

Variance and Standard Deviation
Variance Definition in Statistics
In statistics, the difference between the mean and its data points is known as the variance. In simpler terms, it is the measure of how far a set of data points are far from their mean value. When you have to solve questions, you need to use the following variance formula:
Var ( n ) = E [ (n - μ)2 ]
If the formula has to be described in words, it goes by - the variance is the expectation of the deviation from its mean value that is squared.
Here, μ is equal to the value of E ( n ) and the equation stated above can also be expressed as:
Var (n) = E [(n - μ)2]
Var (n) = E [n2 - 2n E (n) + (E (n))2 ]
Var (n) = E (n2) - 2 E(n) E(n) + (E(n))2
Var (n) = E(x2) - (E(n))2
Since we have covered the formula of the variance, let us try and understand the relationship between standard deviation and the variance.
Variance Formula in Statistics
Previously, we studied that the variance is the square of standard deviation.
This can be expressed as Variance = σ2 = standard deviation2
Some of the other formulas that correspond with it are:
Population deviation σ = \[\sqrt {\frac{{\sum {{{\left( {n - \mu } \right)}^2}} }}{N}} \]and
Standard deviation S = \[\sqrt {\frac{{\sum {\left( {n - \underline n } \right)} }}{{N - 1}}} \]
Here,
X - Number of observations
μ - cumulative mean of values
N - Total values
Variance Properties
The variance of random variable n has the following properties.
Var ( N + k ) = var ( N ), here k is constant value.
Var ( kN ) = k2. Var ( N ), here k is constant value.
Var ( rN + s) = r2.Var( N ), here r and s can be constants.
If N1 , N2, N3, N4, . . . . . . . . . . . . . . Nn are n number of independent and random values or variables.
Variance Example
Question 1: Find the variance of the number 1, 2, 3, 4, 5, 6, 7, 8, 9, 10.
Solution:
Find the mean of the 10 values: Mean = ( 1 + 2 + 3 + 4 + 5 + 6 + 7 + 8 + 9 + 10 ) / 10
Mean = ( 55 ) / 10
Mean = 5.5
Now, we need to find the population variance
σ2 = \[\sqrt {\frac{{\sum {\left( {n - \underline n } \right)} }}{{N - 1}}} \]
= \[\frac{{82.5}}{{10}}\]
Question 2: Find the variance of the number 4, 2, 8, 6, 12, 17, 14, 20.
Solution:
Find the mean of the 10 values: Mean = ( 4+ 2 + 8 + 6 + 12 + 17 + 14 + 20 ) / 8
Mean = ( 83 ) / 8
Mean = 10.375
Now, we need to find the population variance
σ2 = \[\sqrt {\frac{{\sum {\left( {n - \underline n } \right)} }}{{N - 1}}} \]
= \[\frac{{214.484375}}{8}\]
= 26.8105469
Question 3: Find the variance of the number 11, 12, 13, 14, 15, 16, 17, 18, 19, 20.
Solution:
Find the mean of the 10 values: Mean = ( 1 + 2 + 3 + 4 + 5 + 6 + 7 + 8 + 9 + 10 ) / 10
Mean = ( 155 ) / 10
Mean = 15.5
Now, we need to find the population variance
σ2 = \[\sqrt {\frac{{\sum {\left( {n - \underline n } \right)} }}{{N - 1}}} \]
= \[\frac{{82.5}}{{10}}\]
= 8.25
FAQs on Variance
1. What is the Variance?
In statistics, the difference between the mean and its data points is known as the variance. In simpler terms, it is the measure of how far a set of data points are far from their mean value. When you have to solve questions, you need to use the following equation:
Var ( n ) = E [ (n - μ)2 ]
If the formula has to be described in words, it goes by - the variance is the expectation of the deviation from its mean value that is squared.
2. What are the Properties of Variance?
-Var ( N + k ) = var ( N ), here k is constant value.
-Var ( kN ) = k2. Var ( N ), here k is constant value.
-Var ( rN + s) = r2.Var( N ), here r and s can be constants.
-If N1 , N2, N3, N4, . . . . . . . . . . . . . . Nn are n number of independent and random values or variables.
