
Answer
451.2k+ views
Hint: We need to find the correlation coefficient between \[x + y\] and \[x - y\]. Let \[p = x + y\] and \[q = x - y\]. We will find the variance of these variates. Then, we will calculate the covariance between them. Finally, we will find the correlation coefficient using the calculated variances and covariance. The correlation coefficient between two variates is the ratio of the covariance between them, and the product of their standard deviations.
Formula Used:
We will use the following formulas:
1.The covariance between two variates \[x\] and \[y\] is given by the formula \[{\mathop{\rm cov}} \left( {x,y} \right) = E\left( {x - \overline x } \right)\left( {y - \overline y } \right)\], where \[\overline x \] is the mean of the variate \[x\], and \[\overline y \] is the mean of the variate \[y\].
2.The product of the sum and difference of two numbers is given by the algebraic identity \[\left( {a - b} \right)\left( {a + b} \right) = {a^2} - {b^2}\].
3.The variance of a variate \[z\] is given by the formula \[E{\left( {z - \overline z } \right)^2}\].
Complete step-by-step answer:
Let \[p = x + y\] and \[q = x - y\].
We know that if three variates are such that \[z = x + y\], then \[\overline z = \overline x + \overline y \].
Therefore, since \[p = x + y\] and \[q = x - y\], we get
\[\overline p = \overline x + \overline y \] and \[\overline q = \overline x - \overline y \]
The covariance between two variates \[x\] and \[y\] is given by the formula \[{\mathop{\rm cov}} \left( {x,y} \right) = E\left( {x - \overline x } \right)\left( {y - \overline y } \right)\], where \[\overline x \] is the mean of the variate \[x\], and \[\overline y \] is the mean of the variate \[y\].
Therefore, we get
\[ \Rightarrow {\mathop{\rm cov}} \left( {p,q} \right) = E\left( {p - \overline p } \right)\left( {q - \overline q } \right)\]
Substituting \[p = x + y\], \[q = x - y\], \[\overline p = \overline x + \overline y \], and \[\overline q = \overline x - \overline y \] in the expression, we get
\[ \Rightarrow {\mathop{\rm cov}} \left( {p,q} \right) = E\left[ {\left( {x + y} \right) - \left( {\overline x + \overline y } \right)} \right]\left[ {\left( {x - y} \right) - \left( {\overline x - \overline y } \right)} \right]\]
Rewriting the expressions, we get
\[\begin{array}{l} \Rightarrow {\mathop{\rm cov}} \left( {p,q} \right) = E\left[ {x + y - \overline x - \overline y } \right]\left[ {x - y - \overline x + \overline y } \right]\\ \Rightarrow {\mathop{\rm cov}} \left( {p,q} \right) = E\left[ {\left( {x - \overline x } \right) + \left( {y - \overline y } \right)} \right]\left[ {\left( {x - \overline x } \right) - \left( {y - \overline y } \right)} \right]\end{array}\]
The product of the sum and difference of two numbers is given by the algebraic identity \[\left( {a - b} \right)\left( {a + b} \right) = {a^2} - {b^2}\].
Therefore, the equation becomes
\[ \Rightarrow {\mathop{\rm cov}} \left( {p,q} \right) = E\left[ {{{\left( {x - \overline x } \right)}^2} - {{\left( {y - \overline y } \right)}^2}} \right]\]
The expression \[E\left( {x + y} \right)\] can be written as \[E\left( x \right) + E\left( y \right)\].
Therefore, we get
\[ \Rightarrow {\mathop{\rm cov}} \left( {p,q} \right) = E{\left( {x - \overline x } \right)^2} - E{\left( {y - \overline y } \right)^2}\]
The variance of a variate \[z\] is given by the formula \[E{\left( {z - \overline z } \right)^2}\].
Therefore, we get
Variance of \[x\] \[ = E{\left( {x - \overline x } \right)^2}\]
Variance of \[y\] \[ = E{\left( {y - \overline y } \right)^2}\]
It is given that the two variates \[x\] and \[y\] have standard deviations \[{\sigma _x}\] and \[{\sigma _y}\] respectively.
Variance is the square of the standard deviation.
