

Karl Pearson Coefficient of Correlation – A Statistical Study
The study of Karl Pearson Coefficient is an inevitable part of Statistics. Statistics is majorly dependent on Karl Pearson Coefficient Correlation method. The Karl Pearson coefficient is defined as a linear correlation that falls in the numeric range of -1 to +1.
This is a quantitative method that offers the numeric value to form the intensity of the linear relationship between the X and Y variable. But is it really useful for any economic calculation? Let, us find and delve into this topic to get more detailed information on the subject matter – Karl Pearson Coefficient of Correlation.
What do You mean by Correlation Coefficient?
Before delving into details about Karl Pearson Coefficient of Correlation, it is vital to brush up on fundamental concepts about correlation and its coefficient in general.
The correlation coefficient can be defined as a measure of the relationship between two quantitative or qualitative variables, i.e., X and Y. It serves as a statistical tool that helps to analyze and in turn, measure the degree of the linear relationship between the variables.
For example, a change in the monthly income (X) of a person leads to a change in their monthly expenditure (Y). With the help of correlation, you can measure the degree up to which such a change can impact the other variables.
Types of Correlation Coefficient
Depending on the direction of the relationship between variables, correlation can be of three types, namely –
Positive Correlation (0 to +1)
Negative Correlation (0 to -1)
Zero Correlation (0)
Positive Correlation (0 to +1)
In this case, the direction of change between X and Y is the same. For instance, an increase in the duration of a workout leads to an increase in the number of calories one burns.
Negative Correlation (0 to -1)
Here, the direction of change between X and Y variables is opposite. For example, when the price of a commodity increases its demand decreases.
Zero Correlation (0)
There is no relationship between the variables in this case. For instance, an increase in height has no impact on one’s intelligence.
Now that we have refreshed our memory of these basics, let’s move on to Karl Pearson Coefficient of Correlation.
What is Karl Pearson’s Coefficient of Correlation?
This method is also known as the Product Moment Correlation Coefficient and was developed by Karl Pearson. It is one of the three most potent and extensively used methods to measure the level of correlation, besides the Scatter Diagram and Spearman’s Rank Correlation.
The Karl Pearson correlation coefficient method is quantitative and offers numerical value to establish the intensity of the linear relationship between X and Y. Such a coefficient correlation is represented as ‘r’.
The Karl Pearson Coefficient of Correlation formula is expressed as
r = \[\frac{n\left ( \sum xy \right )-\left ( \sum x \right )\left ( \sum y \right )}{\sqrt{\left [ n\sum x^{2}-\left (\sum x \right )^{2} \right ]\left [ n\sum y^{2}-\left (\sum y \right )^{2} \right ]}}\]
In this formula,
\[X-\bar{X}\]
is mean of the X variable.
\[Y-\bar{Y}\]
is the mean of the Y variable.
What Methods are Used to Calculate Karl Pearson’s Coefficient of Correlation?
The Karl Pearson coefficient can be obtained using various methods, which are mentioned below.
Actual Mean Method Which is Expressed as
Actual Mean Method Which is Expressed as -
r = \[\frac{\sum \left ( X-\bar{X} \right )\left ( Y-\bar{Y} \right )}{\sqrt{\sum \left ( X-\bar{X} \right )^{2}\sqrt{\left ( Y-\bar{Y} \right )^{2}}}}\]
Where, \[\bar{X}\] = mean of X variable
\[\bar{Y}\] = mean of Y variable
In this Karl Pearson formula,
x = \[X-\bar{X}\]
y = \[X-\bar{Y}\]
Assumed Mean Method Which is Expressed as
Assume Mean Method
d\[_{x}\] = X - A
d\[_{y}\] = Y - A
r = \[\frac{N\sum d_{x}d_{y}-\left ( \sum d_{x} \right )\left ( \sum d_{y} \right )}{\sqrt{N\sum d_{x}^{2}-\left ( \sum d_{x} \right )^{2}}-\sqrt{N\sum d_{y}^{2}-\left ( \sum d_{y} \right )^{2}}}\]
In this Karl Pearson Correlation formula,
dx = x-series’ deviation from assumed mean, wherein (X - A)
dy = Y-series’ deviation from assumed mean = ( Y - A)
Σdx.dy implies summation of multiple dx and dy.
