

Interval Estimation Definition
Estimation is the method of drawing conclusions regarding an unknown population parameter from a sample. An estimator is a statistic that is used to calculate the value of a parameter that is undefined. Intervals are statistical estimation approaches that employ survey data to generate value ranges that are likely to contain the population value of interest. Point estimates, on the other hand, are single-value estimates of a population value. Confidence intervals are the most well-known of the various forms of statistical intervals. Certain types of analyses and scenarios, on the other hand, necessitate the use of various ranges that provide different details.
In this article we will learn different interval estimation methods. We will learn prediction interval formula and many more along with examples.
Interval estimation in statistics is the computation of an interval, or set of values, within which a parameter. For example, the mean(average) of a population is most likely to be placed. The confidence coefficient is calculated by choosing intervals such that the parameter falls within them with a 95 or 99 percent probability. As a result, the intervals are referred to as confidence interval estimates. Upper and lower confidence limits are the end points of such an interval.
In comparison to point estimation, which is a single number, interval estimation uses sample data to measure an interval of possible (or probable) values for an unknown population parameter. In certain ways, a point estimate is the best estimate of the parameter based on a sample. Any point calculation, it should go without saying, isn't 100% correct. It's just a guess based on a single random sample. The point estimate would be expected to differ from sample to sample if repeated random samples were taken from the population.
Interval Estimate Formula, is Given Below
μ = \[\bar{x}\]\[\pm\] \[Z_{\frac{a}{2}}\frac{σ}{\sqrt{n}}\]
Where, \[\bar{x}\] = mean
\[Z_{\frac{a}{2}}\] = the confidence coefficient
α = Confidence Level
σ = Standard deviation
n = Sample size
Prediction Interval
Provided particular values of the independent variables, a prediction interval is a range that most likely includes the value of the dependent variable for a single new observation. We're estimating ranges for individual observations rather than the mean value for this sort of interval.
95 Prediction Interval
There is a 95% chance that a future observation will fall within the prediction interval if we collect a sample of observations and measure a 95 percent prediction interval based on that sample. On the other hand, there is a 5% chance that the next observation will not be contained within the interval. If we take 20 samples and determine a prediction interval for each, we can expect 19 of the intervals to contain a single future observation, while 1 of the intervals will not.
This interpretation of the prediction interval is depicted graphically in below diagram
[Image will be Uploaded Soon]
In the above diagram, upper and lower prediction interval bounds for 20 samples each containing 10 pH measurements drawn from the same population are plotted. There are also 20 individual future findings included in the storey. One of the twenty single future pH readings (circled in red) falls outside the associated prediction interval area. For a confidence level of 95 percent, this corresponds to the 5% of periods not anticipated to contain a single future observation.
The most popular use of prediction intervals is in regression statistics, but they can also be used for normally distributed results.
Point Estimate of the Population Mean
The most fundamental point and interval estimation process involves the estimation of a population mean. Assume you want to calculate the population mean,, for a quantitative variable. The sample mean x, where the value of x provides a point estimate of, can be computed using data from a simple random sample.
Since the sample mean is used as a point estimate of the population mean, some error can be expected due to the fact that the point estimate is computed using a sample, or subset of the population. The sampling error is the absolute value of the difference between the sample mean, x , and the population mean,μ, written |x̄ − μ|. A probability assumption about the size of the sampling error is included in interval estimation. The basis for such a statement is the sampling distribution of x .
Point estimation and interval estimation
In statistics, point estimators and interval estimators are the two most common types of estimators. Interval estimation is the polar opposite of point estimation. It yields a single value, while the latter yields a number of results. A point estimator is a statistic that is used to measure the value of a population's unknown parameter. When estimating a single statistic that will be the best approximation of the population's unknown parameter, it uses sample data.
Interval estimation, on the other hand, uses sample data to measure the range of potential values for a population's unknown parameter.