Therefore, we get the equations
\[\sigma _x^2 = E{\left( {x - \overline x } \right)^2}\] and \[\sigma _y^2 = E{\left( {y - \overline y } \right)^2}\]
Substituting \[\sigma _x^2 = E{\left( {x - \overline x } \right)^2}\] and \[\sigma _y^2 = E{\left( {y - \overline y } \right)^2}\] in the equation \[{\mathop{\rm cov}} \left( {p,q} \right) = E{\left( {x - \overline x } \right)^2} - E{\left( {y - \overline y } \right)^2}\], we get
\[ \Rightarrow {\mathop{\rm cov}} \left( {p,q} \right) = \sigma _x^2 - \sigma _y^2\]
Now, we will find the variance of the variates \[p = x + y\] and \[q = x - y\].
The variance of a variate \[z\] is given by the formula \[E{\left( {z - \overline z } \right)^2}\].
Therefore, we get
\[ \Rightarrow \] Variance of \[p\] \[ = E{\left( {p - \overline p } \right)^2}\]
\[ \Rightarrow \] Variance of \[q\] \[ = E{\left( {q - \overline q } \right)^2}\]
Substituting \[p = x + y\], \[q = x - y\], \[\overline p = \overline x + \overline y \], and \[\overline q = \overline x - \overline y \] in the expressions, we get
\[ \Rightarrow \] Variance of \[p\] \[ = E{\left[ {\left( {x + y} \right) - \left( {\overline x + \overline y } \right)} \right]^2}\]
\[ \Rightarrow \] Variance of \[q\] \[ = E{\left[ {\left( {x - y} \right) - \left( {\overline x - \overline y } \right)} \right]^2}\]
Rewriting the expressions, we get
\[ \Rightarrow \]Variance of \[p\] \[ = E{\left[ {\left( {x - \overline x } \right) + \left( {y - \overline y } \right)} \right]^2}\]
\[ \Rightarrow \]Variance of \[q\] \[ = E{\left[ {\left( {x - \overline x } \right) - \left( {y - \overline y } \right)} \right]^2}\]
Expanding the terms using algebraic identity, \[{\left( {a + b} \right)^2} = {a^2} + {b^2} + 2ab\], we get
\[ \Rightarrow \] Variance of \[p\] \[ = E{\left[ {\left( {x - \overline x } \right)} \right]^2} + E{\left[ {\left( {y - \overline y } \right)} \right]^2} + 2E\left[ {\left( {x - \overline x } \right)\left( {y - \overline y } \right)} \right]\]
\[ \Rightarrow \]Variance of \[q\] \[ = E{\left[ {\left( {x - \overline x } \right)} \right]^2} + E{\left[ {\left( {y - \overline y } \right)} \right]^2} - 2E\left[ {\left( {x - \overline x } \right)\left( {y - \overline y } \right)} \right]\]
Since the two variates \[x\] and \[y\] are uncorrelated, we get \[E\left[ {\left( {x - \overline x } \right)\left( {y - \overline y } \right)} \right] = 0\].
Therefore, the equations become
\[ \Rightarrow \]Variance of \[p\] \[ = E{\left[ {\left( {x - \overline x } \right)} \right]^2} + E{\left[ {\left( {y - \overline y } \right)} \right]^2}\]
\[ \Rightarrow \] Variance of \[q\] \[ = E{\left[ {\left( {x - \overline x } \right)} \right]^2} + E{\left[ {\left( {y - \overline y } \right)} \right]^2}\]
Thus, we get
\[ \Rightarrow \] Variance of \[p\] \[ = \sigma _x^2 + \sigma _y^2\]
\[ \Rightarrow \] Variance of \[q\] \[ = \sigma _x^2 + \sigma _y^2\]
Taking the square roots on both the sides, we get the standard deviations as
\[ \Rightarrow \] Standard deviation of \[p\] \[ = \sqrt {\sigma _x^2 + \sigma _y^2} \]
\[ \Rightarrow \] Standard deviation of \[q\] \[ = \sqrt {\sigma _x^2 + \sigma _y^2} \]
Finally, we will find the coefficient of correlation of the two variates \[p = x + y\] and \[q = x - y\].
