

Introduction to Outlier
In a data collection, outliers are stragglers, which means they are extremely high or extremely low values. In simple words, it’s the data that lies outside other values in a set.
For example, we have a set of random numbers as follows,
2, 98, 101, 103, 106, 109, 112, 205
Here, 2 and 205 are the outliers.
[Image will be Uploaded Soon]
Most of the data points clustered along the straight line very closely, as you can see in the above chart. The outlier is far from other points.
Outlier Meaning
An outlier is an observation in which in a random sample of a population lies an abnormal distance from other values. In a way, this definition leaves it up to the analyst to determine what would be considered abnormal. It is important to classify normal observations before abnormal observations can be picked out.
Defining Outliers
Examination for important features, including symmetry and deviations from assumptions, of the overall shape of the graphed results.
Examination of the information for odd findings that are far away from the data collection. Such points are also classified as outliers.
Inliers
An Inlier, on the other hand, is an inaccurate data value that is simply within a statistical distribution, making it difficult to separate it from good data values. A simple example of an inlier might be a value recorded in the incorrect units in a record, say degrees Fahrenheit rather than degrees Celsius.
Extreme and Mild Outlier
Mild Outlier:
The data values below the first quartile or above the third quartile that lie between 1.5 times and 3.0 times the interquartile scale.
Extreme Outlier:
Any data values that lie more than 3.0 times the interquartile range below the first quartile or above the third quartile are extreme outliers.
How to Find Outliers?
Extreme Value Analysis: The statistical tails of the underlying data distribution are measured.
Probabilistic and Statistical models: From a probabilistic model of the data, evaluate unlikely instances.
Linear Models: Projection techniques that use linear correlations to model the data into lower dimensions. Outliers can be, for instance, main component analysis and data with significant residual errors.
Proximity-based Models: Data instances as determined by cluster, density or nearest neighbor analysis that is separated from the mass of the data.
Information-Theoretic Models: Outliers are detected as data instances that increase the complexity of the dataset (minimum code length).
High-Dimensional Outlier Detection: Methods that scan outlier subspaces provide a higher-dimensional breakdown of distance-based measures.
Causes of Inlier and Outlier
1. Human Mistakes: Errors in data entry.
2. Instrument Mistakes: Errors in the calculation.
3. Experimental Errors: Extraction of data or planning/executing errors for experiments.
4. Intentional: Dummy outliers for evaluating methods of detection.
5. Errors in Data Processing: Data manipulation or unwanted mutations in the data collection.
6. Errors in Sampling: Collecting or combining data from incorrect or different sources.
Uses of Outliers
Outliers help in Fraud detection, fraudulent loan applications, Intrusion detection in the networks, Activity monitoring, Network performance, Satellite image analysis, Detecting novelties in images, Detecting mislabelled data, and many more.
Fun Fact
Do you know that there is an outlier company which is actually a clothing entity? You can find different kinds of outlier jeans which are famous among the people especially the outlier chinos.
Conclusion
Outliers should be properly investigated. They also provide useful information about the procedure under review or the process of collecting and documenting data. One should try to understand why they occurred and whether similar values are likely to continue to occur before contemplating the potential removal of these points from the results. Outliers are considered bad data points most of the time.
FAQs on Outlier
1. What Outliers Mean?
Ans: In the collection of Data, Outliers are extremely high or extremely low values. In simple words, it’s the data that lies outside other values in a set.
2. Are Outliers Bad Data Values?
Ans: Outliers should be properly investigated. They also provide useful information about the procedure under review or the process of collecting and documenting data. Outliers are considered bad data points most of the time.
3. Which Plot is Used to Detect Outliers?
Ans: The use of box plots is one of the easiest methods for identifying outliers. A graphical display to illustrate the distribution of the data is a box plot. The median and the lower and upper quartiles are used by box plots.
