

An Introduction to Point Estimation in Statistics
In Statistics, Estimation Theory and Hypothesis Testing play a major role in determining solutions to certain problems. Point estimation is one of the areas that help people involved in Statistical analysis come to conclusions regarding many different kinds of questions. Point estimation means using data to calculate the value or the point as it serves as a best guess of any given parameter that may be unknown.
What is the Definition of Point Estimation?
Point estimators are defined as functions that can be used to find the approximate value of a particular point from a given population parameter. The sample data of a population is used to find a point estimate or a statistic that can act as the best estimate of an unknown parameter that is given for a population.
What are the Properties of Point Estimators?
It is desirable for a point estimate to be the following :
Consistent - We can say that the larger is the sample size, the more accurate is the estimate.
Unbiased - The expectation of the observed values of various samples equals the corresponding population parameter. Let’s take, for example, We can say that sample mean is an unbiased estimator for the population mean.
Most Efficient That is also Known as Best Unbiased - of all the various consistent, unbiased estimates, the one possessing the smallest variance (a measure of the amount of dispersion away from the estimate). In simple words, we can say that the estimator varies least from sample to sample and this generally depends on the particular distribution of the population. For example, the mean is more efficient than the median (that is the middle value) for the normal distribution but not for more “skewed” ( also known as asymmetrical) distributions.
What are the Methods Used to Calculate Point Estimators?
The maximum likelihood method is a popularly used way to calculate point estimators. This method uses differential calculus to understand the probability function from a given number of sample parameters.
Named after Thomas Bayes, the Bayesian method is another way using which the frequency function of a parameter can be understood. This is a more non-traditional approach. However, in this case, enough information on the distribution of the parameter is not always given but in case it is, then the estimation can be done fairly easily.
What are the Formulae that Can be Used to Measure Point Estimators?
Some common formulae include:
Maximum Likelihood Estimation or MLE
Jeffrey Estimation
Wilson Estimation
Laplace Estimation
What are the Values Needed to Calculate Point Estimators?
The number of successes is shown by S.
The number of trials is shown by T.
The Z–score is shown by z.
Once You Know All the Values Listed Above, You Can Start Calculating the Point Estimate According to the Following Given Equations:
Maximum Likelihood Estimation: MLE = S / T
Laplace Estimation: Laplace equals (S + 1) / (T + 2)
Jeffrey Estimation: Jeffrey equals (S + 0.5) / (T + 1)
Wilson Estimation: Wilson equals (S + z²/2) / (T + z²)
Once All Four Values have been Calculated, You Need to Choose the Most Accurate One.
This should be done According to the Following Rules Listed below:
If the value of MLE ≤ 0.5, the Wilson Estimation is the most accurate.
If the value of MLE - 0.5 < MLE < 0.9, then the Maximum Likelihood Estimation is the most accurate.
If 0.9 < MLE, then the smaller of Jeffrey and Laplace Estimations is said to be the most accurate.
FAQs on Point Estimation in Statistics
1. What is the meaning of an unbiased point estimator?
It needs to be understood that a point estimation is after all an estimated or approximate value, not a definite one. This always leaves room for error. There can be man-point estimators for a given sample of the population, but the best one is the one that is the most unbiased and efficient. This means that it should have the least variance. The efficiency of the distributor is usually dependent on the kind of sample that is given. This would also affect the probability of it being the most efficient.
2. What is interval estimation?
Just like point estimation, this is another method through which a given sample can be analyzed. In this method, in a given sample, the interval of the possible values of an unknown parameter is calculated. The interval is calculated in a way that it falls between 95 percent probability or higher than that as well. This is also called the confidence interval and it can imply how reliable and efficient the interval calculated is. There are two endpoints which are called the upper and lower limits.
3. Is there a distinction between point and interval estimation?
Interval estimation is the exact opposite of point estimation. They both work on a given sample parameter of the population but use different approaches to come to find the most efficient and unbiased value. In point estimation, results in one specific value for the estimation, and in interval estimation, a number of values are calculated. Internal estimation is usually considered to be a more accurate representation of an estimate as opposed to pointing estimation. This is because it gives a range of values that is usually closer to the correct value.
4. What is the Method of Moments approach to finding point estimation?
This method was introduced in the year 1887 and is used to generate the value of a point in a given parameter. This uses the idea of understanding facts about a certain population and then using that to a sample of that population. The first step to this is to frame equations that will fit into the parameters of the population sample. Then a sample is drawn to be the estimate of the population parameter and the first equations are used to calculate the mean value. This can usually produce a very accurate estimate.
5. What is the Maximum Likelihood Estimator?
Maximum Likelihood Estimator or MLE is a way through which point estimation can be made. It is used to find unknown parameters which can maximize the likelihood of a certain outcome. This approach uses a model and a range of data to compare and give the most accurate value. This always uses sample data from the population in order to find the mean value of the same. It is usually the most common approach to finding out an appointed estimator.
6. What is Point Estimation and Interval Estimation?
A point estimate can be defined as a single value estimate of a parameter. For instance, a sample mean is also known to be a point estimate of a population mean. An interval estimate generally gives you a range of values where the parameter is expected to lie and a confidence interval is the most common type of interval estimate.
7. What is the Purpose of Point Estimation? What is a Point Estimator?
Basically point estimators are known to be functions that are used to find an approximate or an estimated value of a population parameter from various random samples of the population.