Therefore, we get
Coefficient of correlation \[ = \dfrac{{{\mathop{\rm cov}} \left( {p,q} \right)}}{{s.d.\left( p \right)s.d.\left( q \right)}}\]
Substituting \[s.d.\left( p \right) = \sqrt {\sigma _x^2 + \sigma _y^2} \], \[s.d.\left( q \right) = \sqrt {\sigma _x^2 + \sigma _y^2} \], and \[{\mathop{\rm cov}} \left( {p,q} \right) = \sigma _x^2 - \sigma _y^2\] in the expression, we get
\[ \Rightarrow \] Coefficient of correlation \[ = \dfrac{{\sigma _x^2 - \sigma _y^2}}{{\sqrt {\sigma _x^2 + \sigma _y^2} \times \sqrt {\sigma _x^2 + \sigma _y^2} }}\]
Rewriting the expression, we get
\[ \Rightarrow \] Coefficient of correlation \[ = \dfrac{{\sigma _x^2 - \sigma _y^2}}{{\sqrt {\left( {\sigma _x^2 + \sigma _y^2} \right)\left( {\sigma _x^2 + \sigma _y^2} \right)} }} = \dfrac{{\sigma _x^2 - \sigma _y^2}}{{\sqrt {{{\left( {\sigma _x^2 + \sigma _y^2} \right)}^2}} }}\]
Simplifying the expression, we get
Coefficient of correlation \[ = \dfrac{{\sigma _x^2 - \sigma _y^2}}{{\sigma _x^2 + \sigma _y^2}}\]
Therefore, the coefficient of correlation of \[x + y\] and \[x - y\] is \[\dfrac{{\sigma _x^2 - \sigma _y^2}}{{\sigma _x^2 + \sigma _y^2}}\].
Thus, the correct option is option (c).
Note: We used the coefficient of correlation in the solution. The correlation coefficient is donated by the greek letter ‘rho’, that is \[\rho \]. Here we have found out the variance and standard deviation and substituted in the formula of the coefficient of correlation. Standard deviation measures the spread of a statistical data and it is denoted by \[\sigma \]. The standard deviation can never be negative and therefore its smallest value is 0. Variance measures how much each data of a set from its average value. It is always positive and it is denoted by \[{\sigma ^2}\].
Formula Used:
We will use the following formulas:
1.The covariance between two variates \[x\] and \[y\] is given by the formula \[{\mathop{\rm cov}} \left( {x,y} \right) = E\left( {x - \overline x } \right)\left( {y - \overline y } \right)\], where \[\overline x \] is the mean of the variate \[x\], and \[\overline y \] is the mean of the variate \[y\].
2.The product of the sum and difference of two numbers is given by the algebraic identity \[\left( {a - b} \right)\left( {a + b} \right) = {a^2} - {b^2}\].
3.The variance of a variate \[z\] is given by the formula \[E{\left( {z - \overline z } \right)^2}\].
Complete step-by-step answer:
Let \[p = x + y\] and \[q = x - y\].
We know that if three variates are such that \[z = x + y\], then \[\overline z = \overline x + \overline y \].
Therefore, since \[p = x + y\] and \[q = x - y\], we get
\[\overline p = \overline x + \overline y \] and \[\overline q = \overline x - \overline y \]
The covariance between two variates \[x\] and \[y\] is given by the formula \[{\mathop{\rm cov}} \left( {x,y} \right) = E\left( {x - \overline x } \right)\left( {y - \overline y } \right)\], where \[\overline x \] is the mean of the variate \[x\], and \[\overline y \] is the mean of the variate \[y\].
Therefore, we get
\[ \Rightarrow {\mathop{\rm cov}} \left( {p,q} \right) = E\left( {p - \overline p } \right)\left( {q - \overline q } \right)\]
Substituting \[p = x + y\], \[q = x - y\], \[\overline p = \overline x + \overline y \], and \[\overline q = \overline x - \overline y \] in the expression, we get
\[ \Rightarrow {\mathop{\rm cov}} \left( {p,q} \right) = E\left[ {\left( {x + y} \right) - \left( {\overline x + \overline y } \right)} \right]\left[ {\left( {x - y} \right) - \left( {\overline x - \overline y } \right)} \right]\]
Rewriting the expressions, we get
\[\begin{array}{l} \Rightarrow {\mathop{\rm cov}} \left( {p,q} \right) = E\left[ {x + y - \overline x - \overline y } \right]\left[ {x - y - \overline x + \overline y } \right]\\ \Rightarrow {\mathop{\rm cov}} \left( {p,q} \right) = E\left[ {\left( {x - \overline x } \right) + \left( {y - \overline y } \right)} \right]\left[ {\left( {x - \overline x } \right) - \left( {y - \overline y } \right)} \right]\end{array}\]
The product of the sum and difference of two numbers is given by the algebraic identity \[\left( {a - b} \right)\left( {a + b} \right) = {a^2} - {b^2}\].
