

Correlation vs Regression: Key Differences Explained
Ever come up with the question to distinguish between correlation and regression? In mathematics, understanding the relationships between variables is essential. Two key tools used to analyse these relationships are Correlation and Regression. Although they are closely related, they serve different purposes.
Correlation measures the strength and direction of a relationship between two variables, providing a number between -1 and 1 to represent how closely they move together. Regression, on the other hand, goes a step further by creating an equation to predict the value of one variable based on the other.
In this article, we’ll explore the mathematical meaning of correlation and regression, highlight their differences, and explain how they are applied in problem-solving. Let’s discuss these concepts step by step!
Difference Between Regression and Correlation with Examples
Definition of Correlation
Correlation comes from two words: 'Co' (together) and 'relation' (connection). It refers to the relationship between two variables. When a change in one variable causes a similar change in another variable, either directly or indirectly, we say the variables are correlated. If the movement in one variable doesn't lead to any specific change in the other, the variables are uncorrelated. In simple terms, correlation is a statistical method that shows how strongly two variables are related.
Correlation can be positive or negative. A positive correlation happens when two variables move in the same direction—if one increases, the other also increases, and vice versa. An example is the relationship between investment and profit.
On the contrary, if the two variables move in different directions so that an increase in one variable leads to a decline in another variable and vice versa, this situation is known as a negative correlation. For example, Product price and demand.
Given Below are the Measures of Correlation
The correlation coefficient of Karl Pearson’s Product-moment
Scatter diagram
Coefficient of concurrent deviations
Coefficient of Spearman’s rank correlation
Examples:
a. Height and Weight
You measure the heights and weights of a group of people. The correlation coefficient (r) might be 0.9, indicating a strong positive relationship. This means taller people tend to weigh more, but correlation does not explain how much more they weigh or whether height causes weight changes.
b. Study Time and Test Scores
The correlation coefficient between hours studied and test scores might be r = 0.75, suggesting a moderately strong positive relationship. More study time generally leads to higher test scores.
Definition of Regression
A statistical technique based on the average mathematical relationship between two or more variables is known as regression, to estimate the change in the metric dependent variable due to the change in one or more independent variables. It plays an important role in many human activities since it is a powerful and flexible tool that is used to forecast past, present, or future events based on past or present events. For example, The future profit of a business can be estimated on the basis of past records.
There are 3 types of regression they are,
Linear Regression
Multiple Regression
Polynomial Regression
There are two variables x and y in a simple linear regression, wherein y depends on x or say that is influenced by x. Here y is called as a variable dependent, or criterion, and x is a variable independent or predictor. The line of regression y on x is expressed as below:
Y = a + bx
where, a = constant,
b = regression coefficient,
The a and b are the two regression parameters in this equation.
Examples:
a. Predicting Weight Based on Height
Using height as the independent variable, regression might give the equation:
Weight = 30 + 2.5 × (Height in Inches)
If someone's height is 70 inches, you can predict their weight:
Weight = 30 + 2.5 × 70 = 205 lbs.
b. Predicting Test Scores
Using study hours as the independent variable, regression might give the equation:
Test Score = 40 + 5 × (Hours Studied)
If a student studies for 6 hours, the predicted score is:
Test Score = 40 + 5 × 6 =70
10 Difference Between Correlation and Regression
Correlation and Regression Difference - They are Not the Same Thing
Here’s the difference between correlation and regression analysis. To sum up, there are four key aspects that differ from those terms.
Correlation shows that there is a connection between two variables, but it doesn't explain how one affects the other. On the other hand, regression focuses on how one variable impacts the other.
Correlation doesn't show cause and effect, but regression does.
The correlation between x and y is the same as between y and x, but the results of regression for x and y, and y and x, are different.
Finally, one single point is a graphical representation of a correlation. Whereas one line visualizes a linear regression.
Similarities Between Correlation And Regression
After examining the differences, let’s explore how correlation and regression are alike.
If two variables have a positive correlation, their regression slope will also be positive.
When variables show a negative correlation, the regression slope will decline.
Both methods are used as statistical tools to better understand the relationship between variables.
Advantages of Correlation Analysis:
Correlation analysis helps students to get a more clear and concise summary regarding the relation between the two variables.
