

Standard Normal
The standard normal distribution is a type of normal distribution. It appears when a normal random variable has a mean value equals zero and the value of standard deviation equals one. The mean of standard normal distribution is always equal to its median and mode.
The random variable of a standard normal curve is known as the standard score or a Z-score. It is possible to change each normal random variable X into a z score through the following standard normal distribution formula
Z = (X- μ)/σ
In the standard normal distribution formula given above,
X represents a normal random variable
μ is the mean of X,
σ is the standard deviation of X. Y
In probability theory, the normal or Gaussian distribution is the most significant continuous probability distribution.
Standard Normal Distribution Table
The std normal distribution table shows the probability of a continuous distributed random variable Z, whose mean value is equal to 0 and the value of standard deviation equal to one.The mean of standard normal distribution is always equal to its median and mode.
The normal distribution also known as Gaussian distribution is a continuous probability distribution. It is appropriate only for the positive values of Z.
The std normal distribution table is used to examine the area under the bend (f(z)) to find the probability of a particular range of distribution. The normal distribution density function f(z) is called the Bell Curve as its shape looks like a bell.
If you are looking to find the probability of a value is not exactly or more than a fixed positive z value then you can find the value with the help of a std normal distribution table. This is known as area Φ.
A std normal distribution table introduces a cumulative probability associated with a specific z-score. The rows of the std normal distribution table signify the whole number and tenths place of the z-score whereas the columns of the std normal distribution table signifies the hundredths place.The cumulative probability (from -∞ to the z-score) appears in the cell of the table.
For example, to find the cumulative probability of a z-score equal to -1.21, comparing the row of the table holding -1.2 with the column holding 0.01.The table shows that the probability that a standard normal random variable will be less than -1.21 is 0.1131;i.e. is, P(Z < -1.21) = 0.1131. The std normal distribution table is also known as a z-score table.
Std Normal Distribution Table
Area Under a Standard Normal Curve
In Mathematics, the area under the curve of the graph of an equation is determined by managing that equation's specific terms directly, such as by integrating the curve between the x-coordinates of interest. With the normal curve, you rather look up either one or two numbers in a table known as z-values and, if required you can perform a subtraction step.
The area under the standard normal curve regardless of its accurate shape, is given the value 1.0. All limited areas under the standard normal curve are thus decimal numbers between 0 and 1 and can be easily converted into percentages by multiplying them by 100.
Z-tables enable reading up to the hundredth place of the score to provide areas to four or five particular digits. This can be done by placing the tenth place on the left axis and then reading across the specific row to find the hundredth place.
This states why the proportion of the area to the left of z = -2.58 is .00494.
Area of Standard Normal Distribution
Graphically, the probability of Z, not a prerequisite “a” being Φ(a), considered from the standard normal distribution table, is represented in the following way.
[Image will be Uploaded Soon]
P(Z < –a)
As described above, the standard normal distribution table just provides the probability to values not necessarily a positive z value (i.e.,values of z on the right- hand side of the mean). So, how will you determine the probability below a negative z value (as listed below)?
[Image will be Uploaded Soon]
P(Z > a)
The probability of P(Z greater than a) is 1 – Φ(a). You can understand the reason behind this by looking at the interpretation given below
[Image will be Uploaded Soon]
As we know Φ(a) and comprehend that the total area under the standard normal curve is 1. Hence, numerically it is represented as P(Z > an) is: 1 Φ(a).
P(Z > –a)
The probability of P(Z greater than –a) is P(a), which is Φ(a). To understand this, we are required to value the symmetry of the standard normal distribution curve. We are making an effort to determine the region below:
[Image will be Uploaded Soon]
If this Particular Area is in the Region we Require:
You can observe that this is the same size area as the area we are looking for, This area under the standard normal curve can be found straight from the standard normal distribution table. It is
P(Z < a). In this way, the P(Z greater than (–a)) is P(Z lesser than a), which is Φ(a)
Normal Distribution Examples and Solutions
Here, you can see some of the normal distribution examples and solutions
1. For some laptops, the time between charging the laptop battery is normally distributed with a mean of 50 hours and a standard deviation of 15 hours. Rajesh owns one of these laptops and wants to know the probability that the time period will be between 50 and 70 hours.
Solution: Let us consider y as the random variable that indicates the time period.