Therefore, the equation becomes
\[ \Rightarrow {\mathop{\rm cov}} \left( {p,q} \right) = E\left[ {{{\left( {x - \overline x } \right)}^2} - {{\left( {y - \overline y } \right)}^2}} \right]\]
The expression \[E\left( {x + y} \right)\] can be written as \[E\left( x \right) + E\left( y \right)\].
Therefore, we get
\[ \Rightarrow {\mathop{\rm cov}} \left( {p,q} \right) = E{\left( {x - \overline x } \right)^2} - E{\left( {y - \overline y } \right)^2}\]
The variance of a variate \[z\] is given by the formula \[E{\left( {z - \overline z } \right)^2}\].
Therefore, we get
Variance of \[x\] \[ = E{\left( {x - \overline x } \right)^2}\]
Variance of \[y\] \[ = E{\left( {y - \overline y } \right)^2}\]
It is given that the two variates \[x\] and \[y\] have standard deviations \[{\sigma _x}\] and \[{\sigma _y}\] respectively.
Variance is the square of the standard deviation.
Therefore, we get the equations
\[\sigma _x^2 = E{\left( {x - \overline x } \right)^2}\] and \[\sigma _y^2 = E{\left( {y - \overline y } \right)^2}\]
Substituting \[\sigma _x^2 = E{\left( {x - \overline x } \right)^2}\] and \[\sigma _y^2 = E{\left( {y - \overline y } \right)^2}\] in the equation \[{\mathop{\rm cov}} \left( {p,q} \right) = E{\left( {x - \overline x } \right)^2} - E{\left( {y - \overline y } \right)^2}\], we get
\[ \Rightarrow {\mathop{\rm cov}} \left( {p,q} \right) = \sigma _x^2 - \sigma _y^2\]
Now, we will find the variance of the variates \[p = x + y\] and \[q = x - y\].
The variance of a variate \[z\] is given by the formula \[E{\left( {z - \overline z } \right)^2}\].
Therefore, we get
\[ \Rightarrow \] Variance of \[p\] \[ = E{\left( {p - \overline p } \right)^2}\]
\[ \Rightarrow \] Variance of \[q\] \[ = E{\left( {q - \overline q } \right)^2}\]
Substituting \[p = x + y\], \[q = x - y\], \[\overline p = \overline x + \overline y \], and \[\overline q = \overline x - \overline y \] in the expressions, we get
\[ \Rightarrow \] Variance of \[p\] \[ = E{\left[ {\left( {x + y} \right) - \left( {\overline x + \overline y } \right)} \right]^2}\]
\[ \Rightarrow \] Variance of \[q\] \[ = E{\left[ {\left( {x - y} \right) - \left( {\overline x - \overline y } \right)} \right]^2}\]
Rewriting the expressions, we get
\[ \Rightarrow \]Variance of \[p\] \[ = E{\left[ {\left( {x - \overline x } \right) + \left( {y - \overline y } \right)} \right]^2}\]
\[ \Rightarrow \]Variance of \[q\] \[ = E{\left[ {\left( {x - \overline x } \right) - \left( {y - \overline y } \right)} \right]^2}\]
Expanding the terms using algebraic identity, \[{\left( {a + b} \right)^2} = {a^2} + {b^2} + 2ab\], we get
\[ \Rightarrow \] Variance of \[p\] \[ = E{\left[ {\left( {x - \overline x } \right)} \right]^2} + E{\left[ {\left( {y - \overline y } \right)} \right]^2} + 2E\left[ {\left( {x - \overline x } \right)\left( {y - \overline y } \right)} \right]\]
\[ \Rightarrow \]Variance of \[q\] \[ = E{\left[ {\left( {x - \overline x } \right)} \right]^2} + E{\left[ {\left( {y - \overline y } \right)} \right]^2} - 2E\left[ {\left( {x - \overline x } \right)\left( {y - \overline y } \right)} \right]\]
Since the two variates \[x\] and \[y\] are uncorrelated, we get \[E\left[ {\left( {x - \overline x } \right)\left( {y - \overline y } \right)} \right] = 0\].
