

You must have read the word ‘Significance’ often during research studies and other statistical procedures. Significance is crucial to reject or form a precise interpretation, based on the available data and values. This is more like a testing process to accept or reject a hypothesis in a study, and hence the name “Tests of Significance”. Significance testing is prominent in research fields, specifically in Surveys. Even subjects such as psychology, biology, medicine, and mathematics consider Statistical significance as a good contributor to real-time data.
Important Pointers to Know about the Tests of Significance
In the stance of reaching a particular conclusion, either the data has to be supported or rejected. This is possible through collecting the required data/information from the target population and deciding if the collected sample is enough or not. This method of assigning claims to pre-collected data after proper assessment and evaluations through some testing procedures are called Tests of Significance. This process is also helpful to identify if the sample is related to each other, as a result of random chance or not, and if yes, then to what extent.
A vast majority of the research surveys have some degree of error in the. Finding the percentage or level of error is the responsibility of the researcher or study head to in advance to the project submission. Regardless of the error, significance testing has its backbone as sample size. Only the collected data can speak volumes concerning if the information is sufficient or not.
How Useful are Tests of Significance in Statistics?
In judging the quality of research (accuracy, sample size, target population, data collection method, etc.), the experimenter must share if the setup is furnished with good research methodologies or by fluke.
And for this reason, the resulting value from tests of significance will judge the measurement’s quality and precision. This value can be both strong or weak.
In statistical studies, language usage plays a key role in influencing the level of significance tests. With poor language structure, the tests of significance will result in misinterpreted values. As per the recommendation of many statisticians and psychologists, only 5% chance error is allowed (meaning 95% of the research was properly undertaken).
The 5-Step Procedure to Significance Testing
Follow the 5 steps below to perform tests of significance for any subject-matter.
State your research hypothesis
Have a Null Hypothesis (H0)
Choose your error or probability level
Start the computation using accurate tests of significance
Interpret the obtained results
Defining the 2 Types of Errors
Statistical procedures are subjected to varying levels of errors. These errors can either be Type 1 or Type 2.
Type 1 Error: The hypothesis is absent but the researcher marks it as an existing relationship (present). The Research Hypothesis must be rejected and the Null Hypothesis has to be supported but the reverse is done.
Type 2 Error: Opposite of Type 1. The relationship would have existed but the researcher is unable to find it, hence giving interpretation is absent. The Research Hypothesis has to be accepted while rejecting the Null Hypothesis but the vice-versa happens.
2 Approaches to Statistical Testing
With a pre-collected data, the tests of significance are undertaken either as a one-tailed process or a two-tailed method. Also referred to as one-sided and two-sided tests, let’s get a brief idea about both:
One-Tailed/One-Sided: When it is possible to estimate the deviation in a parameter theoretically, only from 1 direction, using a benchmark assumption.
Two-Tailed/Two-Sided: When it is possible to evaluate a parameter’s deviation theoretically, from both the sides or directions, using any premise.
Only the research hypothesis can state if the former or latter type of statistical testing can be performed.
Conclusion
Tests of significance or significance testing involve the support or rejection of a sample data collected from the target population. This is a mandated procedure in psychology, medicine and majorly in statistics. The goal of significance testing is to find out if the results are obtained through proper researching or fluke work. Errors are possible during this process and it can be Type 1 (when a subject is absent but marked as Present) or Type 2 (when something is present but denoted as Absent). Only 5% chance error is possibly allowed for any research. When the data parameter’s deviation is estimated from 1 point, then it 1-sided statistical testing and if it’s in both the directions, then it two-tailed or two-sided respectively.
FAQs on Tests of Significance
1. Define p-Value Testing Subject to Research.
During a research study, the p-value represents the observed sample’s function and helps in finding the results using the statistical testing hypothesis. The value for a threshold has to be predetermined before performing the test.
2. How to Represent Type 1 and Type 2 Errors in a Research Study?
Use the symbol alpha (α) to denote Type 1 error and use beta (β) to represent Type 2 error for any research study. The researcher must also denote the degree of error for both the cases before starting the testing procedures.
3. What Does it Mean to Say by 0.05 Level of Significance?
By saying 0.05 is the level of significance, it means that there is a 5% chance of risk in interpreting that there is a presence of a subject when in reality any difference is absent.