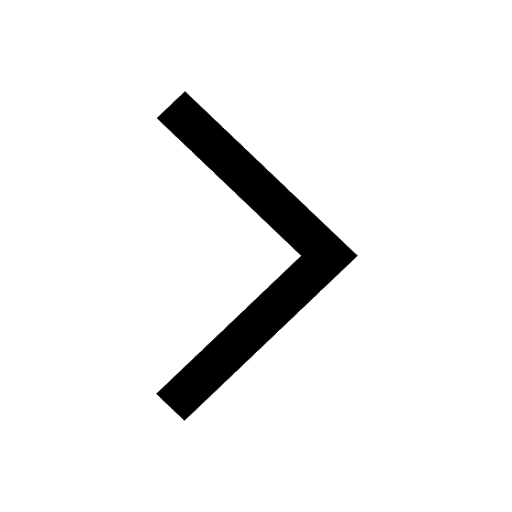
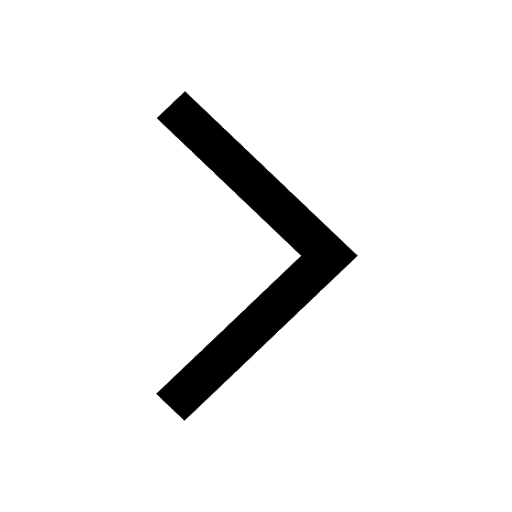
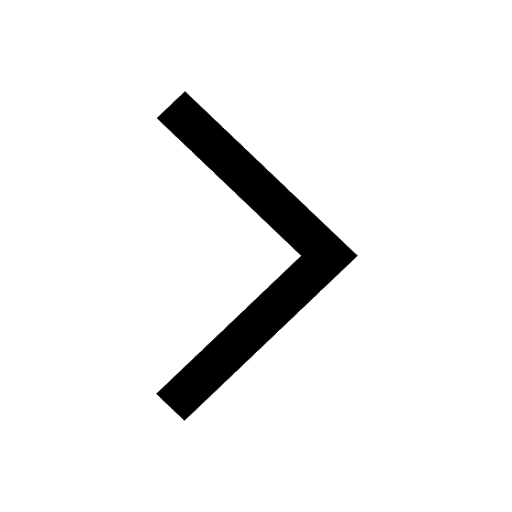
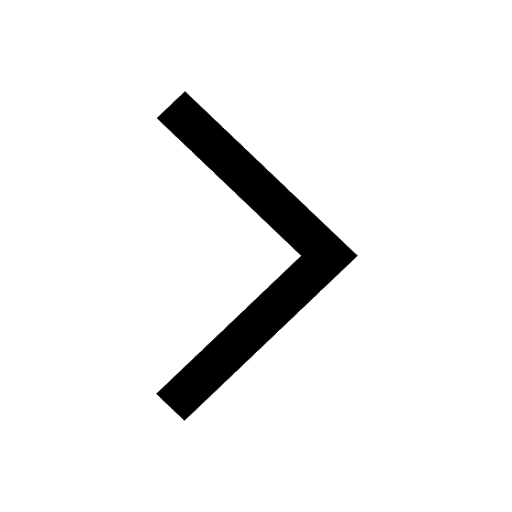