Therefore, the equations become
\[ \Rightarrow \]Variance of \[p\] \[ = E{\left[ {\left( {x - \overline x } \right)} \right]^2} + E{\left[ {\left( {y - \overline y } \right)} \right]^2}\]
\[ \Rightarrow \] Variance of \[q\] \[ = E{\left[ {\left( {x - \overline x } \right)} \right]^2} + E{\left[ {\left( {y - \overline y } \right)} \right]^2}\]
Thus, we get
\[ \Rightarrow \] Variance of \[p\] \[ = \sigma _x^2 + \sigma _y^2\]
\[ \Rightarrow \] Variance of \[q\] \[ = \sigma _x^2 + \sigma _y^2\]
Taking the square roots on both the sides, we get the standard deviations as
\[ \Rightarrow \] Standard deviation of \[p\] \[ = \sqrt {\sigma _x^2 + \sigma _y^2} \]
\[ \Rightarrow \] Standard deviation of \[q\] \[ = \sqrt {\sigma _x^2 + \sigma _y^2} \]
Finally, we will find the coefficient of correlation of the two variates \[p = x + y\] and \[q = x - y\].
Therefore, we get
Coefficient of correlation \[ = \dfrac{{{\mathop{\rm cov}} \left( {p,q} \right)}}{{s.d.\left( p \right)s.d.\left( q \right)}}\]