Advantages of Regression Analysis:
By using regression analysis one of the greatest advantages is that it allows you to take a detailed look at the data and includes an equation that can be used to predict and optimize the data set in the future.
Conclusion
In this article, we learned the key differences between correlation and regression. Correlation measures the strength and direction of a relationship between two variables, but it doesn’t imply cause and effect. Regression, however, not only shows the relationship but also provides an equation to predict one variable based on the other. While correlation is about understanding connections, regression focuses on modelling and forecasting relationships. Together, they are powerful tools for analyzing and interpreting data.
FAQs on Key Difference Between Correlation and Regression
1. What are the different types of regression according to their functionality?
Regression is a method used to model and evaluate relationships between variables, and at times how they contribute and are linked to generating a specific result together. The different types of regression according to their functionality are as follows:
1. Simple Linear Regression - This is a statistical method used to summarize and study the relationships between any two continuous variables – an independent variable and a dependent one.
2. Multiple Linear Regression - This regression type examines the linear relationship between a dependent variable and more than one independent variable that exists.
2. What are the different types of Correlation according to their character?
The three types of relation to their character are -
1. Positive Correlation - If two variables are seen moving in the same direction, whereby an increase in the value of one variable results in an increase in another, and vice versa.
2. Negative Correlation - on the other hand, when two variables are seen moving in different directions, and in a way that any increase in one variable results in a decrease in the value of the other, and vice versa.
3. Zero Correlation - If any change in one variable is not dependent on the other, then Zero Correlation is said to have the variables.
3. What are some of the key differences between Correlation and Regression that need to be noted while studying the chapter?
Some of the key Difference Between Correlation and Regression that need to be noted while studying the chapter can be provided as follows:
Correlation is a measure that is used to represent a linear relationship between two variables whereas regression is a measure used to fit the best line and estimate one variable by keeping a basis of the other variable present.
In correlation, it is observed that there is no difference between the dependent and independent variables while in regression both the variables are interdependent on each other.
Correlation helps find a numerical value that expresses the relation between different variables. While on the other hand regression is a goal to predict the values of random variables by fixing the values of determining variables.
4. Why is it necessary to learn the difference between Correlation and Regression?
Correlation and regression are some of the values that find application in statistics and these allow finding various relations and values for different variables involved. This topic is also considered to be one of the most important topics in Class 12 and hence students need to be prepared for the same. Both of the measures play an important role in varying situations and hence it is important to analyze and understand under which condition to use which measure. This also allows students to calculate the answer effectively and apply the logic learned. By learning more about the Difference Between Correlation and Regression students can apply the required measures under the required conditions.
5. How does Vedantu help students learn more about the difference between Correlation and Regression?
Vedantu is an open platform that helps the student learn more about how to use various logic and solve certain problems during both exams and real-life situations. By understanding the Difference Between Correlation and Regression students get major help for not only their Class 12 exams but also are able to discover more about the topic. Vedantu also helps students practice the topic that they have learned by solving some Vedantu sample papers for Class 12 that shows how each of the topics is applied to various questions involved. This in turn helps students to analyze a problem successfully.
6. What are the various conditions under which correlation and regressions are to be used?
Correlation and regression are two different quantities and are used under various conditions that can be given as follows:
1. Correlation: This measure is used when there is an immediate requirement for a direction to be understood. Here there will be a relation between two or more variables being involved.
2. Regression: This is a measure used when there is a requirement to optimize and explain the numerical response provided from y to x. Here it helps to understand how y can influence x by creating an approximation.
7. What is the difference between the correlation slope and the regression slope and how are they connected to each other?
The correlation measures the direction and strength of the association between two numeric values such as A and B which will always remain between the values -1 and 1. The linear equation is followed to connect the two variables in a regression analysis. Here both the measures help in understanding the degree and direction of a link between the two numeric values available. While both of these slopes are different from each other they are also dependent on each other for example if the regression slope has a negative value then the correlation slope value will also be negative. While if the regression slope value is positive then the correlation slope value is also positive.
8. What is the difference between correlation and regression in statistics?
Correlation is a measure that shows how strongly two variables are related and whether they move together in the same or opposite direction. Regression, on the other hand, goes deeper by creating an equation that explains how one variable affects the other, helping us make predictions.