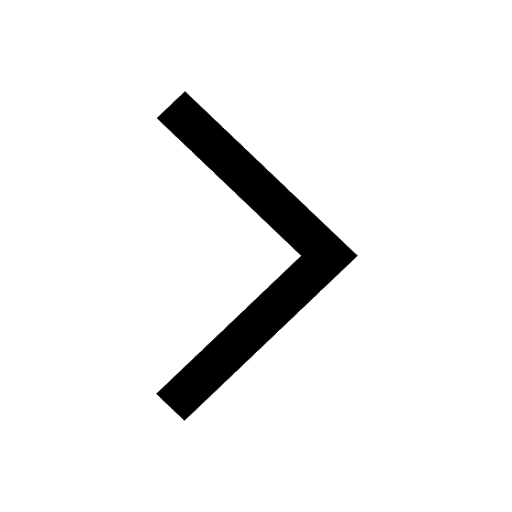
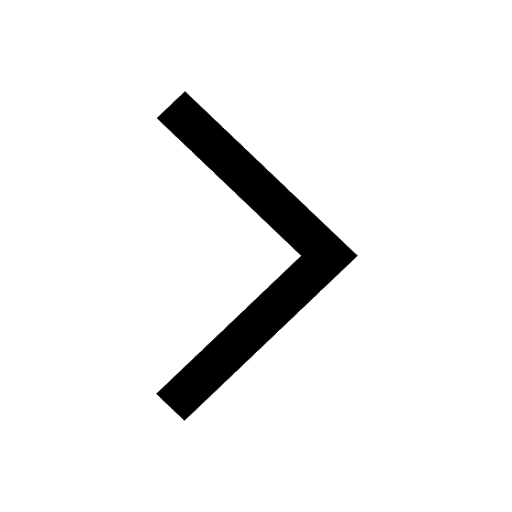
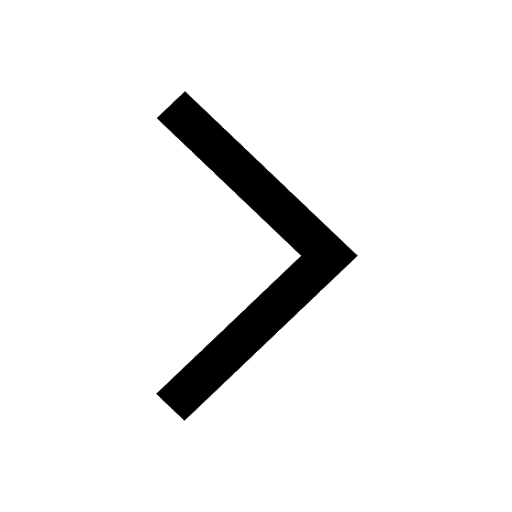
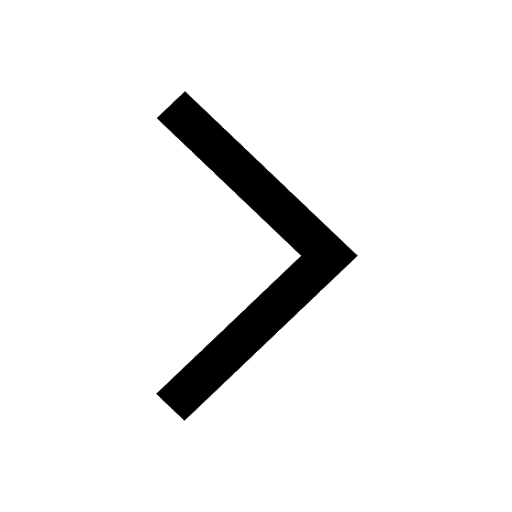
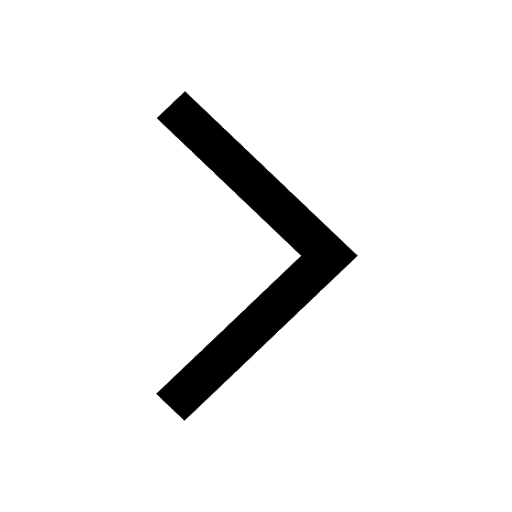
