

What is Probability Density Function (PDF)?
Definition of Probability Density Function-
The probability function which is represented for the density of a continuous random variable lying between a certain range of values is known as Probability Density Function.
It is known as a probability distribution function or just a probability function. This function is referred to as a cumulative distribution function or Probability Mass Function (PMF).
Probability Distribution function (PDF) is defined for continuous random variables whereas PMF (Probability Mass Function) is defined for discrete random variables.
PMF does not work for continuous random variables because for any continuous random variable P(X=x) =0 for all x∈R , where R = real numbers.
The function defined in the form of an integral of the density of the variable density over a given range is known as PDF and it is generally denoted by f (x).
The function f(x) can be positive or non-negative at any point in the graph.
The integral of the Probability Density Function over the entire space is always equal to one.
Formula for Probability Density Function (PDF)-
The probability taken by a variable X in case of a continuous random variable on some given value x is always equal to zero. Instead of finding P(X = x), we need to calculate the probability of X lying in a given interval (a, b).
Now, let’s calculate it for P (a< X< b). We can do this by using the Probability Density Function. The Probability distribution function formula can be defined as,
P (a<X<b)= \[\int_{a}^{b}\]f(x)
The symbol f(x) is used to represent the curve. f(x) is the function that corresponds to the graph; to draw the graph of the probability distribution we use the density function f(x).
Formula Review-
Properties of Probability Density Function-
Let us assume x is the continuous random variable with f(x) as the density function, the probability distribution function should satisfy the following given conditions:
For a continuous random variable that takes any value between certain limits, let us say a and b, and we calculate it by finding the area under its curve and the x-axis, within(b) as the upper limit and the (a) as the lower limit and, then the Probability Density Function can be denoted by
P(x) = \[\int_{a}^{b}\]f(x)
It is non-negative for all the possible values, that is f(x) is greater than 0, for all values of x.
f(x) > 0
The area between the horizontal X-axis and the density curve is equal to 1, that is,
\[\int_{-∞}^{∞}\]dx = 1
Because of the property of continuous random variable, for all over the given range the density function curve is continuous which defines itself over a range of continuous values or the domain of the variable.
Types of Probability Distribution-
Probability Distributions generally come in many different shapes with various different properties, as defined by the standard deviation, mean, skewness, and kurtosis. The different Probability distributions represent different data generation processes and serve different purposes. There are different types of Probability Distribution like -
Normal Distribution
Chi square Distribution
Binomial Distribution
Poisson Distribution
Out of all the Probability Distributions, the normal distribution is the most commonly used in engineering, science, finance, and investing.
A Few Applications of Probability Density Function You Need to Know!
The applications of the probability density function are listed below:
The probability density function can be used to model the annual data of atmospheric NOx temporal concentration.
Probability Density Function is also used to model the diesel engine combustion.
In Statistics, it is used to calculate the probabilities associated with the random variables.
Probability Distributions are used in risk management.
It is also used to evaluate the probability and amount of losses.
Questions to be Solved-
Question 1) Let X be a random variable with Probability Density Function,
f(x) =
Find the constant c.
Solution) Now to find the constant c we can use \[\int_{-∞}^{∞}\]f(x)u du = 1
= \[\int_{-∞}^{∞}\]f(x)u du = 1
= \[\int_{-1}^{1}\]c\[u^{2}\]du = 1
=\[\frac{2}{3}\]c
Thus, the value of the constant is \[\frac{2}{3}\]
FAQs on Probability Density Function
Question 1) What Does PDF and CDF in Probability Mean?
Answer) PDF stands for probability density function. It is difficult to differentiate between the two. When X is a continuous random variable, then When X is a discrete random variable, then. CDF is a function whose output is generally a probability but the output of Probability Density Functions is a non negative number.
Question 2) What is the Difference Between Probability Mass Function and Density Function?
Answer) The integral of a probability density function gives the probability that a random variable falls within some given interval whereas the probability mass functions are used for discrete distributions.
Question 3) What is the Formula for Probability Density Function?
Answer) The Probability distribution function formula can be defined as,
P(a<X<b) = b∫a f(x)
The symbol f(x) is used to represent the curve; f(x) is the function that corresponds to the graph; to draw the graph of the probability distribution we use the density function f(x).