Σdx2 is the summation of the square of dx.
Σdy2 is the summation of the square of dy.
Σdx is the summation of X-series' deviation.
Σdy is a summation of the Y-series.
N is the number of observations in pairs.
Step Deviation Method Which is Expressed as
r = \[\frac{dX'dY'-\frac{\sum d'X\sum dY'}{N}}{\sqrt{\left ( \sum dx^{1} \right )^{2}}-\frac{\left (\sum dx^{1} \right )^{2}}{N}.\left ( \sum dy' \right )^{2}\frac{\left ( \sum dy' \right )^{2}}{N}}\]
In this particular Karl Pearson Method,
dx′=dxC1dx′=dxC1
dy′=dyC2dy′=dyC2
C1 = Common factor for series -x
C2 = Common factor for series -y
dx is x-series’ deviation from the assumed mean, where (X - A)
dy is Y-series’ deviation from the assumed mean, where ( Y - A)
Σdx.dy implies summation of multiple dx and dy.
Σdx2 is the summation of the square of dx.
Σdy2 is the summation of the square of dy.
Σdx is the summation of X-series' deviation.
Σdy is the summation of the Y-series.
N is the number of observations in pairs.
Solving a Few Karl Pearson Coefficient of Correlation Questions
Task 1: Refer to the table below and find out ‘r’ with the help of the provided data. Use the Actual Mean Method to solve it.
Task 2: With the help of this table below, find out ‘r’ using Karl Pearson Coefficient of Correlation Direct Method Formula.
Pro Tip: Try to solve one or two Karl Pearson coefficient of correlation problems using all the methods to figure out which is the easiest and shortest method of the lot. However, make sure to be thorough with all the formulas of the Karl Pearson coefficient of correlation, so that you can attempt them in your exams with greater confidence.
Once you have solved the Karl Pearson Coefficient of Correlation sums, you will be able to understand the degree of relationship between discussed variables and relate it with reality better.
Overview of the Properties of the Coefficient of Correlation
Since we gained a fair idea about Pearson’s correlation of coefficient and have also become familiar with its question format, let’s learn about its properties as well.
In case you are wondering, “Why should I check out the properties of coefficient of correlation?” - Note that a clear idea about correlation coefficient will come in handy both during exam preparation and while solving Karl Pearson Coefficient of Correlation sums. It will help you retain every minute yet vital pointer about this ratio and would further prevent you from making any silly mistake.
That being said, let’s glance through these significant properties in brief –
The Correlation Coefficient (r) does not have any unit.
r with a positive value signifies that both X and Y move along the same direction.
r with a negative value indicates an inverse relation between X and Y.
X and Y are said to be not correlated if the value of r is 0.
r with a high value signifies a strong linear relationship between two variables.
r with a low value signifies a weak relationship between two variables.
Correlation between two variables is said to be perfect if the value of r is either +1 or -1.
Assumptions of Karl Pearson Coefficient Correlation
When we calculate the Karl Pearson Correlation, we are required to make a few assumptions in mind.
Following are the two main assumptions:
There is always a linear relationship between any two variables.
We are required to keep the outliers to a minimum range or remove them totally.
Outliers are data that contrasts drastically with the rest of the data. It might signify many extreme data which actually does not fit in the set. You can spot an outlier by plotting the data in a graph paper and looking for any extreme study.
Use of Karl Pearson Coefficient in Real Life
We see that the Karl Pearson Coefficient Correlation is used extensively in mathematical procedures. In the calculation of any economic problem, this gains great vitality by estimating the variables for X and Y and thereby sorting to find the intensity between them.
To logically and accurately understand the effect of one change in regard to another we can use this method. For example, a shoe manufacturer in order to understand the varied sizes of shoes he first needs to assimilate the common foot sizes, after placing them in the Karl Pearson Coefficient Correlation formula he can estimate the requirement accordingly.