Example of Point Estimate is Given Below:
A single value of a statistic is a point estimate of a population parameter. The sample mean x, for example, is a point estimate of the population mean μ. The sample proportion p is a point estimate of the population proportion P, in the same way.
Common Methods of Finding Point Estimates
The method of point estimation entails using the value of a statistic derived from survey data to achieve the best approximation of the population's corresponding unknown parameter. The point estimators can be calculated using a variety of methods, each with its own set of properties.
Given Below are Two Methods to Find Point Estimates:
Method of Moments - It begins with known facts about a population, which are then applied to a sample of the population. The first step is to create equations that connect population moments to unknown parameters.
The next step is to select a population sample from which to estimate population moments. The sample mean of the population moments is used to solve the equations obtained in step one. This yields the most accurate estimation of unknown population parameters.
Maximum Likelihood Estimator - The maximum likelihood estimator is a point estimation tool that tries to find unknown parameters that increase the likelihood function. It compares data sets and finds the best fit for the data by using the values from a proven model.
For example, a study may be curious about the average weight of premature babies. Because measuring all premature babies in the population will be impractical, the researcher will take a sample from a single area. The researcher will use the maximum likelihood estimator to find the average weight of the entire population of pre-term babies based on the sample data since the weight of pre-term babies follows a normal distribution.