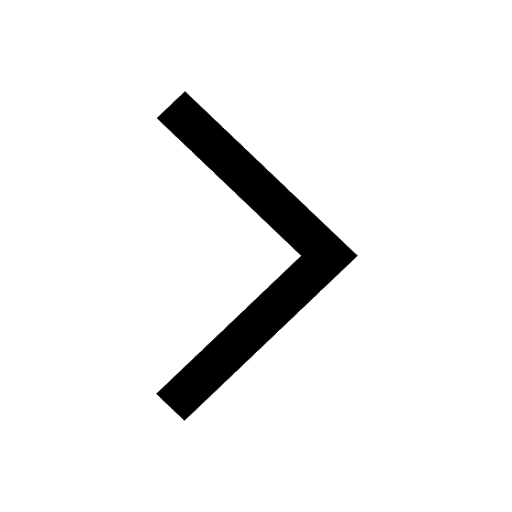
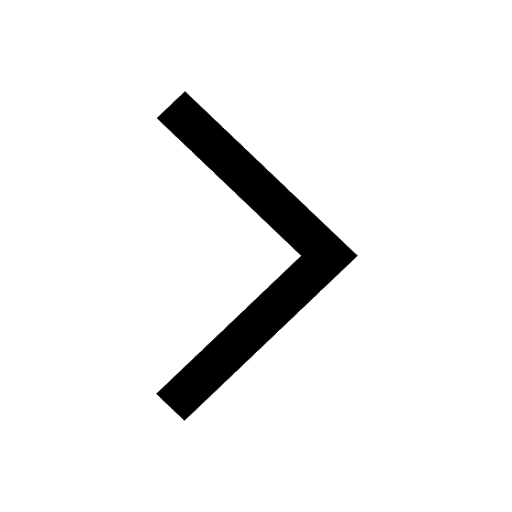
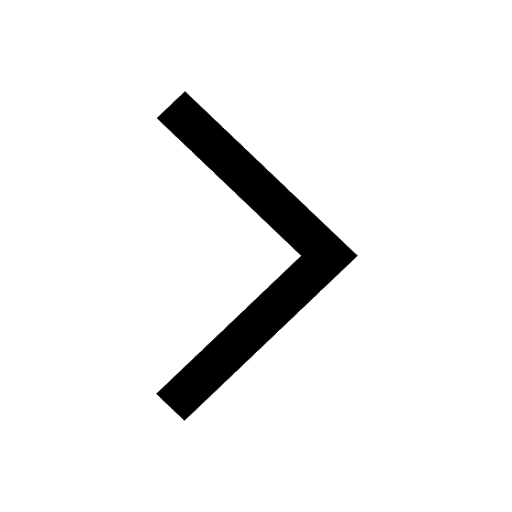
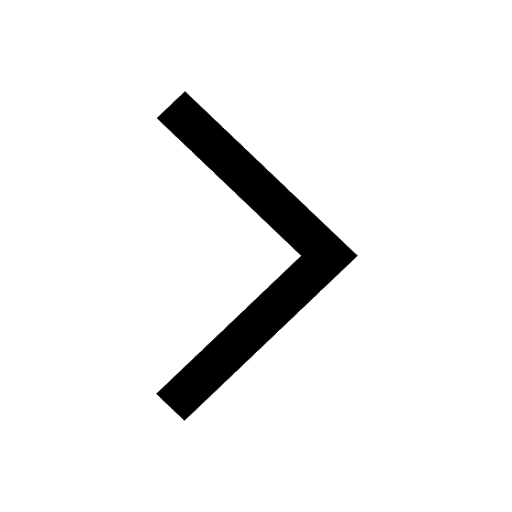