4. How to Know if I Performed the Tests of Significance Correctly?
You can find out if you did the tests of significance correctly or not by doing the research once again and not by repeating the tests, as it might give false positives. If you get the same type of results again (in the 2nd research) then you have probably completed your significance testing correctly.
5. Is there any Disadvantage of Using Tests of Significance?
Yes. By using tests of significance you can only find if the data is collected through chance or not. But it does not support good results when the sample size is small. Moreover, the Null Hypothesis, for the majority cases, is false even though not.
6. What are the 2 Types of Hypothesis in a Sample?
The 2 types of Hypothesis in a sample is a Null Hypothesis which comes under the no difference category and the 2nd type is Alternative Hypothesis that possesses a significant difference.
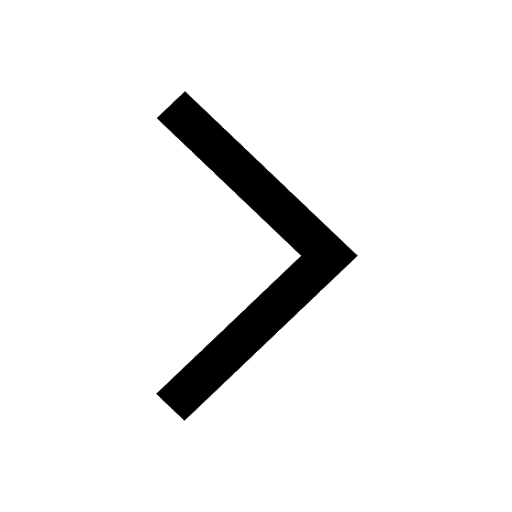
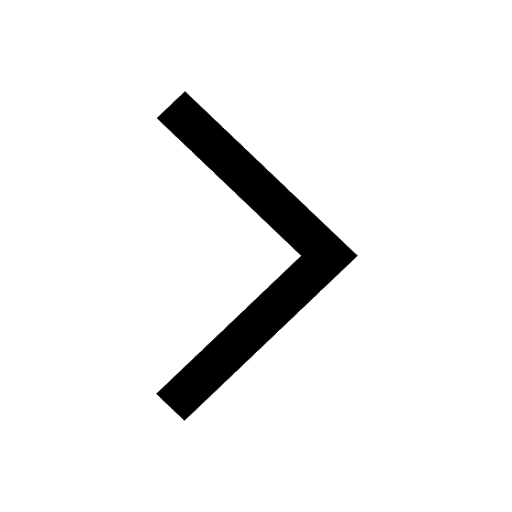
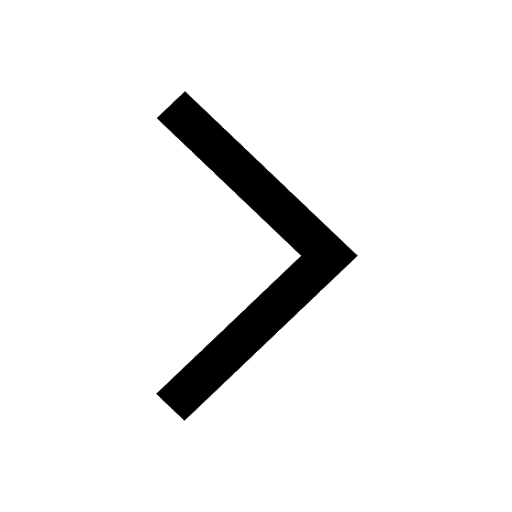
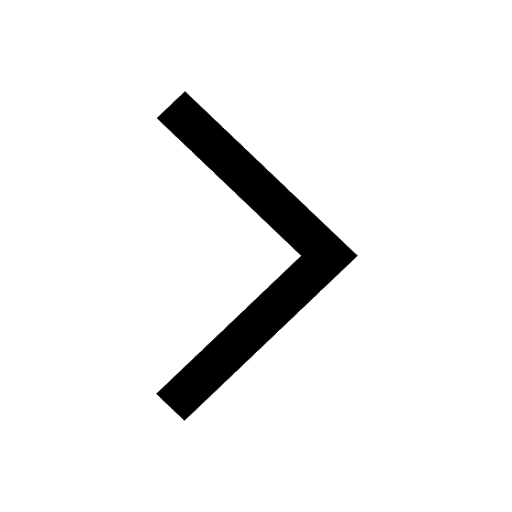
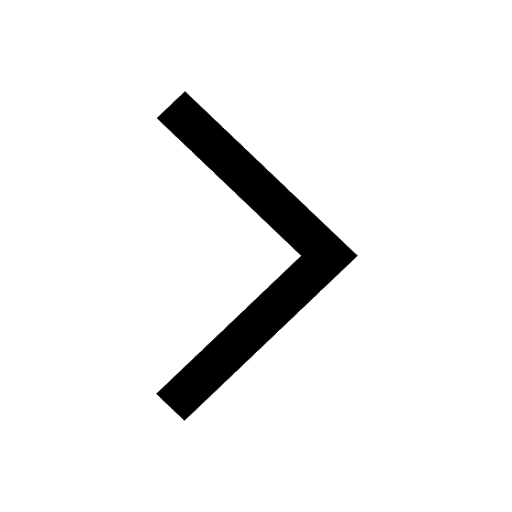
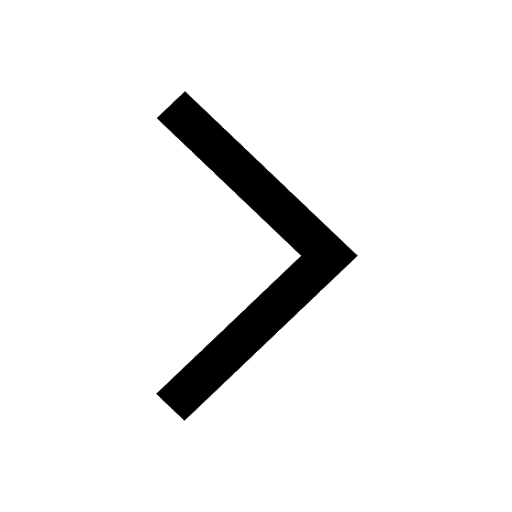
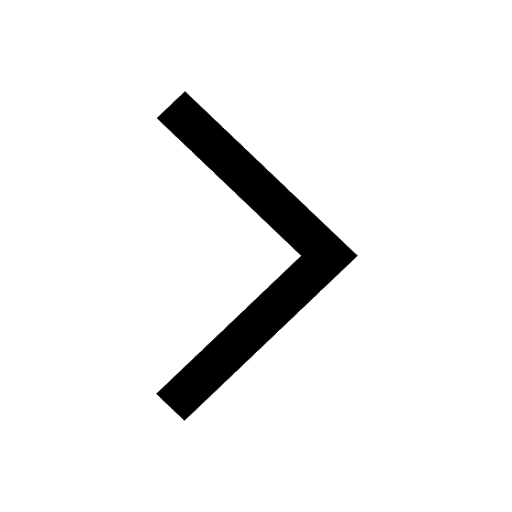
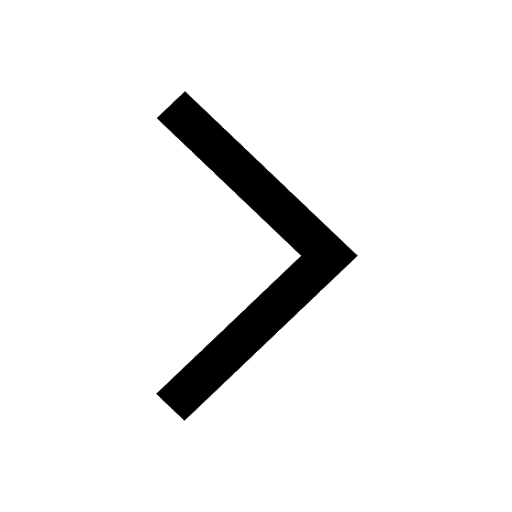