Substituting \[s.d.\left( p \right) = \sqrt {\sigma _x^2 + \sigma _y^2} \], \[s.d.\left( q \right) = \sqrt {\sigma _x^2 + \sigma _y^2} \], and \[{\mathop{\rm cov}} \left( {p,q} \right) = \sigma _x^2 - \sigma _y^2\] in the expression, we get
\[ \Rightarrow \] Coefficient of correlation \[ = \dfrac{{\sigma _x^2 - \sigma _y^2}}{{\sqrt {\sigma _x^2 + \sigma _y^2} \times \sqrt {\sigma _x^2 + \sigma _y^2} }}\]
Rewriting the expression, we get
\[ \Rightarrow \] Coefficient of correlation \[ = \dfrac{{\sigma _x^2 - \sigma _y^2}}{{\sqrt {\left( {\sigma _x^2 + \sigma _y^2} \right)\left( {\sigma _x^2 + \sigma _y^2} \right)} }} = \dfrac{{\sigma _x^2 - \sigma _y^2}}{{\sqrt {{{\left( {\sigma _x^2 + \sigma _y^2} \right)}^2}} }}\]
Simplifying the expression, we get
Coefficient of correlation \[ = \dfrac{{\sigma _x^2 - \sigma _y^2}}{{\sigma _x^2 + \sigma _y^2}}\]
Therefore, the coefficient of correlation of \[x + y\] and \[x - y\] is \[\dfrac{{\sigma _x^2 - \sigma _y^2}}{{\sigma _x^2 + \sigma _y^2}}\].
Thus, the correct option is option (c).