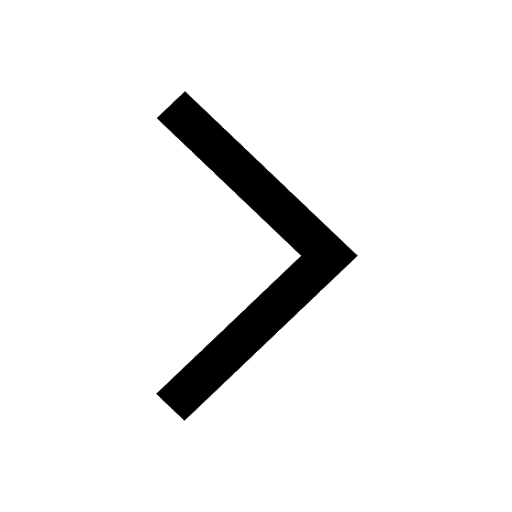
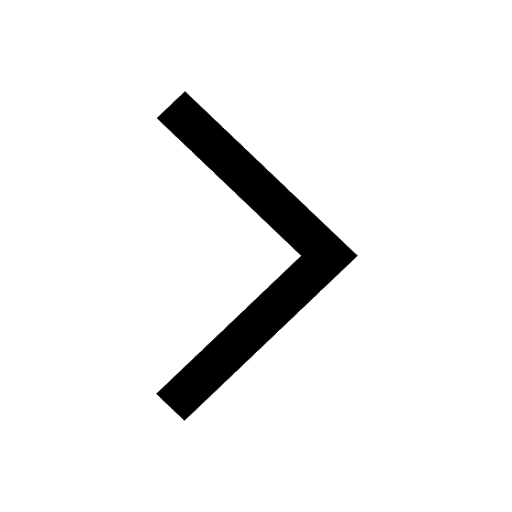
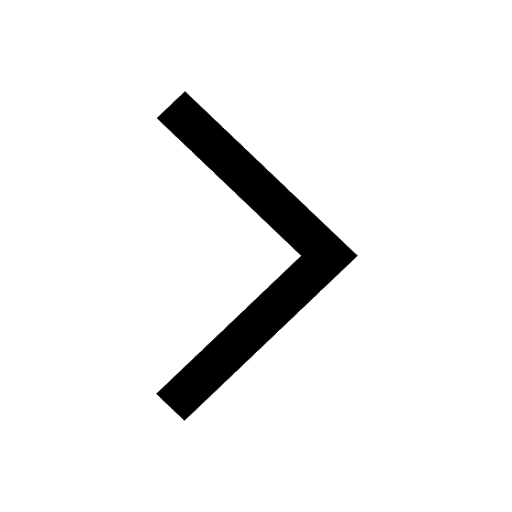
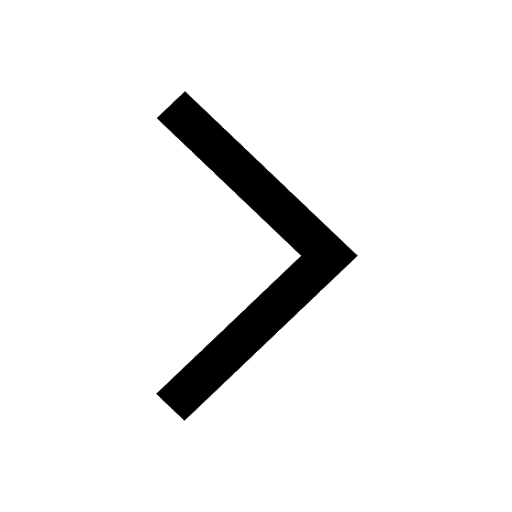