Question 4) Can Probability Density Function is Greater Than 1?
Answer) A probability density function unlike probabilities, can take on values greater than one; for example, the uniform distribution on the interval [0, 0.5] has probability density f(x) =2 for 0≤x≤0.5 and f(x) =0 elsewhere.
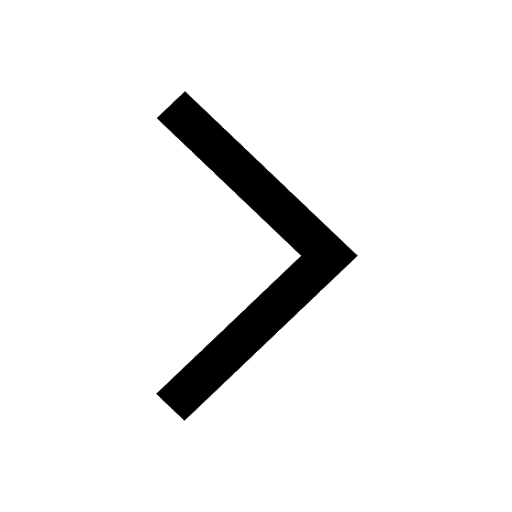
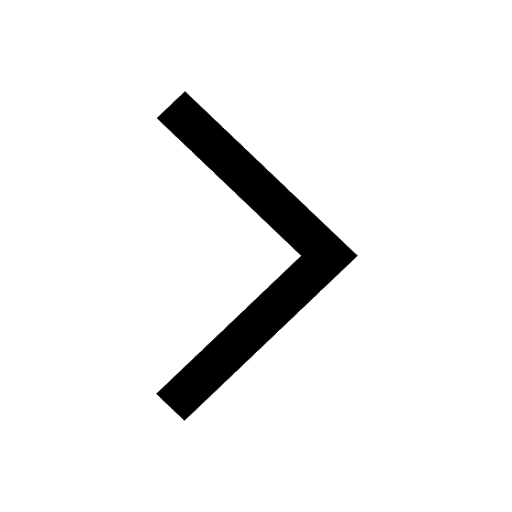
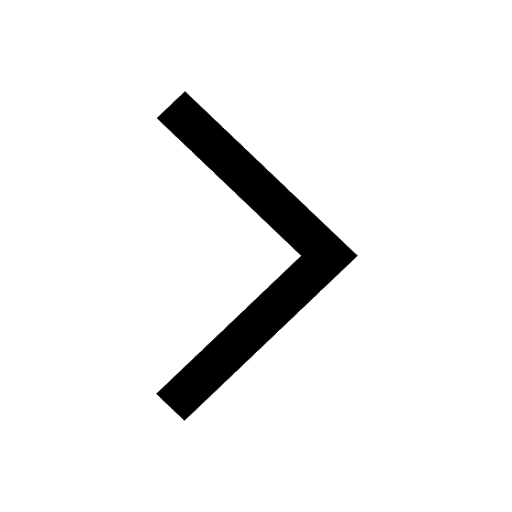
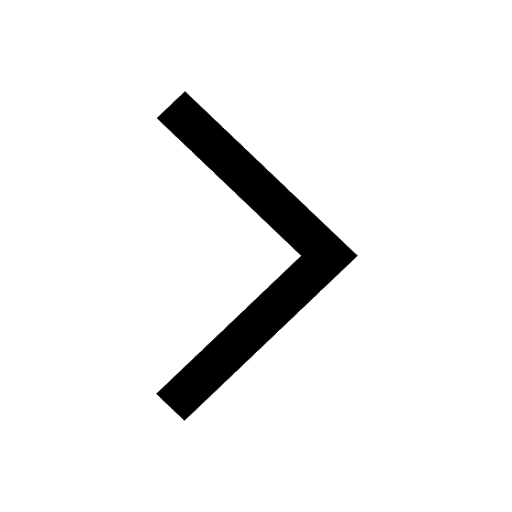
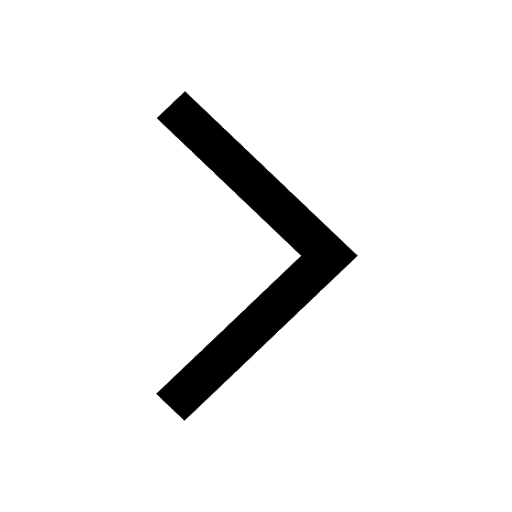