9. Correlation vs Regression: Which is better for analysis?
It depends on the goal. Use correlation if you just want to measure the strength and direction of a relationship. Use regression if you want to understand how one variable influences the other or to predict future values.
10. What is the difference between correlation and regression in simple terms?
Correlation tells us how closely two things are related but doesn’t show cause-and-effect. Regression explains the relationship by creating a formula that allows us to predict one variable based on the other.
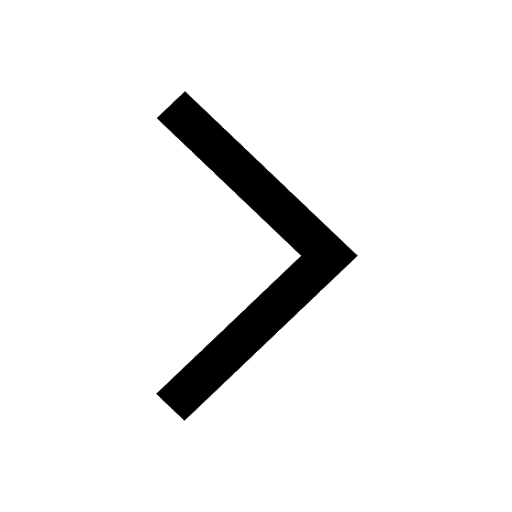
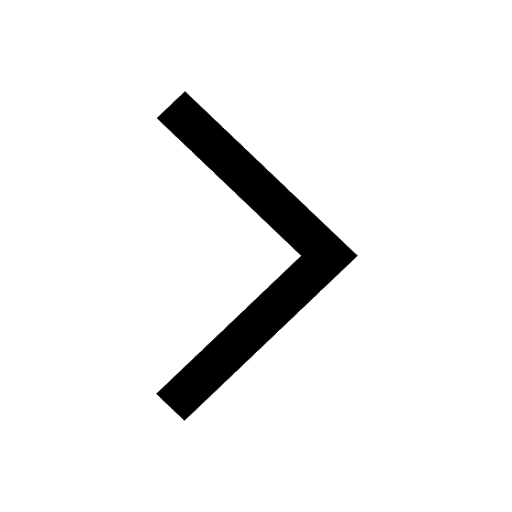