Given, the mean value(μ) = 50 and standard deviation, σ = 15
We are required to find the probability that y lies between 50 and 70 or P( 50 < y < 70)
We find the probability through the standard normal distribution formula given below:
z = (X– Mean) / Standard deviation
If we consider x = 50 , then z = (50 – 50) / 15 = 0
If we consider x = 70 , then z = (70 – 50) / 15 = 1.33
P( 50< x< 70) = P( 0< z < 1.33) = [area to the left of z = 1.33] – [area to the left of z = 0]
From the standard normal distribution table we get the value, such as;
P( 0 < z < 1.33) = 0.9082 – 0.5 = 0.4082
The probability that Rajesh laptop has a time period between 50 and 70 hours is 0.4082.
2. A unit known as radar is used to measure speeds of buses on a motorway. The speed of the buses are normally distributed with a mean of 90 km/hr and a standard deviation of 10 km/hr. What is the probability that a bus sliced randomly is travelling at more than 100 km/hr?
Solution: Let y be any random variable that indicates the speed of buses.
Given- Mean(μ)= 90 and standard deviation ( σ) = 10.
We have to find the probability that y is higher than 100 or P(y > 100)
We find the probability through the standard normal distribution formula given below:
z = (X– Mean) / Standard deviation
If we take x= 100 ,then z = (100 - 90) / 10 = 1
P(y > 90) = P(z > 1) = (Total area) - (area to the left of z = 1)
= 1 - 0.8413 = 0.1587
The probability that a bus selected randomly has a speed greater than 100 km/hr is 0.1587
Quiz Time
1. If P(X ≤ 3) = 0.2 and X is Normally Distributed with Mean 5. What will be the Standard Deviation of X.
σ = 0.42
σ = −0.42
σ = 0.38
σ = 2.38
2. The Shape of the Normal Distribution Curve is
Bell Shaped
Flat
Circular
Spiked
3. The Area Under the Standard Normal Distribution Curve is?
0
1
∞
not defined
4. The Mean of the Standard Normal Distribution is Always Equal to its Median and Mode.
True
False
FAQs on Standard Normal Distribution
1. What are the Different Properties of Normal Distribution?
Answer: Some of the properties of the standard normal distribution are given below:
The shape of the normal distribution is symmetric
The normal distribution has a mound in between and tails going down to the left and right.
The mean of normal distribution is found directly in the middle of the distribution.( The mean of the population is represented by Greek symbol μ).
The mean, median and the mode of normal distribution are equal because it is symmetrical in shape.
The standard deviation is the distance from the center to the saddle point (the place from where the shape of the curve changes from an upside-down-bowl shape to a right-side-up bowl shape. (The standard deviation of the population is represented by Greek symbol σ)
Each normal distribution has distinct values of means and standards deviation which make them different from others. But,all the normal distributions have the same bell shape.
About 68% of the values fall within one standard deviation of the mean, about 95% of the values fall within one standard deviation of the mean,and about 99.7% or more falls within one standard deviation of the mean.
2. What are the Practical Applications of Standard Normal Distribution?
Answer: With the help of the standard normal distribution, you will be able to know in which subject you scored high marks and in which subject you have to put more effort due to the low marks. One you will know the subject in which you scored higher than another subject, you might think you are better or worked hard in the subject you scored high marks.
You can only say that you're better in a subject in which you scored high marks if you get a score with a certain number of standard deviations above the mean. The standard deviation helps you to know how strongly your data is clustered around the mean. It helps you to compare different distributions that have different types of data with different means.