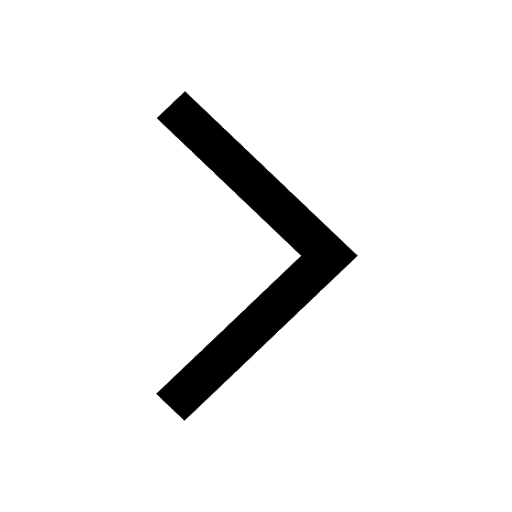
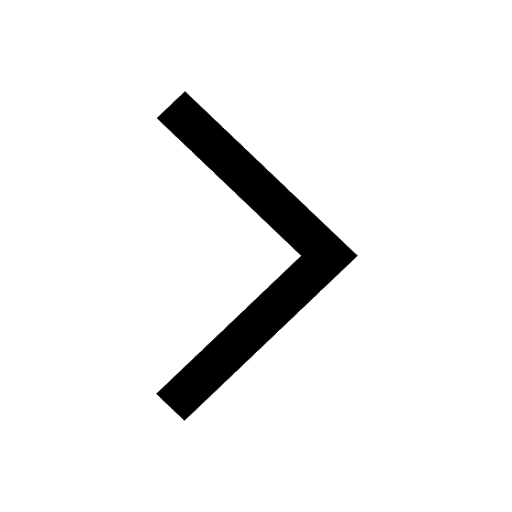
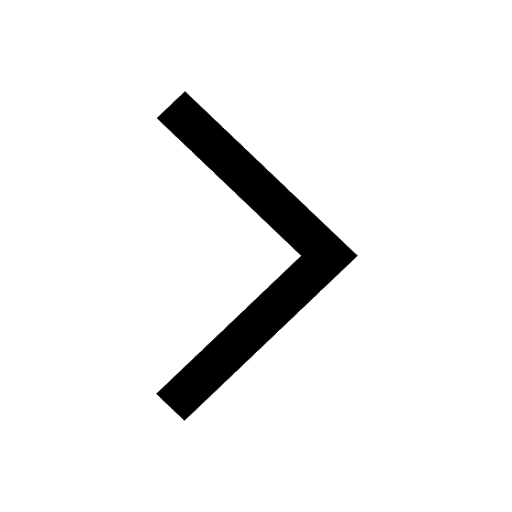
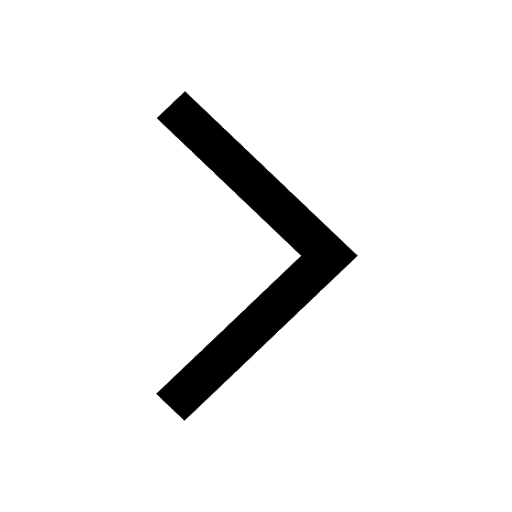
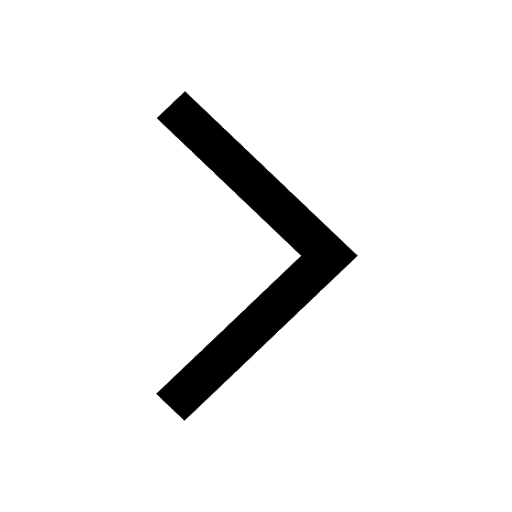