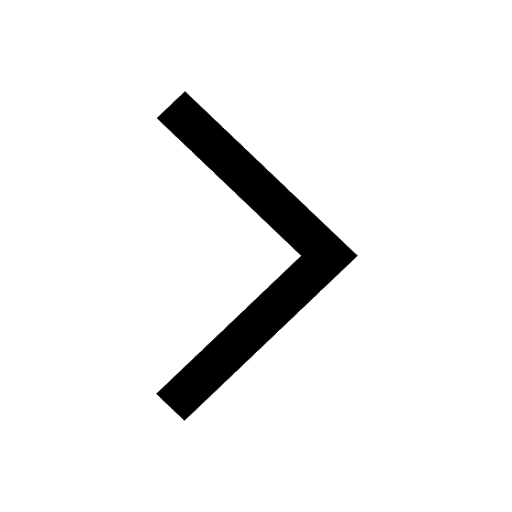
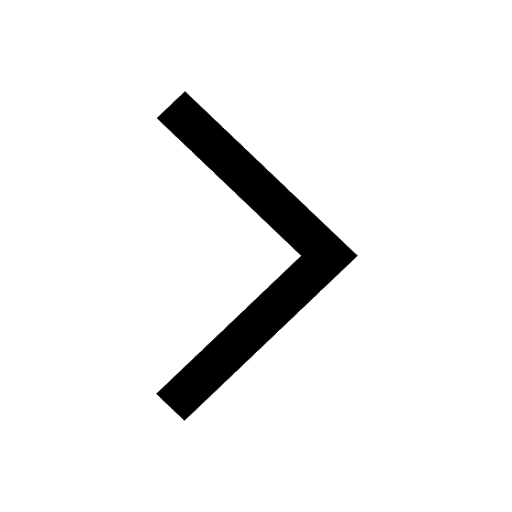
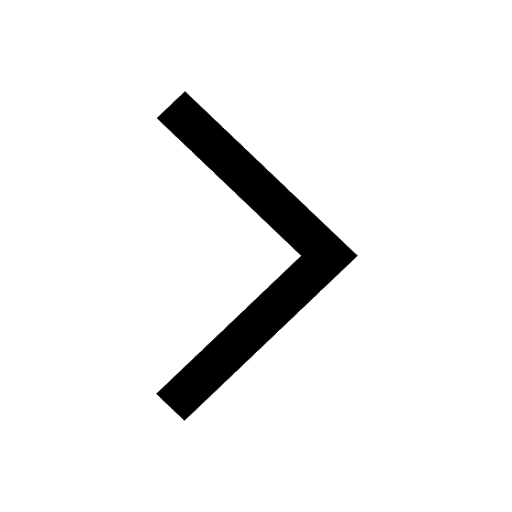
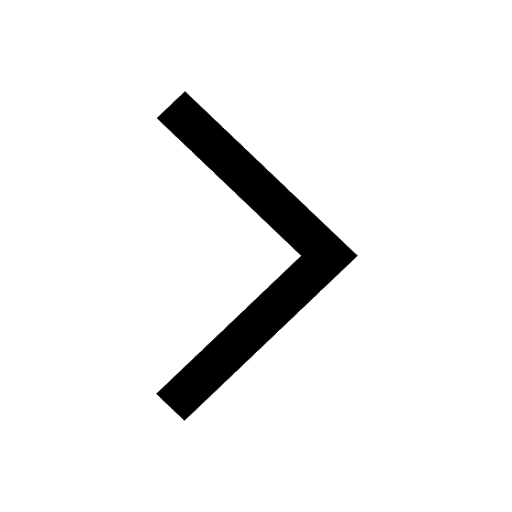
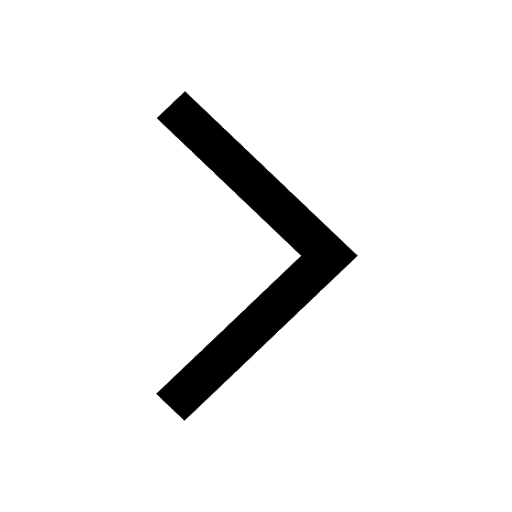