Note: We used the coefficient of correlation in the solution. The correlation coefficient is donated by the greek letter ‘rho’, that is \[\rho \]. Here we have found out the variance and standard deviation and substituted in the formula of the coefficient of correlation. Standard deviation measures the spread of a statistical data and it is denoted by \[\sigma \]. The standard deviation can never be negative and therefore its smallest value is 0. Variance measures how much each data of a set from its average value. It is always positive and it is denoted by \[{\sigma ^2}\].
Recently Updated Pages
Fill in the blanks with suitable prepositions Break class 10 english CBSE
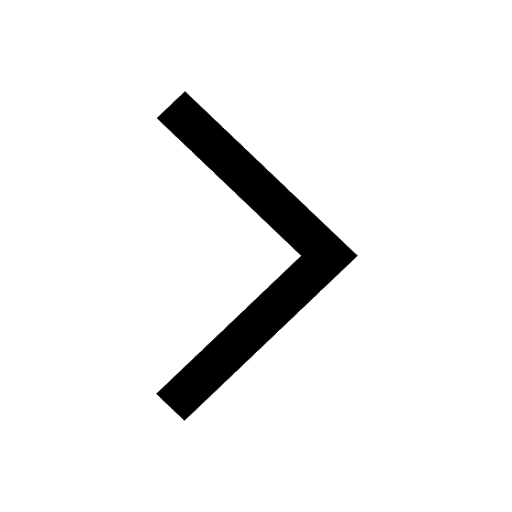
Fill in the blanks with suitable articles Tribune is class 10 english CBSE
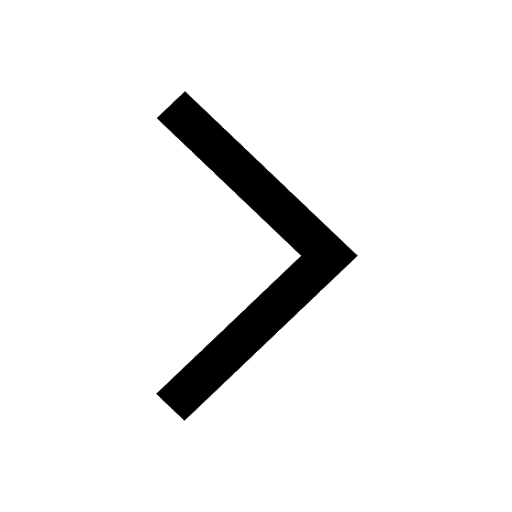
Rearrange the following words and phrases to form a class 10 english CBSE
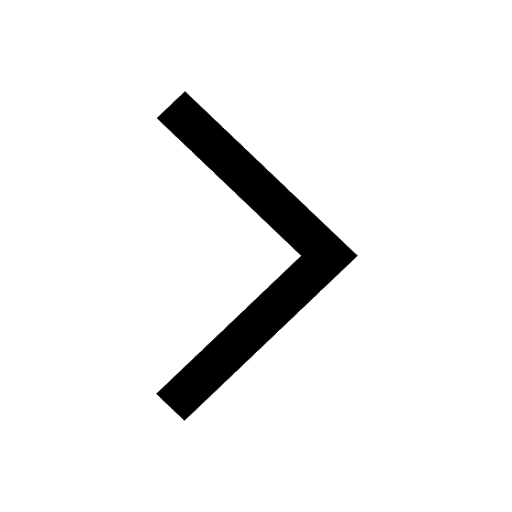
Select the opposite of the given word Permit aGive class 10 english CBSE
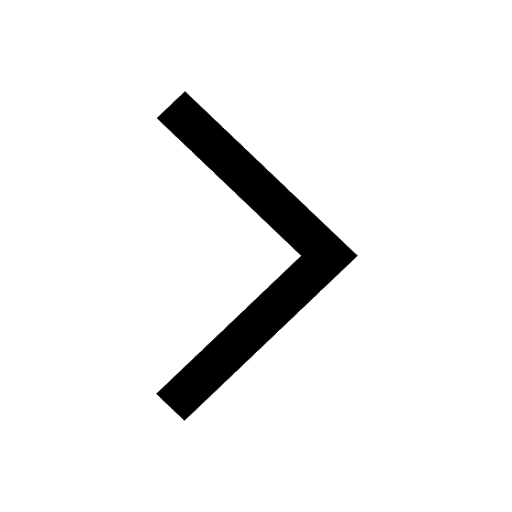
Fill in the blank with the most appropriate option class 10 english CBSE
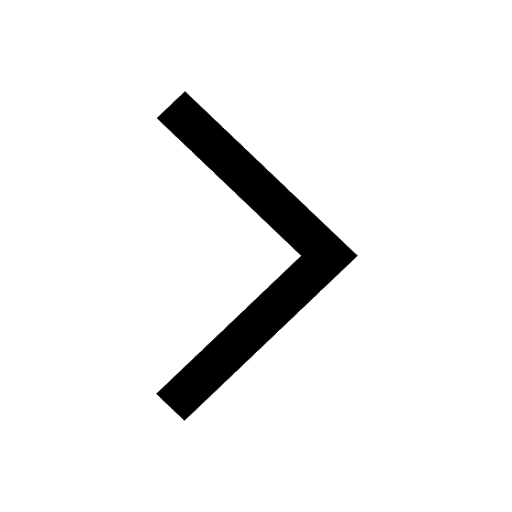
Some places have oneline notices Which option is a class 10 english CBSE