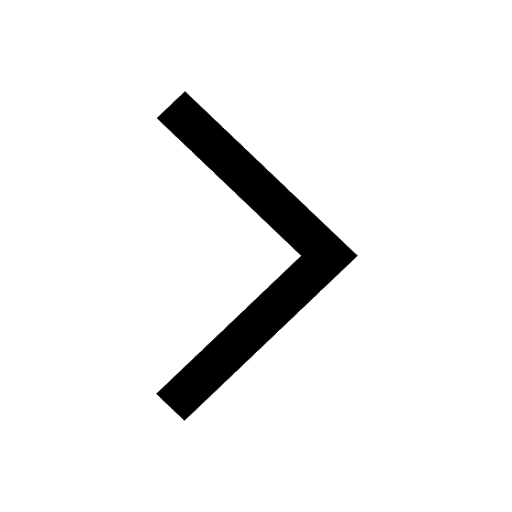
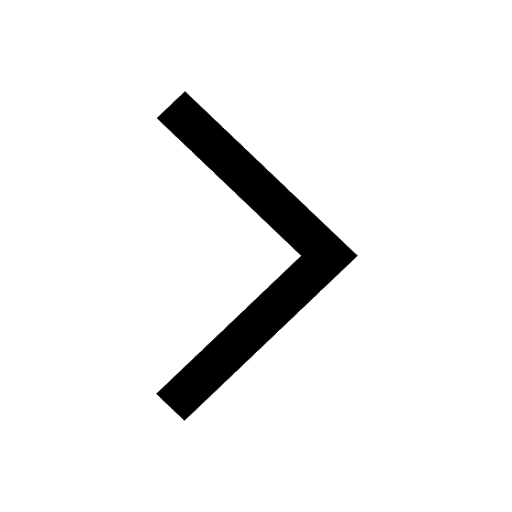
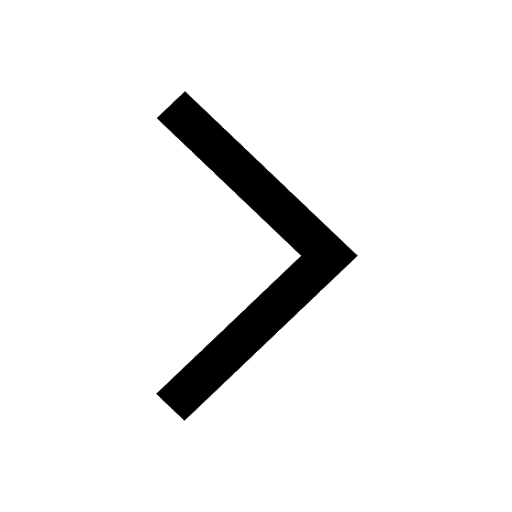
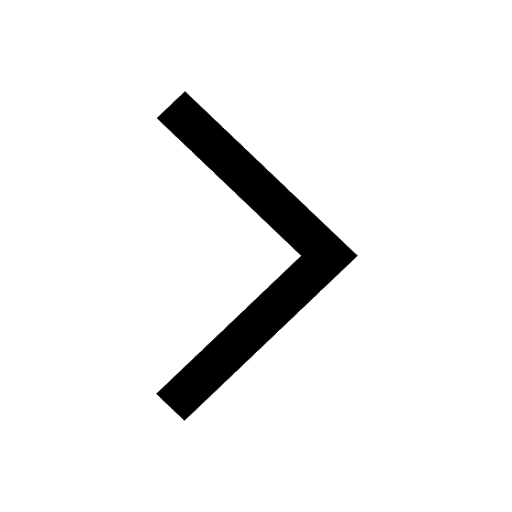
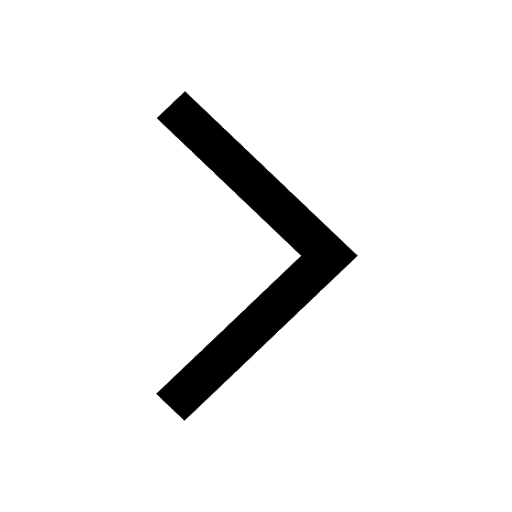
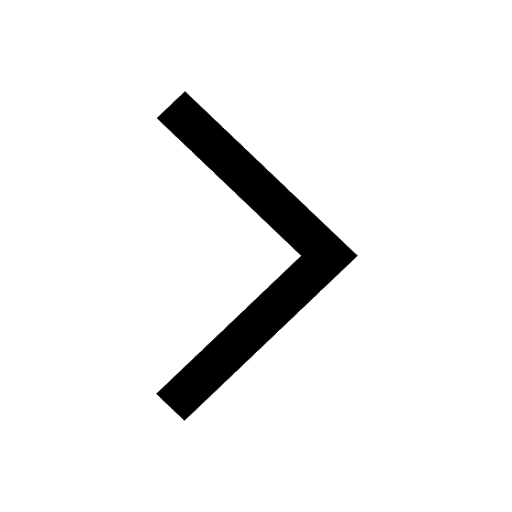
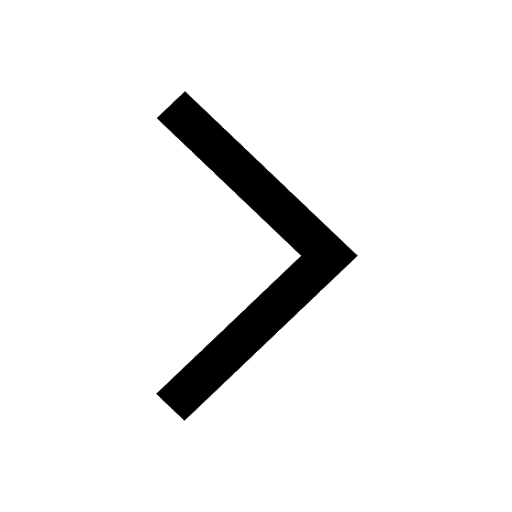
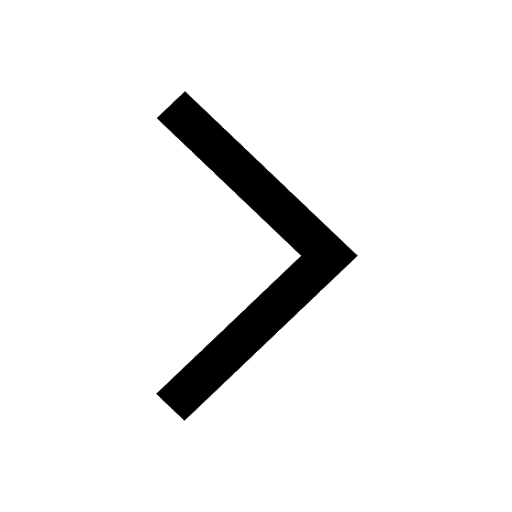