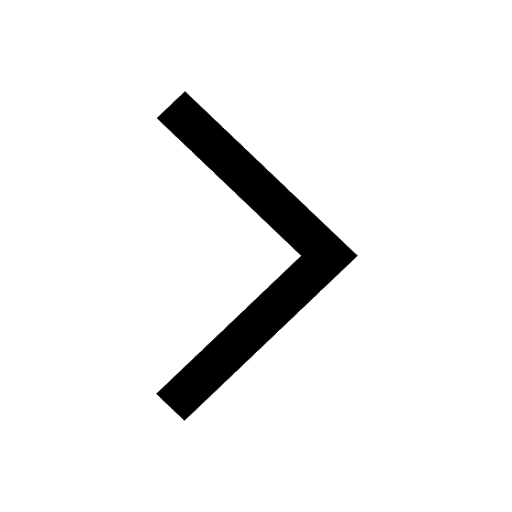
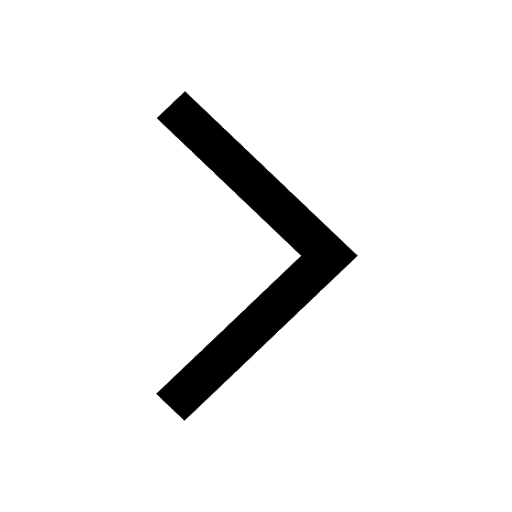
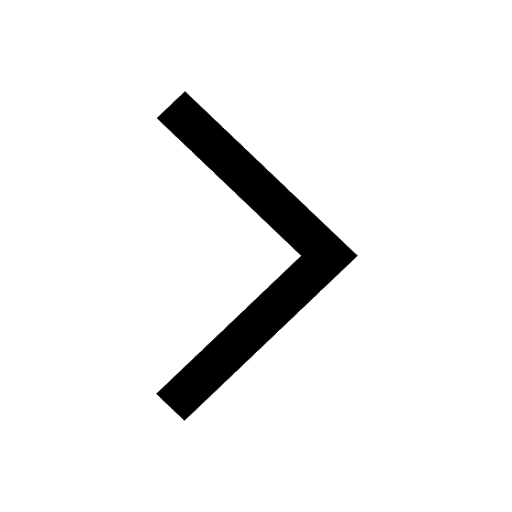
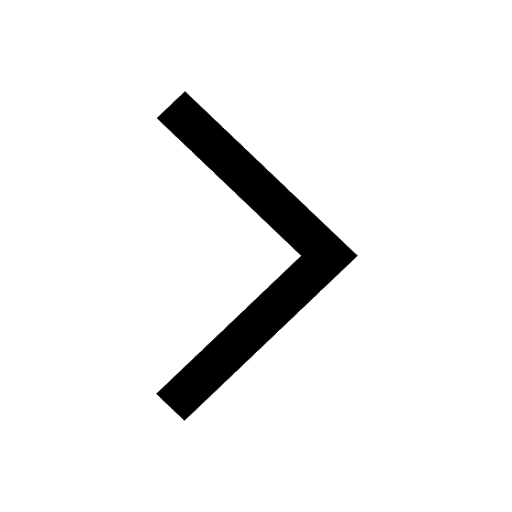