FAQs on Interval Estimation
1. Define Bias in Point Estimation.
Ans: The discrepancy between the estimator's predicted value and the value of the parameter being measured is the bias of a point estimator. The estimator is called unbiased when the expected value of the parameter and the value of the parameter being estimated are equal. Furthermore, the smaller the bias is, the closer the predicted value of a parameter is to the value of the parameter being evaluated.
2. Define Confidence Interval.
Ans: A confidence interval expresses the degree of uncertainty associated with a given statistic. A margin of error is often used for confidence intervals. It indicates how assured you can be that the results of a poll or survey are representative of what you would expect to find if the entire population were surveyed. Confidence intervals and confidence levels are inextricably linked.
3. How Do You Explain a Prediction Interval?
Ans: Given the settings of the predictors, a prediction interval is a sequence of values that is likely to contain the value of a single new observation. For example, we can be 95 percent certain that the next new observation will fall within a 95 percent prediction interval of [5 10].
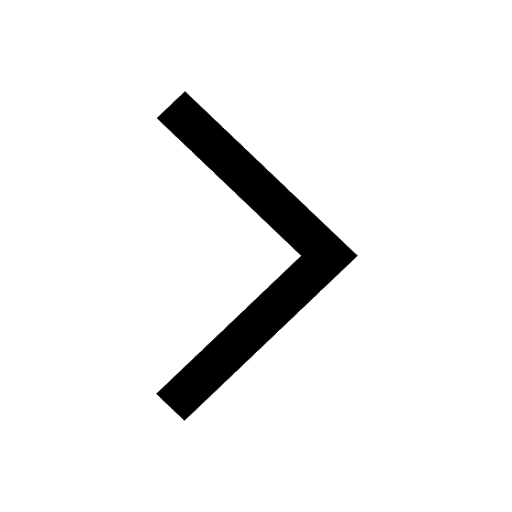
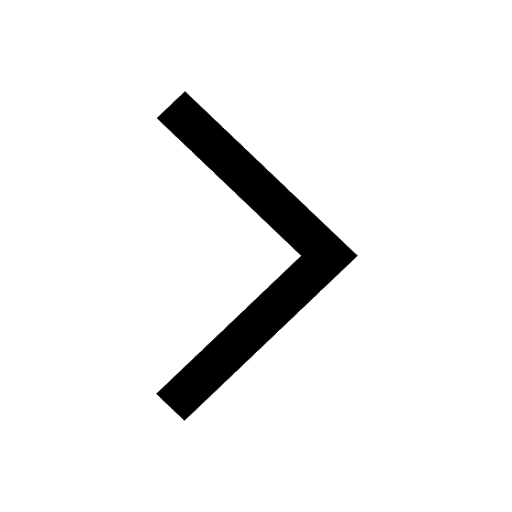
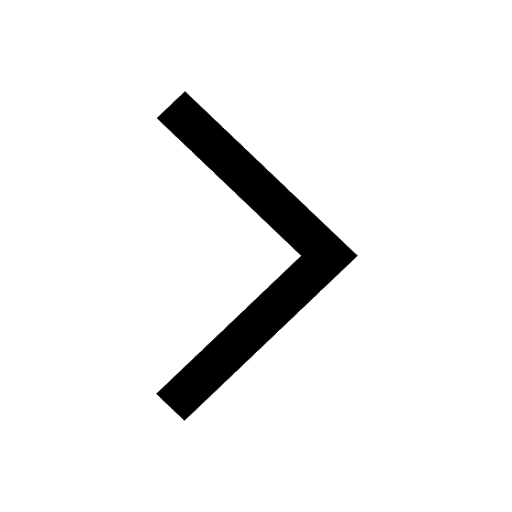
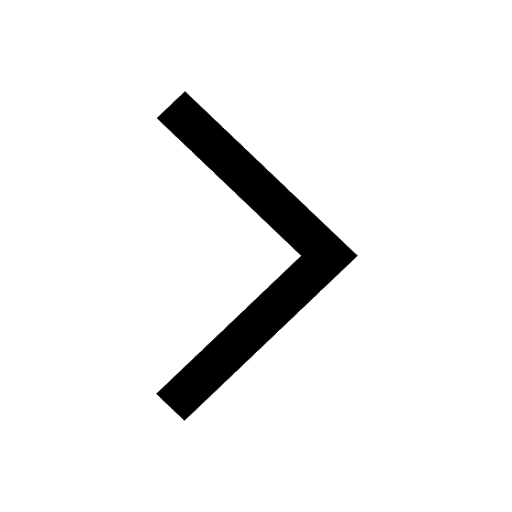
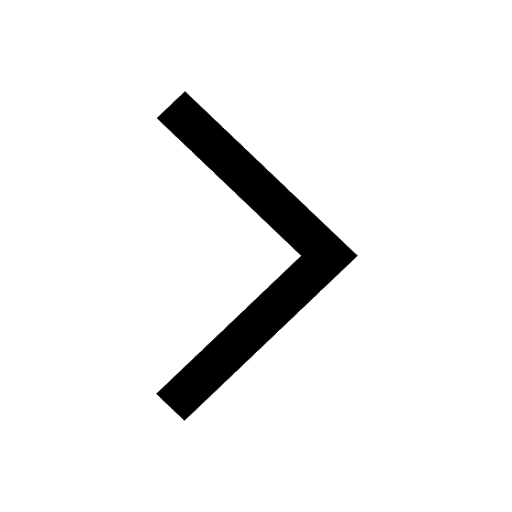
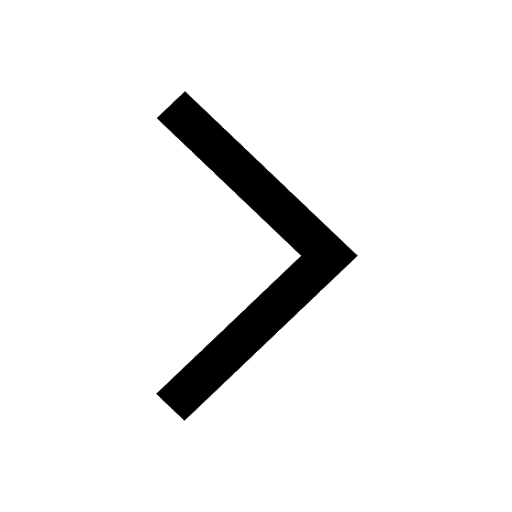