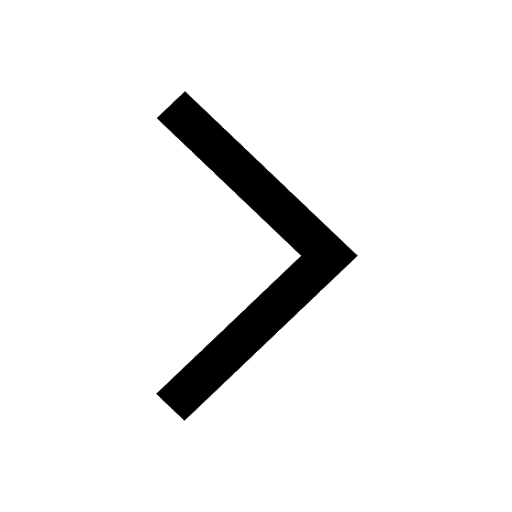
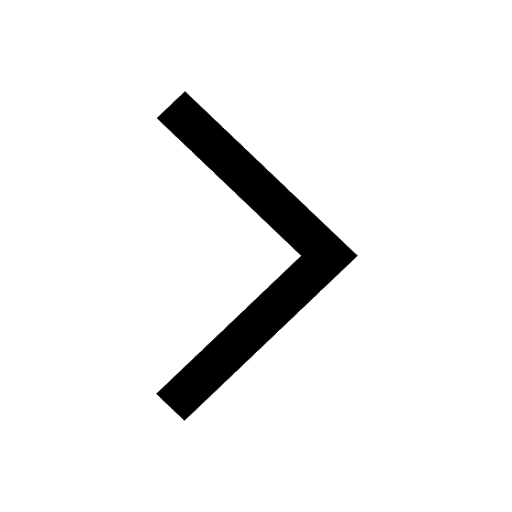
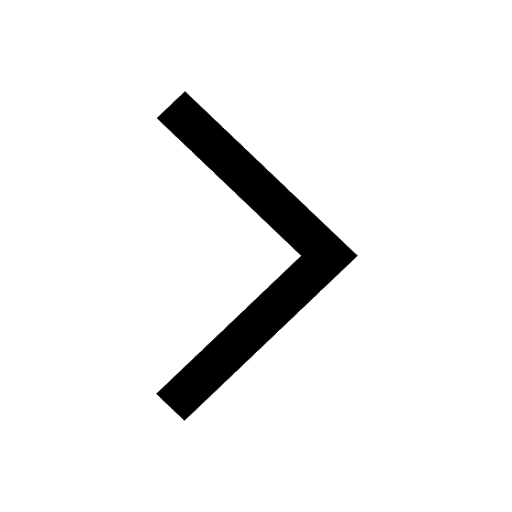
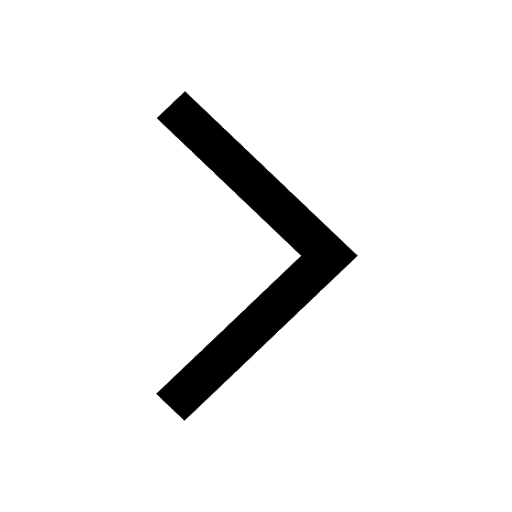
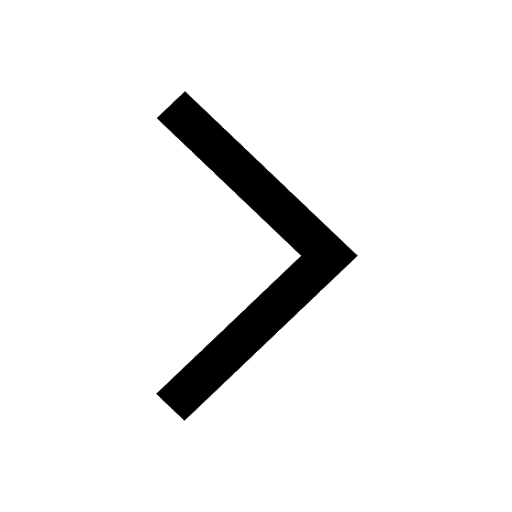
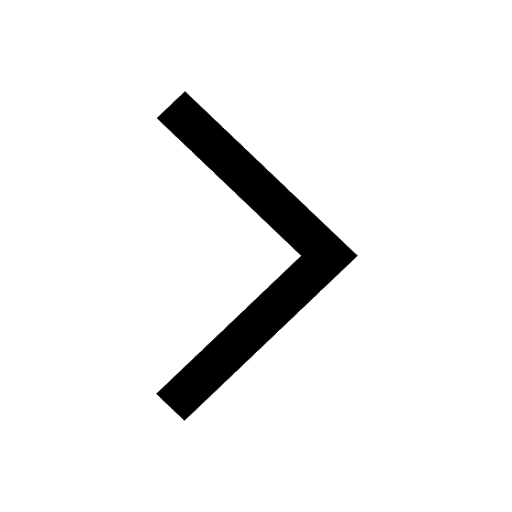
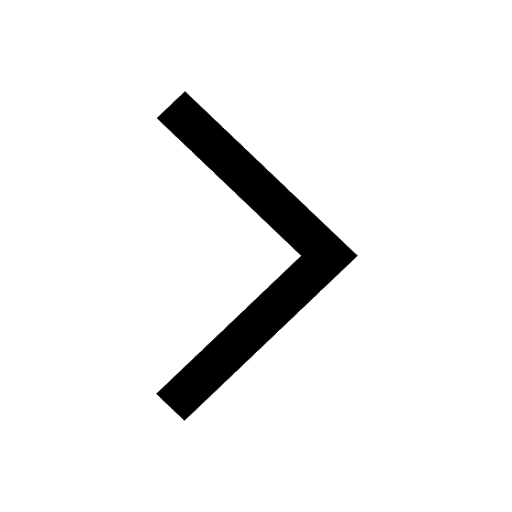