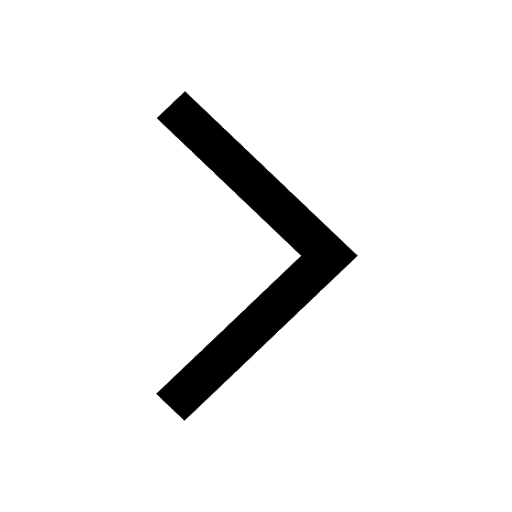
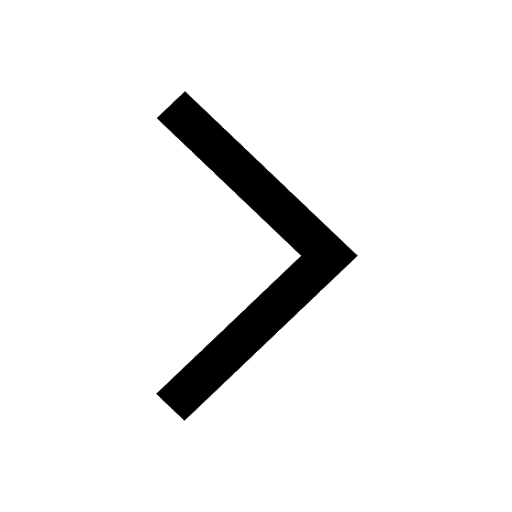
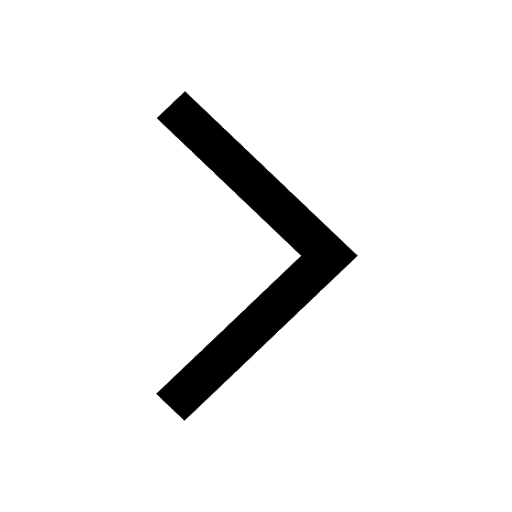
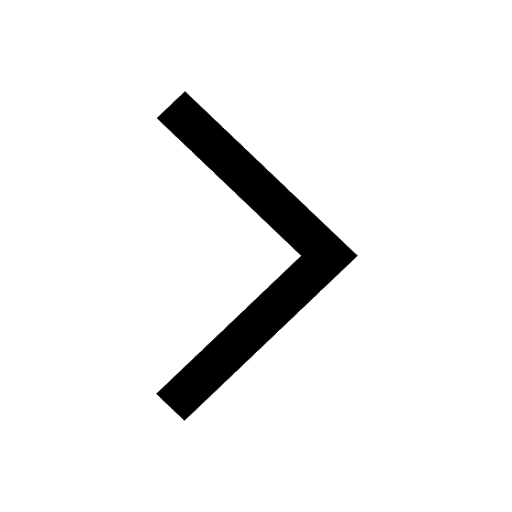
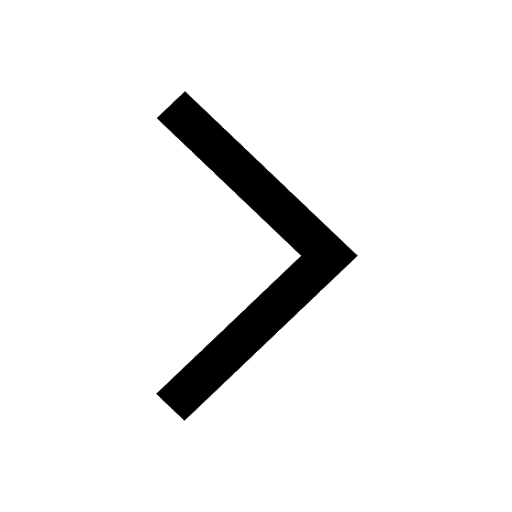
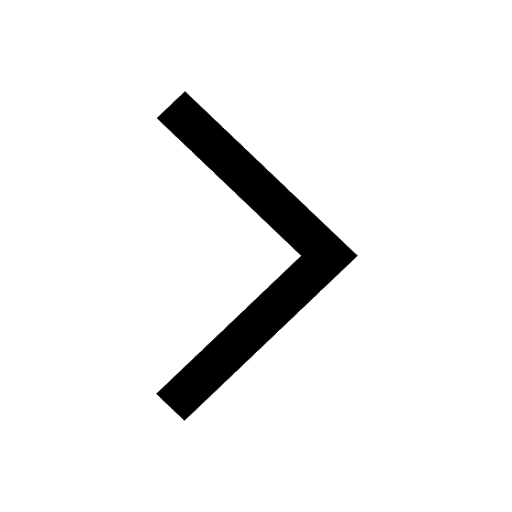