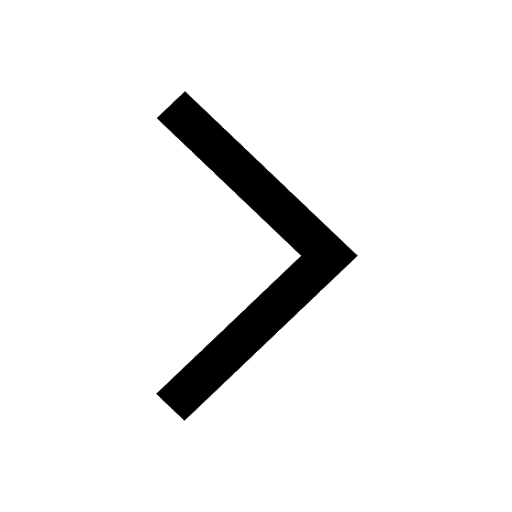
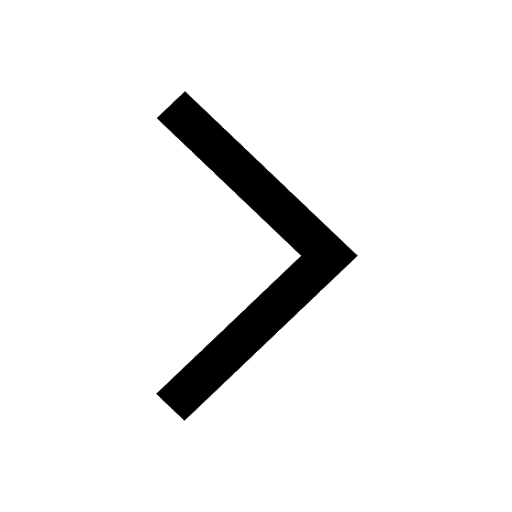
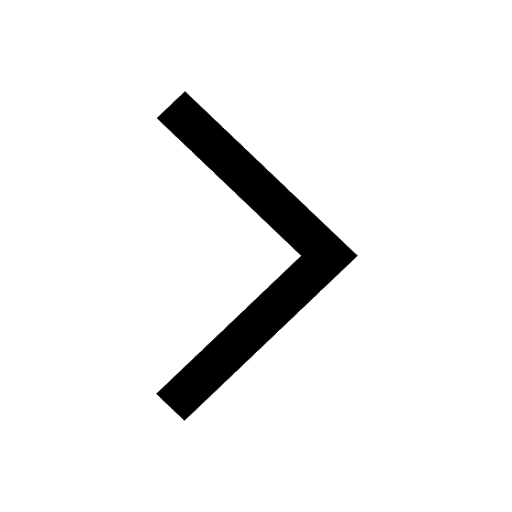
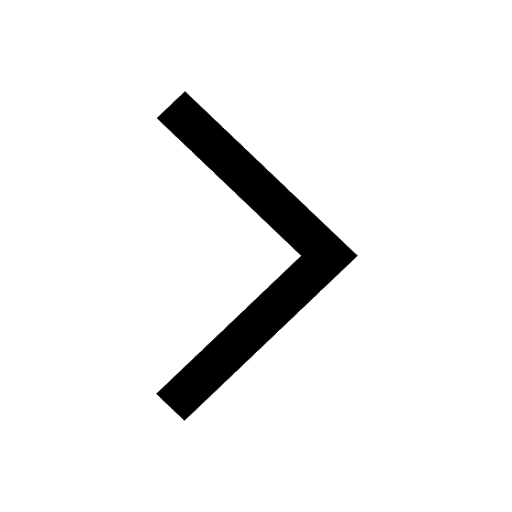
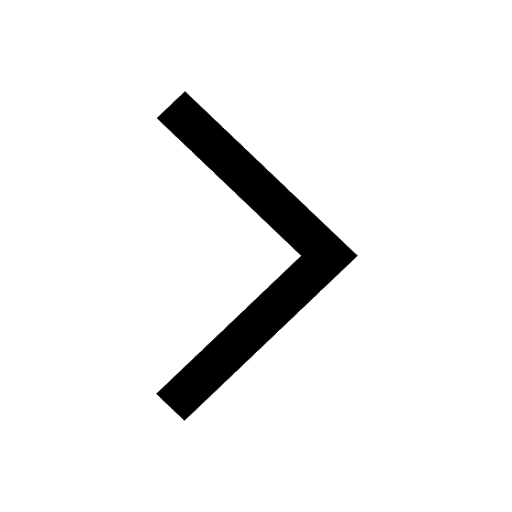
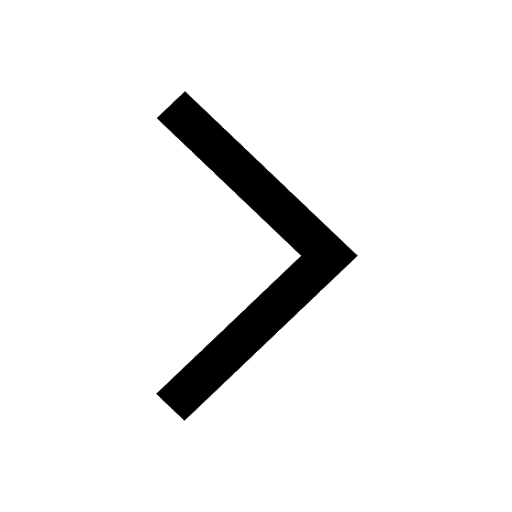