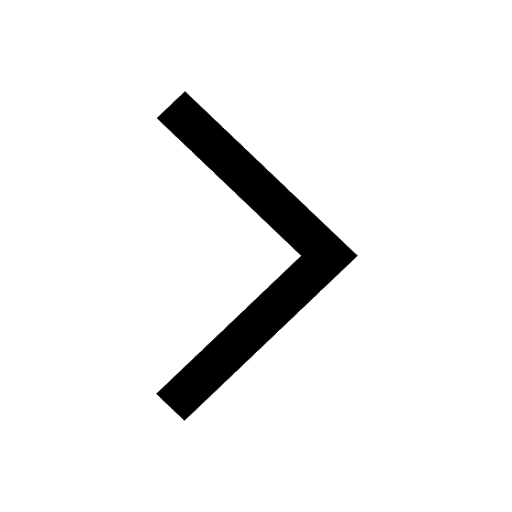
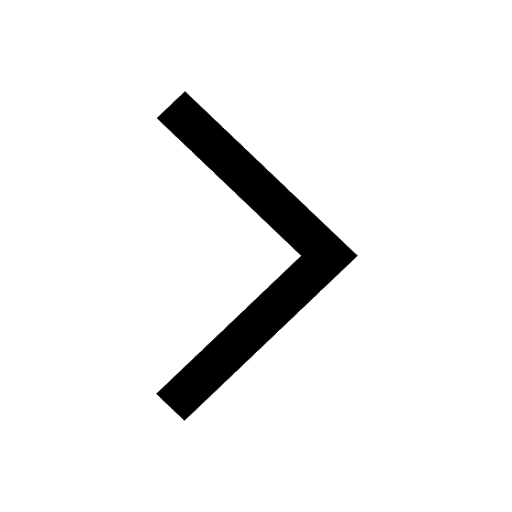
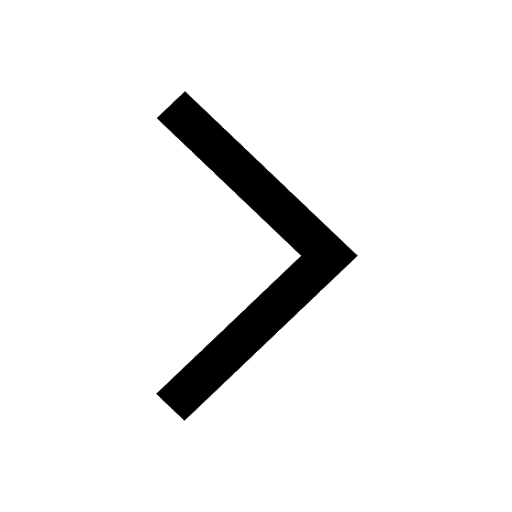
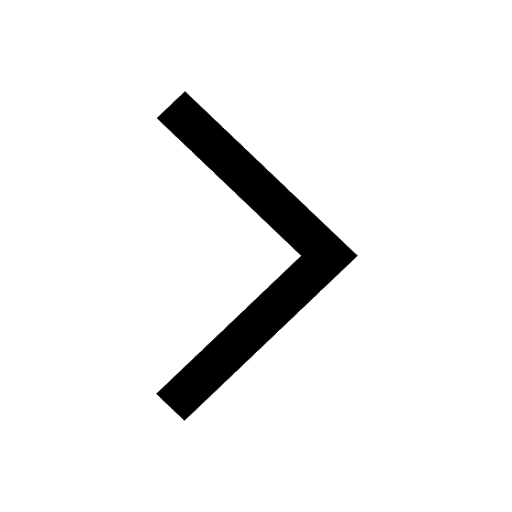
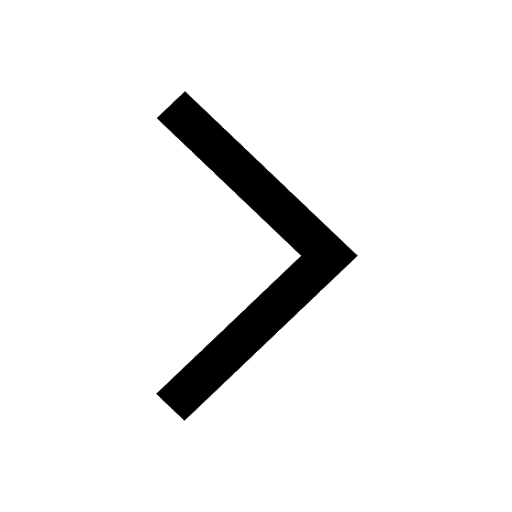
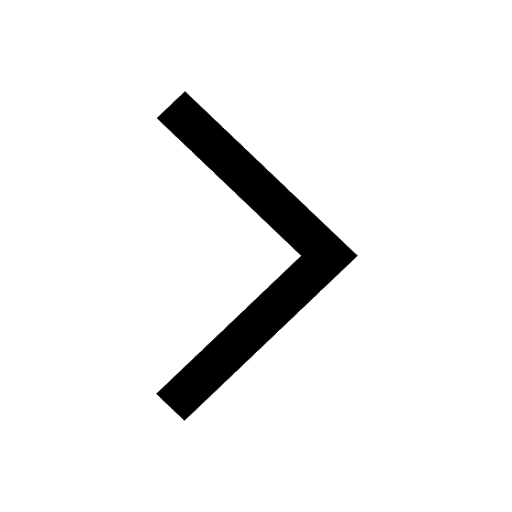
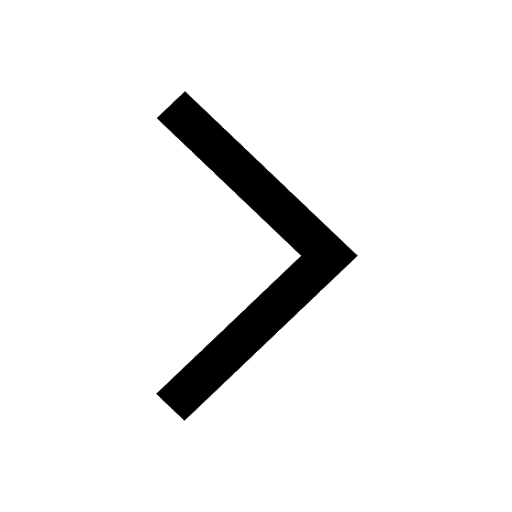
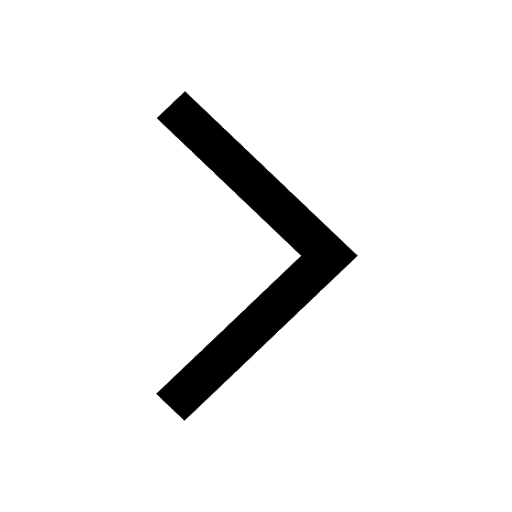
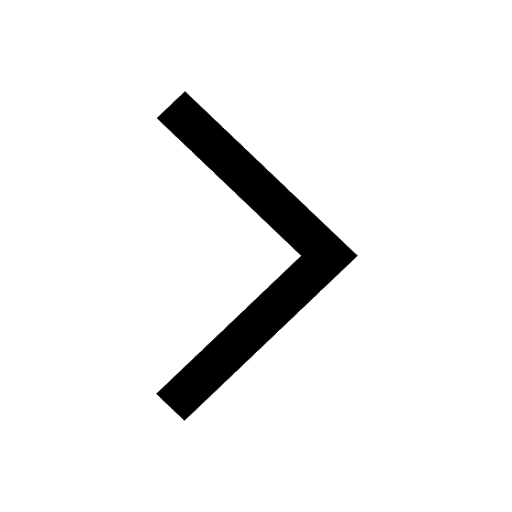
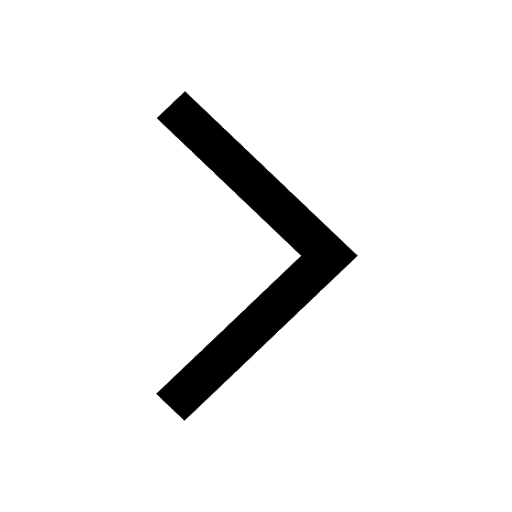