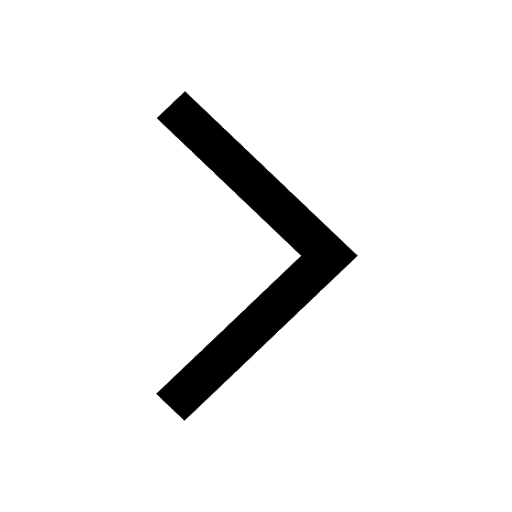
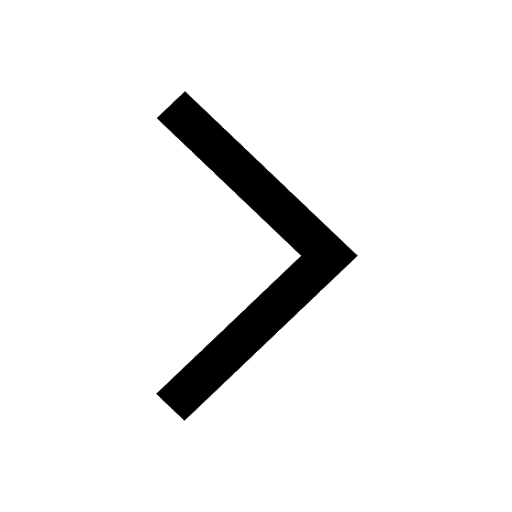
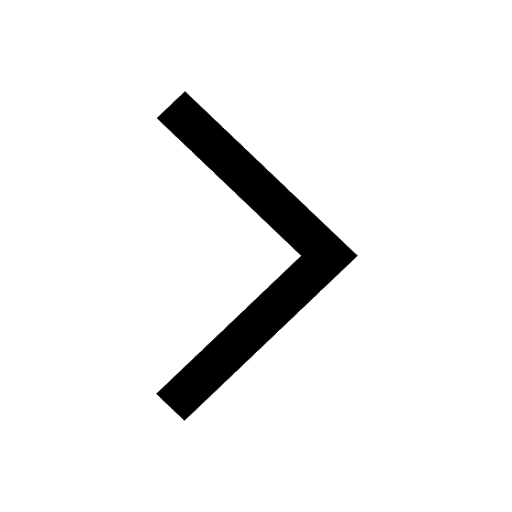
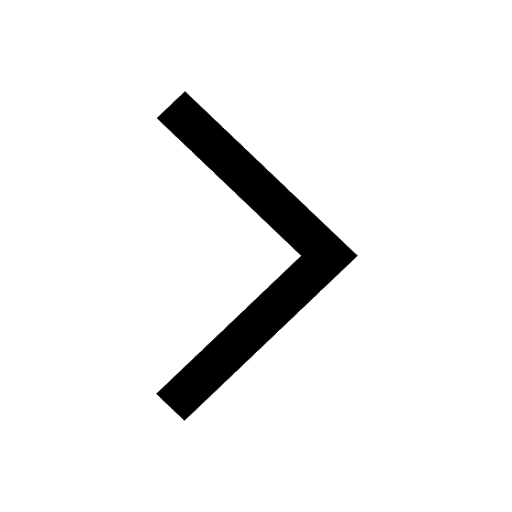
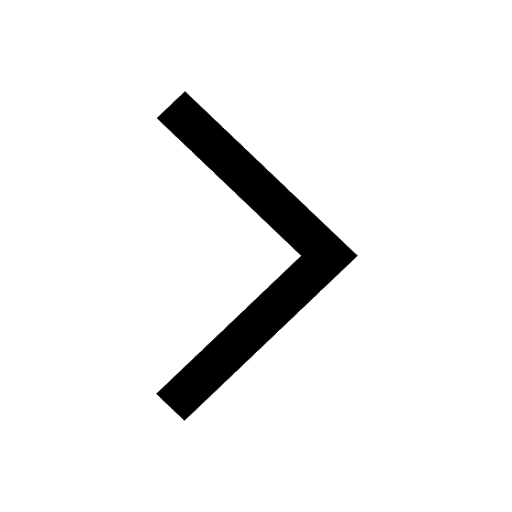
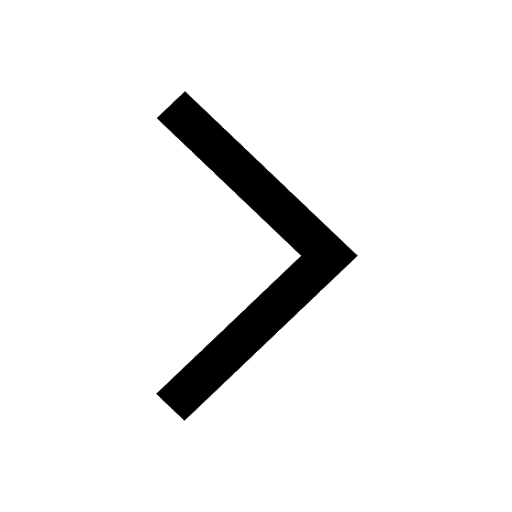