What are Correlation and Covariance: Introduction
FAQs on Difference Between Correlation and Covariance for JEE Main 2025
1. How to calculate covariance?
Covariance is calculated by taking the average of the products of the deviations of each variable from their respective means. The formula for covariance between two variables X and Y is: Cov(X, Y) = Σ((X - μX) * (Y - μY)) / N, where μX and μY are the means of X and Y, and N is the number of data points.
2. What is the unit of correlation?
Correlation is a unitless quantity.
3. What is the significance of correlation?
In correlation, the magnitude and direction of the correlation coefficient indicate the strength and direction of the relationship.
4. Which one is a scale-independent quantity among correlation and covariance?
Correlation is a scale-independent quantity, as it is not affected by the scale of the variable.
5. What does the correlation interpret?
Correlation provides a clear interpretation of the relationship. A correlation coefficient of (+1) indicates a perfect positive relationship, (-1) indicates a perfect relationship, and 0 suggests no linear relationship.
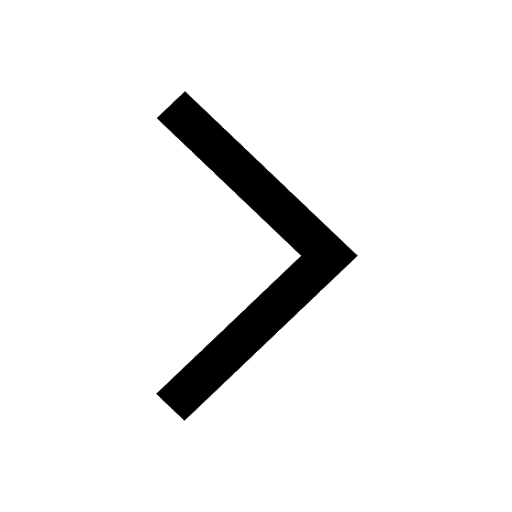
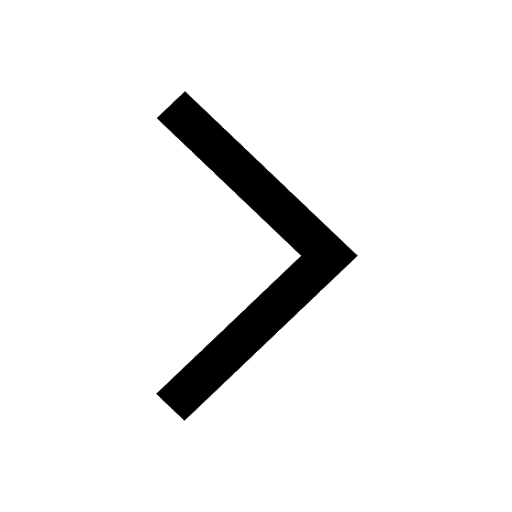
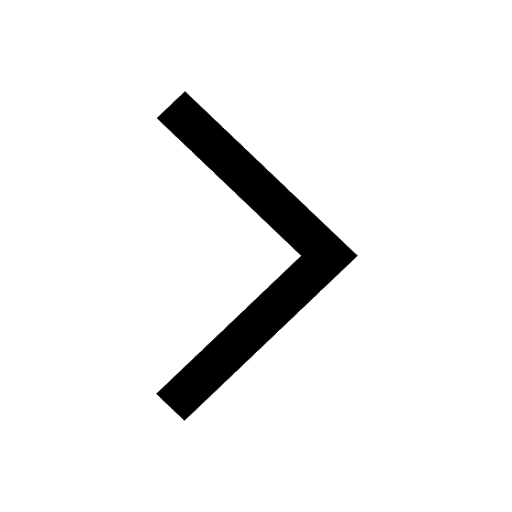
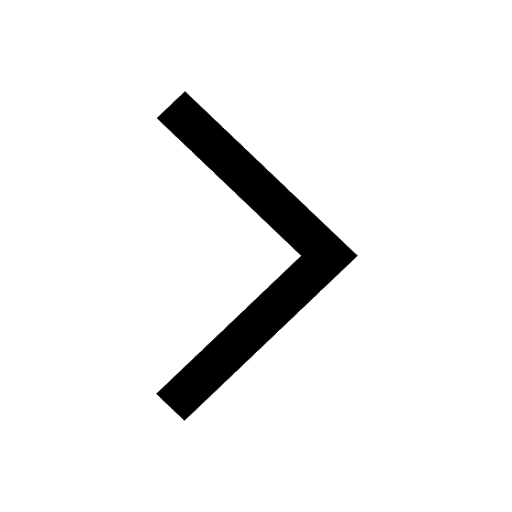
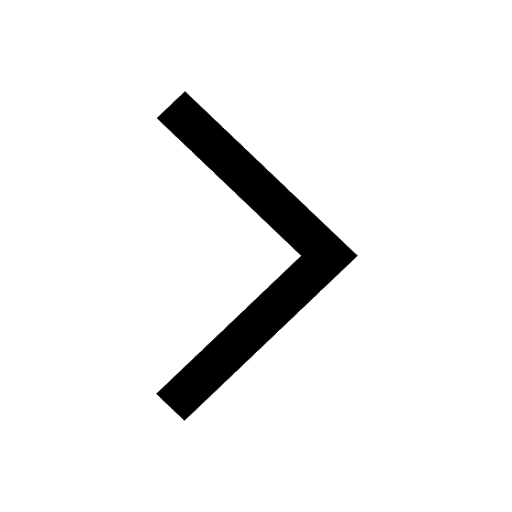
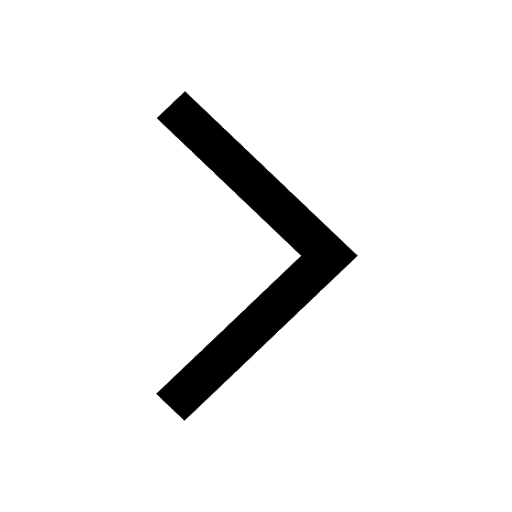
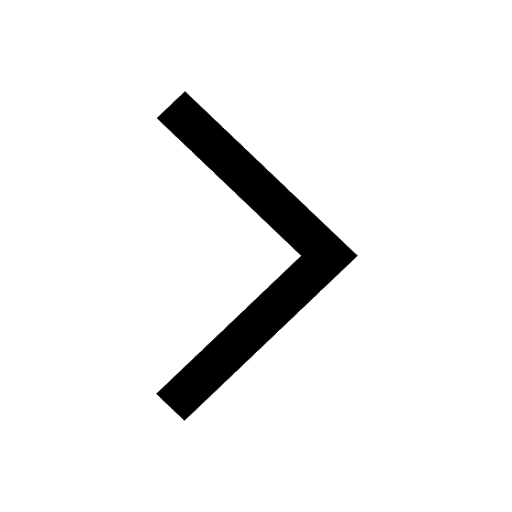
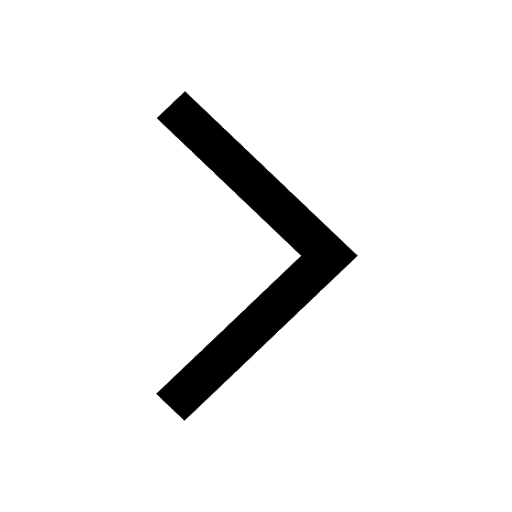
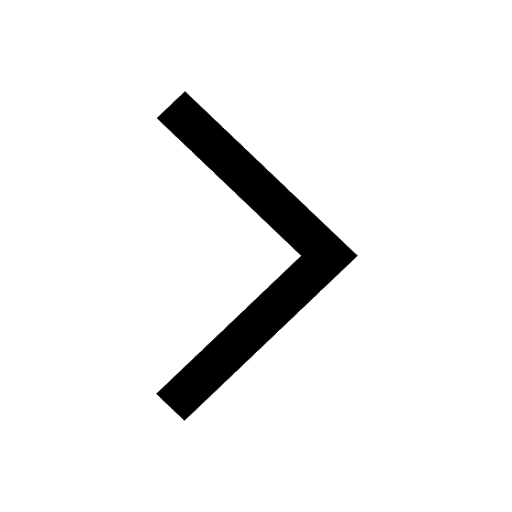
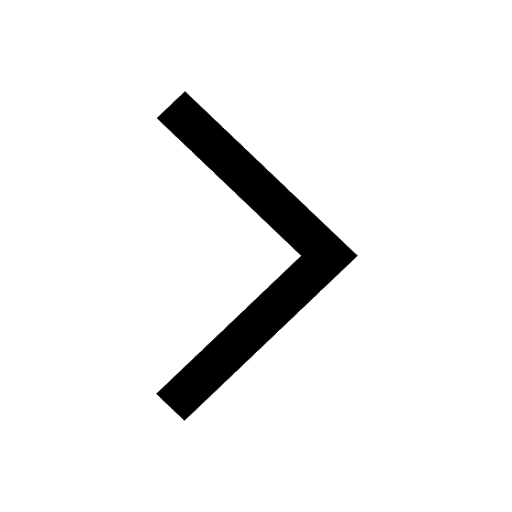
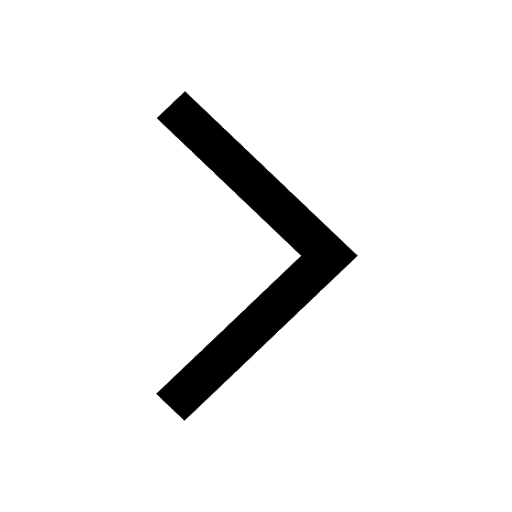
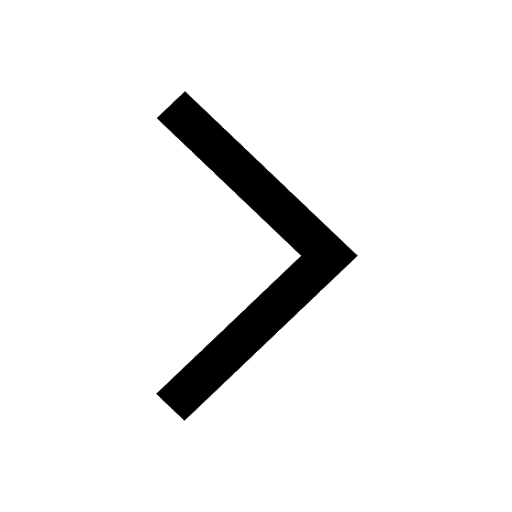