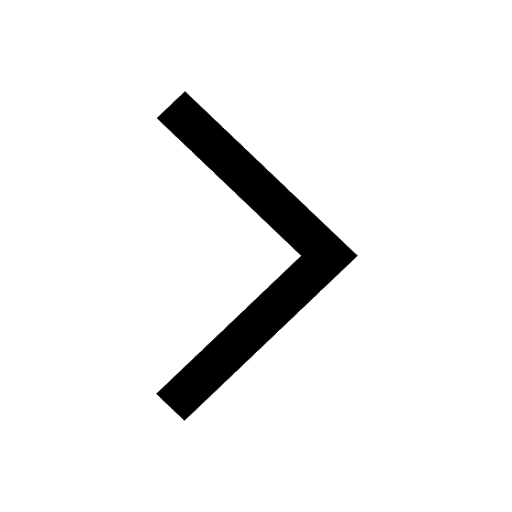
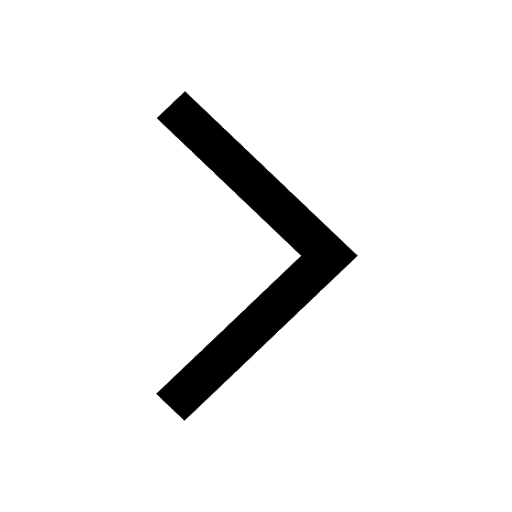
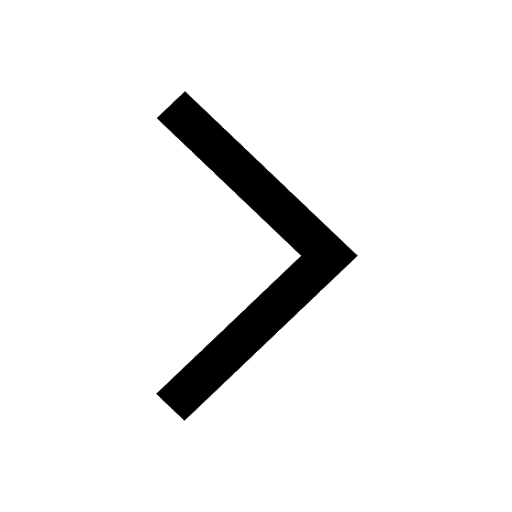
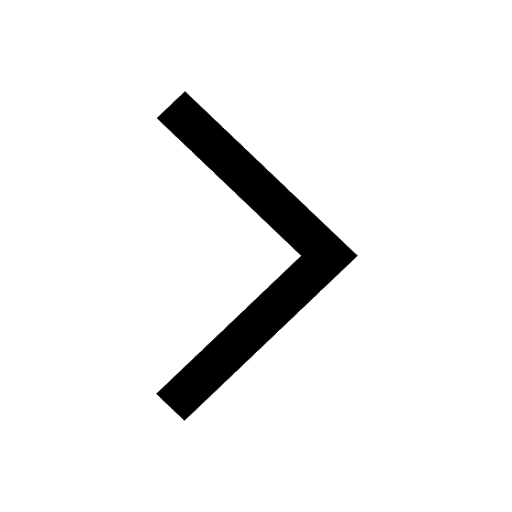
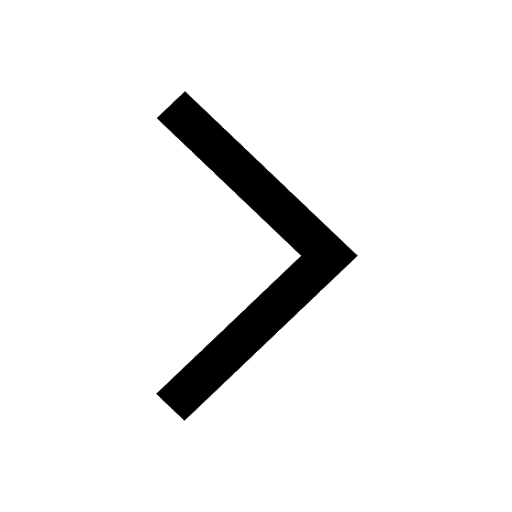
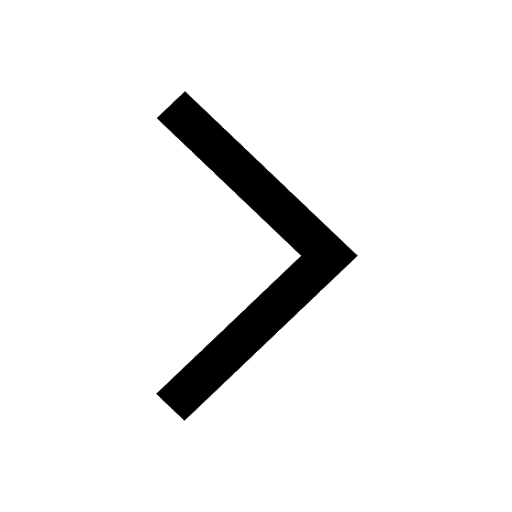
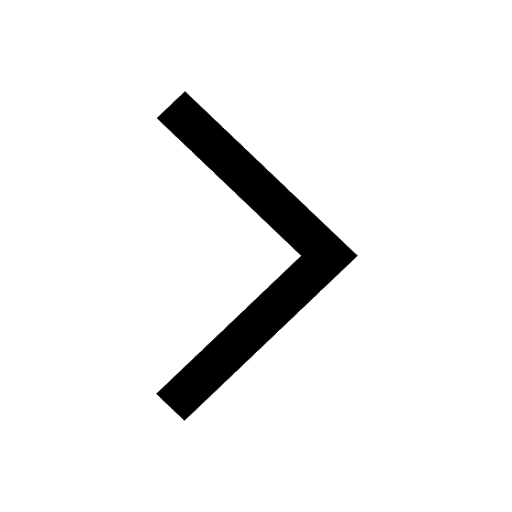
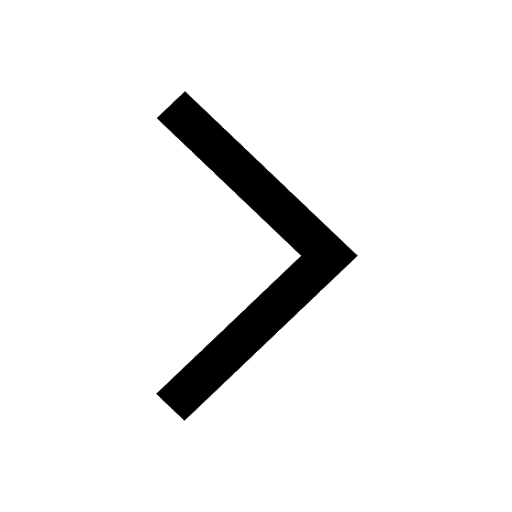