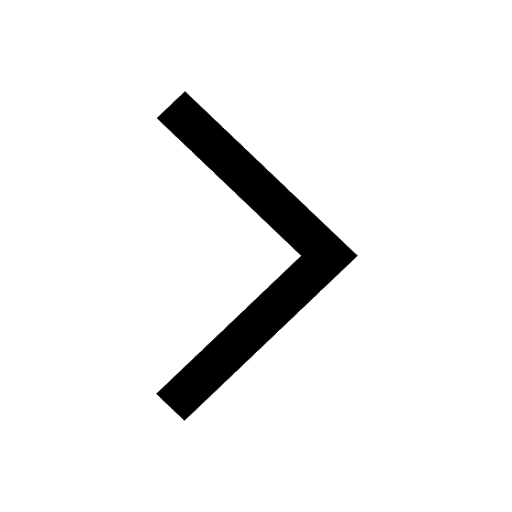
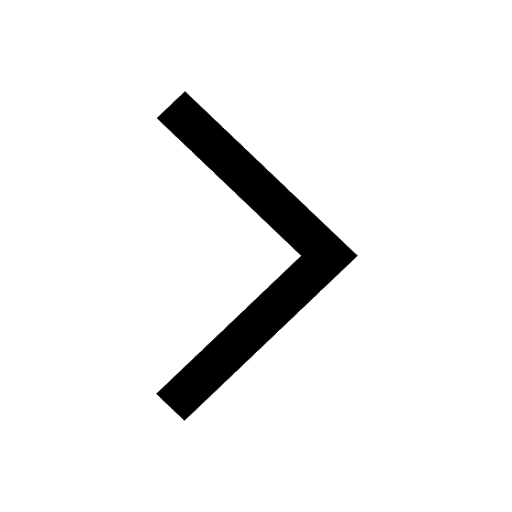
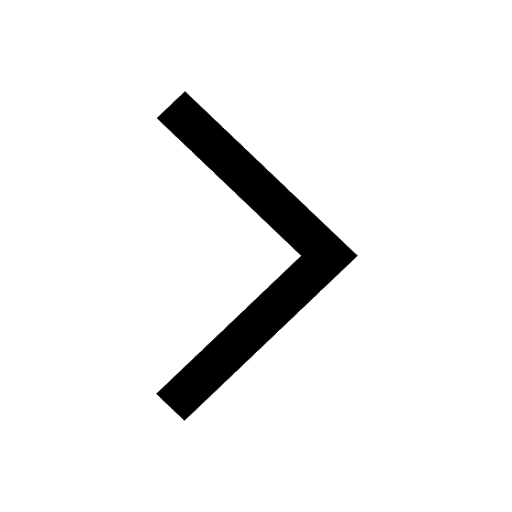
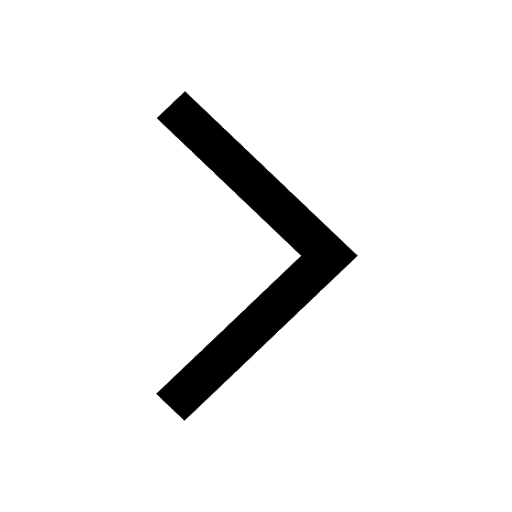
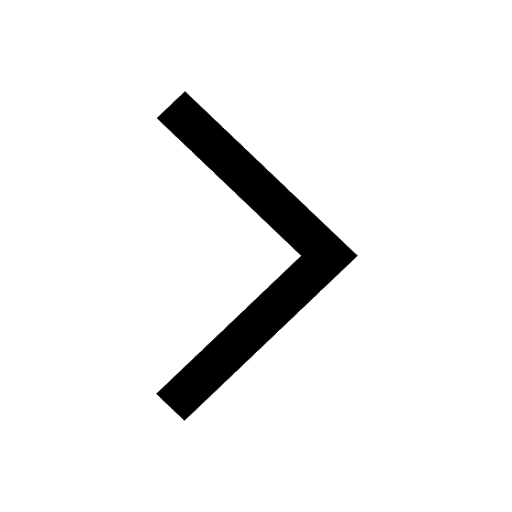
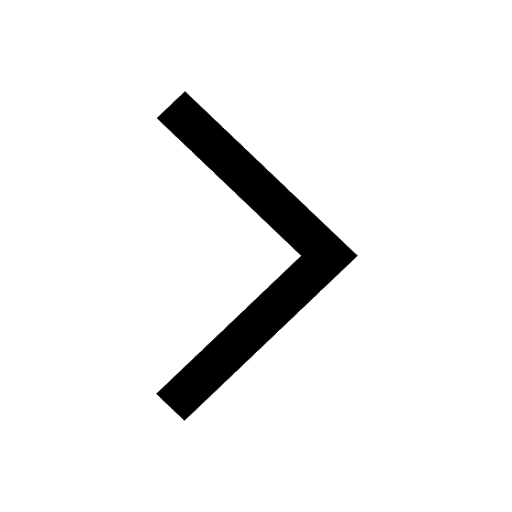
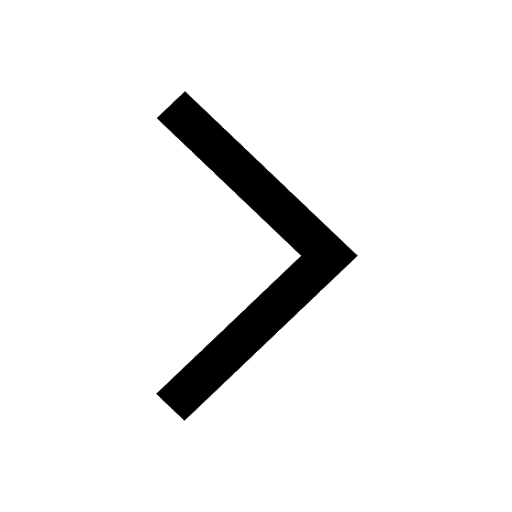