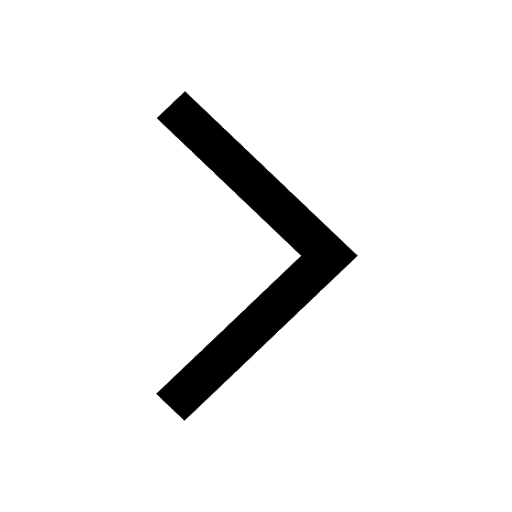
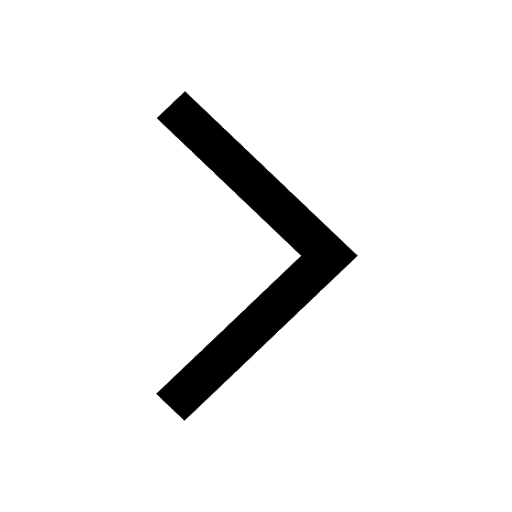
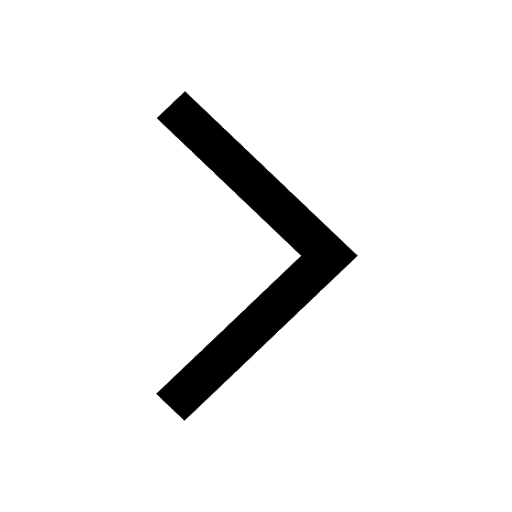
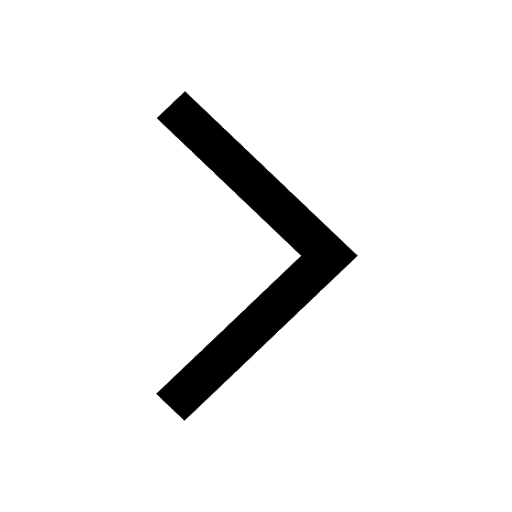
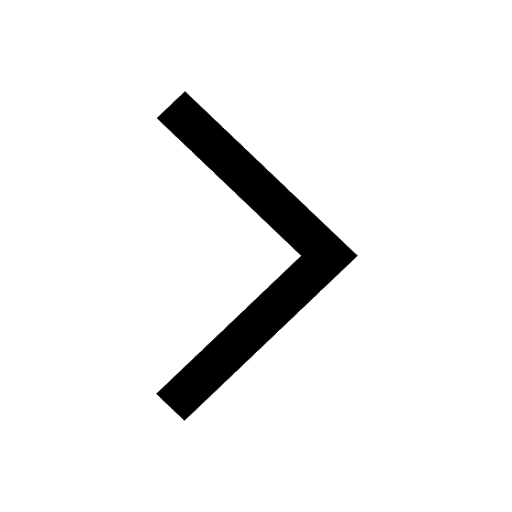