Did You Know?
The correlation was developed in 1885 by Francis Galton!
Karl Pearson was actually a British statistician who was known as the leading founder of modern statistics.
It is regarded as the best method of measuring the association between two variables of interest as it is based on another popular method called covariance.
Karl Pearson’s method gets highly affected by extreme value items, so we cannot draw any immediate conclusion using this method.
FAQs on Karl Pearson’s Coefficient of Correlation
1. How to find Karl Pearson's Coefficient Correlation?
By using this formula, one can find coefficient correlation
r = \[\frac{n\left ( \sum xy \right )-\left ( \sum x \right )\left ( \sum y \right )}{\sqrt{\left [ n\sum x^{2}-\left ( \sum x \right )^{2} \right ]\left [ n\sum y^{2}-\left ( \sum y \right )^{2} \right ]}}\]
2. How to Calculate Karl Pearson's Coefficient of Correlation?
You can use Karl Pearson's shortcut method or the direct method to calculate r.
Direct Method
r\[\left ( X,Y \right )\] = \[\frac{\sum \left ( x_{i}-\bar{x} \right )\left ( y_{i}-\bar{y} \right )}{\sqrt{\left ( \sum x_{i}^{2}-n\bar{x}^{2} \right )\left ( \sum y_{i}^{2}-n\bar{y} ^{2}\right )}}\]
Shortcut Method