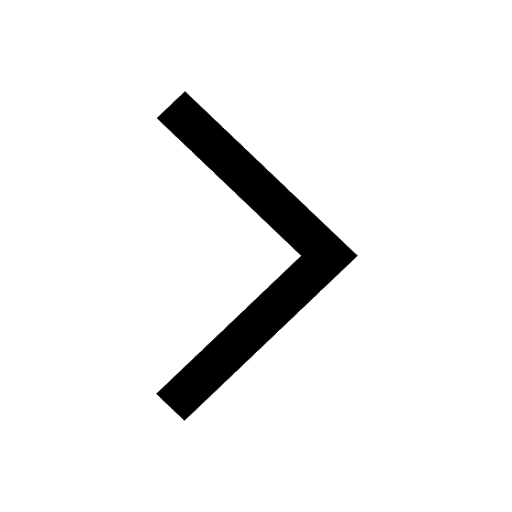
Trending doubts
Fill the blanks with the suitable prepositions 1 The class 9 english CBSE
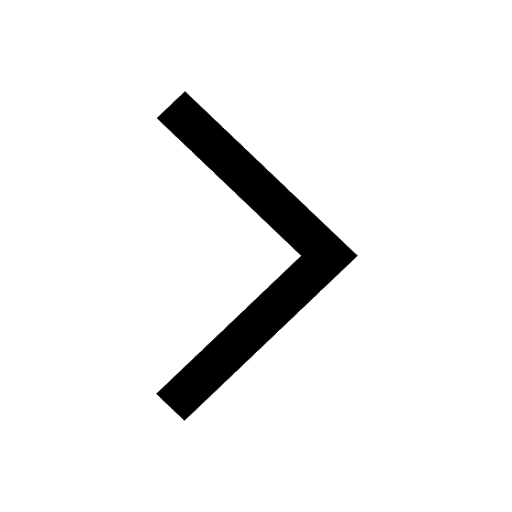
How do you graph the function fx 4x class 9 maths CBSE
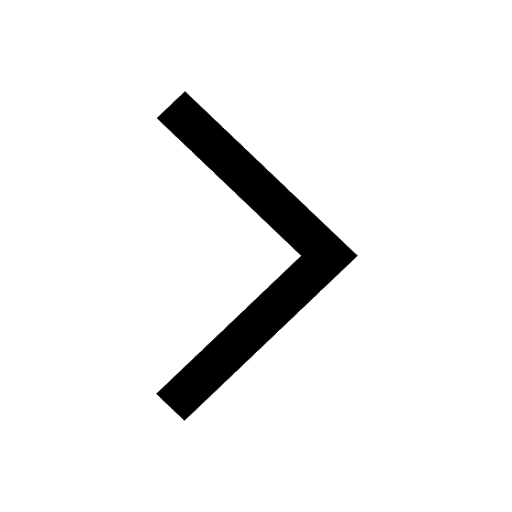
When was Karauli Praja Mandal established 11934 21936 class 10 social science CBSE
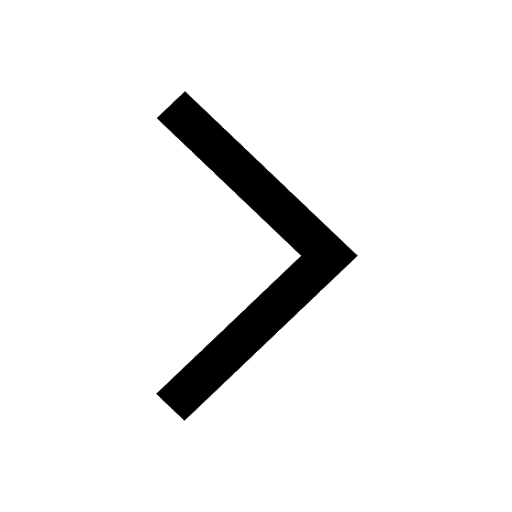
Which are the Top 10 Largest Countries of the World?
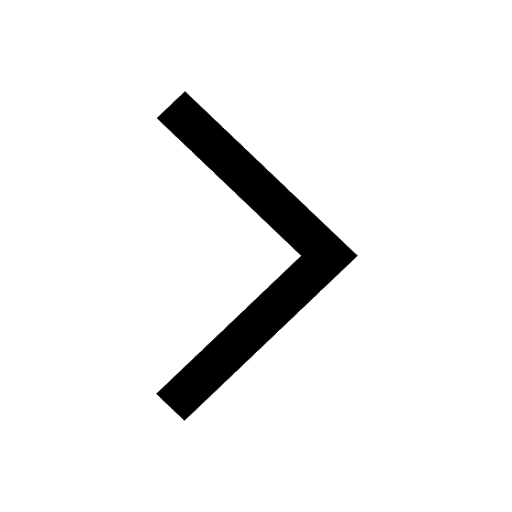
What is the definite integral of zero a constant b class 12 maths CBSE
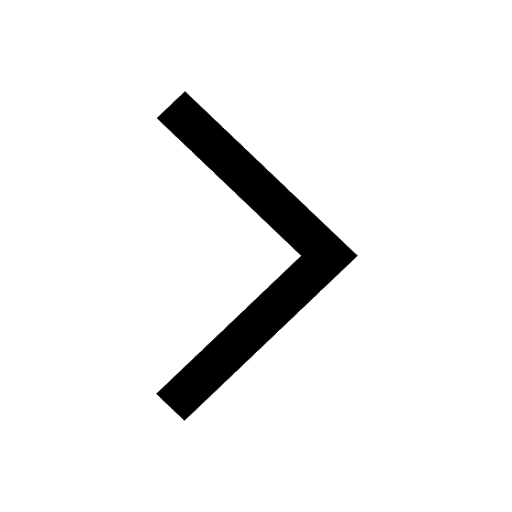
Why is steel more elastic than rubber class 11 physics CBSE
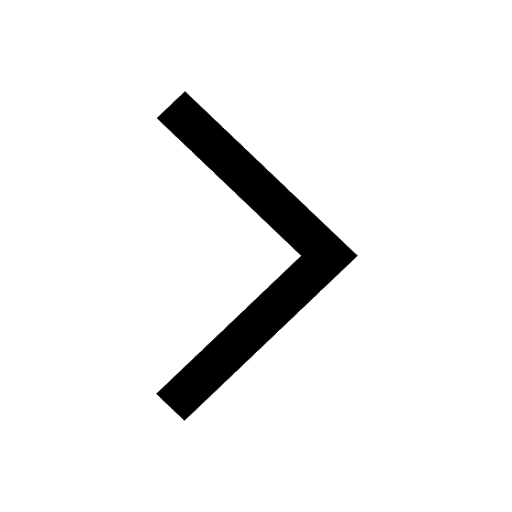
Distinguish between the following Ferrous and nonferrous class 9 social science CBSE
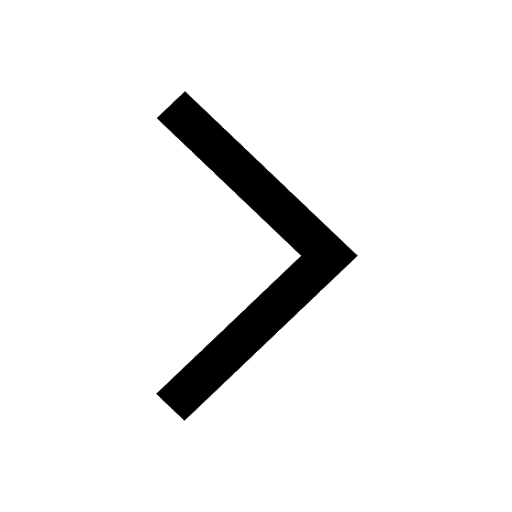
The Equation xxx + 2 is Satisfied when x is Equal to Class 10 Maths
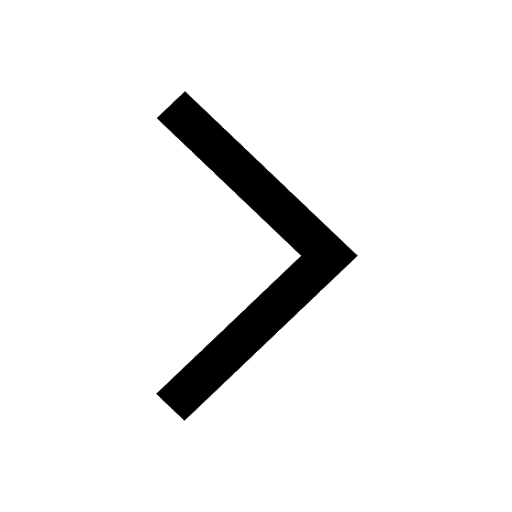
Differentiate between homogeneous and heterogeneous class 12 chemistry CBSE
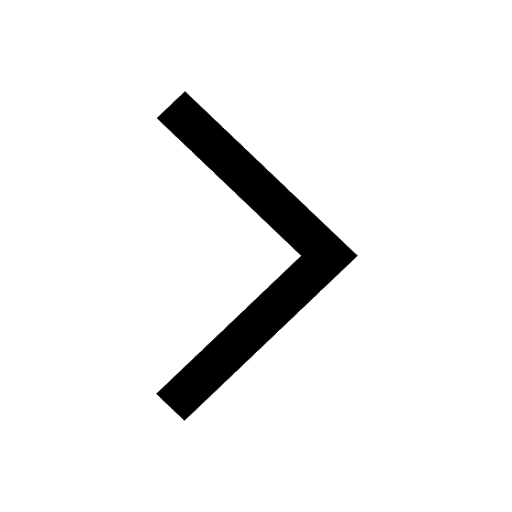