r\[\left ( X,Y \right )\] = \[\frac{\sum x_{i}y_{i} -n\bar{x}\bar{y} }{\sqrt{\left ( \sum x_{i}^{2}-n\bar{x}^{2} \right )\left ( \sum y_{i}^{2}-n\bar{y} ^{2}\right )}}\]
3. What is the alternative method of calculating the Coefficient of Correlation?
You can use the Covariance formula to compute the value of r. The formula of the covariance method is expressed as –
Cov(X,Y) = \[\frac{\sum \left ( X-\bar{X} \right )\left ( Y-\bar{Y} \right )}{N}=\frac{\sum xy}{N}\]
4. Give Examples of Positive and Negative Correlation.
For negative correlation (0 to -1) – As the cost of flight tickets increases, it leads to a decrease in its demand. For positive correlation (0 to +1) – An increase in temperature increases the demand for soft drinks and ice cream.
5. Give an Example for Zero Correlation.
An increase in the cost of mango and an increase in demand for shirts is not related and hence is a Karl Pearson coefficient of correlation example for zero correlation.
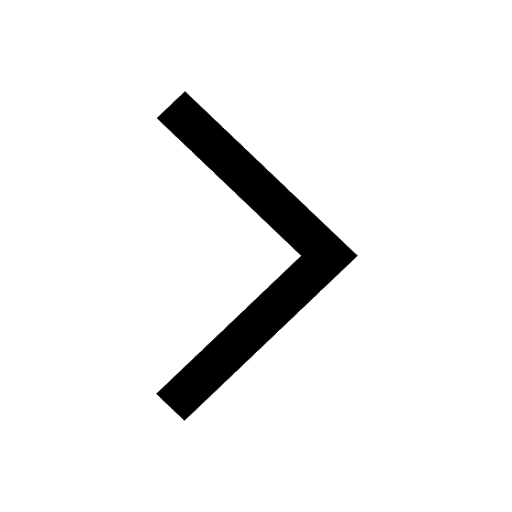
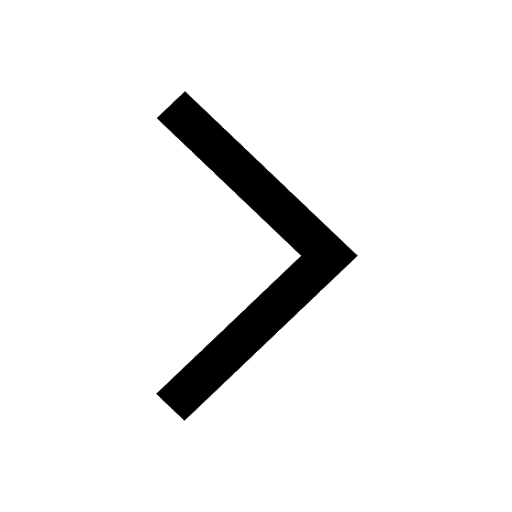
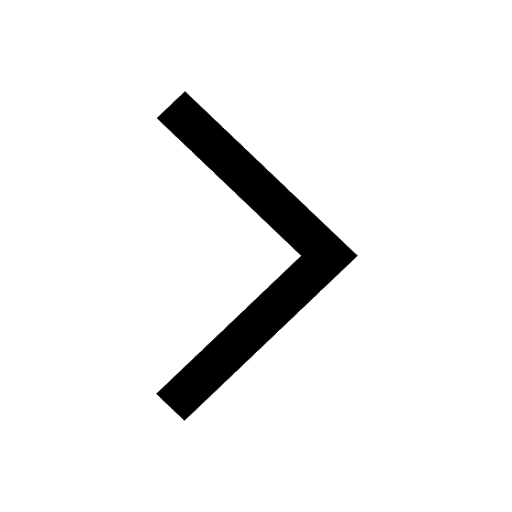
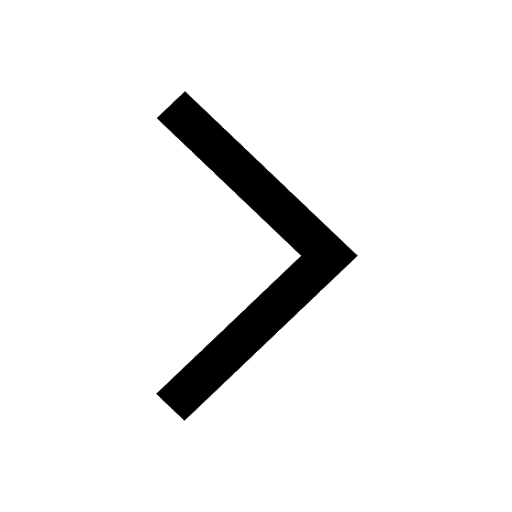
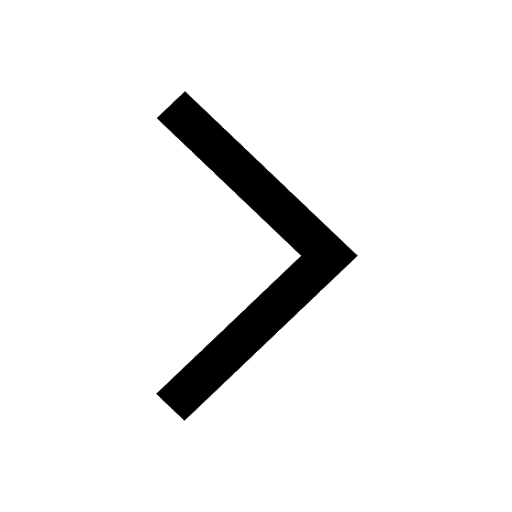
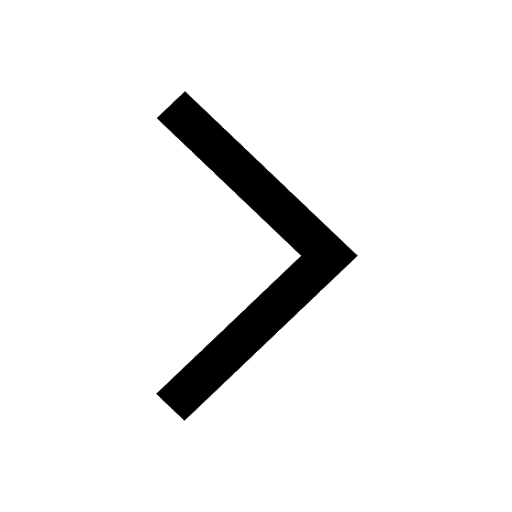